Colorado River Basin Actionable and Strategic Integrated Science and Technology (ASIST) Pilot Project Progress Toward an Information Management and Technology Plan
Links
- Document: Report (4.12 MB pdf) , HTML , XML
- Related Works:
- USGS Circular 1483 Rocky Mountain Region Science Exchange 2020—EarthMAP and the Colorado River Basin
- USGS Circular 1502 Colorado River Basin Actionable and Strategic Integrated Science and Technology Project—Science Strategy
- USGS Fact Sheet 2022-3010 Addressing Stakeholder Science Needs for Integrated Drought Science in the Colorado River Basin
- USGS Fact Sheet 2022-3016 Colorado River Basin Actionable and Strategic Integrated Science and Technology (ASIST)
- USGS Fact Sheet 2022-3051 U.S. Geological Survey Colorado River Basin Actionable and Strategic Integrated Science and Technology (ASIST)—Information Management Technology Plan
- USGS Open-File Report 2022-1040 Presented Abstracts from the U.S. Geological Survey 2020 Rocky Mountain Region Science Exchange (September 15–17, 2020)
- Download citation as: RIS | Dublin Core
Acknowledgments
The authors acknowledge the Colorado River Basin Science and Technology Collaboration Meeting participants from across the U.S. Geological Survey, the EarthMAP Program Management Team, Capacity Assessment Team, Use Case Development Team, Building EarthMAP Capacity Project, Office of the Associate Chief Information Officer, Core Science Systems, Community for Data Integration, science centers, and program coordinators for assistance in identifying the major challenges and recommended approaches to implement information management and technology to support integrated drought science in the Colorado River Basin. Thank you to William Andrews, Ken Bagstad, and Leslie Hsu for reviewing an early draft of this document. Special thanks to Kathryn Thomas and Nancy Sternberg for serving as peer reviewers of this document.
Executive Summary
The U.S. Geological Survey carries out a wide variety of multidisciplinary science projects through the Bureau’s regions, mission areas, programs, and science centers. However, this structure can limit interactions among individual scientists, segregate data holdings, and make it difficult to apply holistic, interdisciplinary science. In addition, technological advances in sensors, data storage and analysis, computing power, and networking have resulted in an exponential growth in the volume, variety, and complexity of data. To address some of these challenges, the U.S. Geological Survey initiated the Colorado River Basin Actionable and Strategic Integrated Science and Technology (ASIST) pilot project to facilitate interdisciplinary science in the drought-stricken basin and apply information management and technology (IMT) resources that can be used to deliver actionable science efficiently and effectively.
In fiscal year 2021, the Data Management and Advanced Technology subgroup of the ASIST pilot project worked toward developing an IMT plan that includes several advanced IMT solutions that are being implemented Bureau-wide by the Office of the Associate Chief Information Officer. This plan identifies applications, opportunities, and steps to leverage new and existing technologies, data, models, and knowledge to support integrated science projects across the Colorado River Basin. The subgroup also created an inventory of available IMT resources and their locations. The Colorado River Basin ASIST pilot project also developed a multiyear approach to build capacity for supporting integrated science projects in the Colorado River Basin, which provides an advanced IMT framework for expediting the production of interdisciplinary science related to the basin.
Introduction
The demand for data, scientific information, and predictions of how the Earth system will respond to natural and human-induced climate change has increased to the point where it is challenging to effectively and efficiently use the large number of U.S. Geological Survey (USGS) and other studies, data repositories, and collective knowledge available in the Colorado River Basin. Technological advances in sensors, data storage and analysis, computing power, and networking have resulted in an exponential growth in the volume, variety, and complexity of data. The dataset size, analysis complexity, number of data sources, and timely processing of data all contribute to the evolving demands on advancing technology for ingesting, processing, analyzing, and visualizing large, complex datasets (Gorton and Gracio, 2012). A comprehensive integration of techniques and technological advances can effectively harness the vast amount of data being generated and substantially accelerate scientific progress to address some of the world's most challenging problems (Critchlow and van Dam, 2017).
Implementation of technologies to meet the demands of today and the future demands for data-intensive science is a core part of the USGS mission (U.S. Geological Survey, 2021). In a 2017 workshop, USGS leaders described four overarching grand challenges to advance and integrate Earth science across USGS disciplines to address complex society problems (Jenni and others, 2017). Workshop participants identified Earth Monitoring, Analyses, and Projections (EarthMAP) as a long-term vision to integrate the portfolio of USGS science in a modular framework. In 2020, USGS began advancing the implementation of EarthMAP in the Colorado River Basin. The Colorado River Basin was selected because it is experiencing its worst drought since records began in the early 1900s (Lukas and Payton, 2020) and the ongoing threat of drought to the area’s biosecurity, natural resource conditions, and societal well-being. In response, the Colorado River Basin Actionable and Strategic Integrated Science and Technology (ASIST) pilot project was tasked with developing and implementing a basin-wide EarthMAP framework for scientific research and delivery of actionable science-based information to stakeholders. Similarly, in a 2021–7 informal strategy document, the USGS Office of the Associate Chief Information Officer (ACIO) outlined strategic goals to enable the USGS to meet 21st-century science needs (P. Exeter, U.S. Geological Survey, oral commun., 2022). Some of these needs include creating a Bureau-wide information management and technology (IMT) enterprise architecture, continuing to develop cloud technologies, expanding sensor networks, enabling new enterprise data-management capabilities, leveraging high performance computing (HPC) environments, and continuing to advance analytics and modeling tools and capabilities such as artificial intelligence (AI) and machine learning (ML). Data acquisition and management are important aspects for interpreting integrated Earth systems, and therefore, management and analysis of all pertinent data will be essential for the USGS to successfully accomplish its mission in the future. To this end, advanced IMT capabilities, which include the Internet of Things (IoT), HPC, cloud computing, AI, and ML, will be needed to manage Earth-system challenges (U.S. Geological Survey, 2021). These capabilities exist in the USGS, but their availability, interconnectivity, and management may not be apparent to all USGS scientists.
The Data Management and Advanced Technology subgroup of the ASIST pilot project is working toward a better understanding of the complexities associated with USGS data collected in the Colorado River Basin. The vast amount of past and ongoing USGS science in the Colorado River Basin is demonstrated with more than 840 publications, 575 data releases, and 330 project webpages that consider some aspect of science in the basin as of late 2021. To further complicate matters, these publications (and related models), datasets, and webpages are cataloged and available in multiple, unrelated locations, across numerous internal systems, data repositories, and local offices. This lack of interconnected availability limits the ability to efficiently access, synthesize, and interpret scientific resources. Drought-related data from the Colorado River Basin are diverse, including discrete, continuous, aerial, remote sensing, geophysical, geospatial, and other types of data in varied formats collected over multiple temporal and spatial scales. The Data Management and Advanced Technology subgroup is developing an IMT plan that includes several advanced IMT capabilities that are being implemented Bureau-wide by the ACIO. This document provides a review of the subgroups first-year activities with associated products and suggests a prospectus for continuing work. The document identifies applications, opportunities, and steps to leverage new and existing technologies, data, models, and knowledge to support integrated science projects across the Colorado River Basin.
Activity Highlights and Lessons Learned in Fiscal Year 2021
The Data Management and Advanced Technology subgroup researched technologies and data management practices that are being used in the USGS. This effort included informal meetings with USGS program and project leaders with discussions focused on innovative technology efforts that are improving science workflows. An early discussion with the ACIO provided context for the future direction of enterprise IMT. In addition, discussions with ACIO focused on cloud-based technologies available in the USGS Cloud Hosting Solutions (CHS) environment. Workers in the Core Science Systems (CSS) Science Analytics and Synthesis (SAS) group led discussions about data and model catalogs, analysis-ready data, and advanced research computing. Leaders at the USGS Earth Resources Observation and Science (EROS) Center provided insight into their state-of-the-art data-management system and how analysis-ready data have transformed the efficiency of delivering science results to stakeholders. In addition, examples of big data analytics and ML were presented to the subgroup and provided an applied approach to leveraging the vast amount of data collected by the USGS. In the end, a broad array of advanced technology and state-of-the-art science being utilized in the USGS were discussed and provided the foundation for moving the IMT plan forward.
Inventory of USGS Enterprise IMT Resources
The USGS gained access to many new data-intensive technologies and related resources in the past decade. However, for the typical USGS scientist, accessing such resources is not intuitive because they are spread across different locations, managed by different groups, and connected in different ways. To address these issues, the Data Management and Advanced Technology subgroup worked with the ACIO and CSS to review and document available enterprise IMT resources in the USGS with application to science in the Colorado River Basin. This inventory was initially compiled in a spreadsheet, and the concept eventually evolved into a more user-friendly diagram that illustrates how the different resources relate to one another (fig. 1). The resources are grouped into generalized IMT service categories, and the diagram identifies the “information technology ecosystem” where the resources reside (for example, cloud, hybrid, or on premises). In addition, the diagram places these resources into the USGS Science Data Lifecycle Model that describes the stages of data management and how data flow through a research project (Faundeen and others, 2013). Additional details about the IMT resources identified in figure 1 can be found in appendix 1.
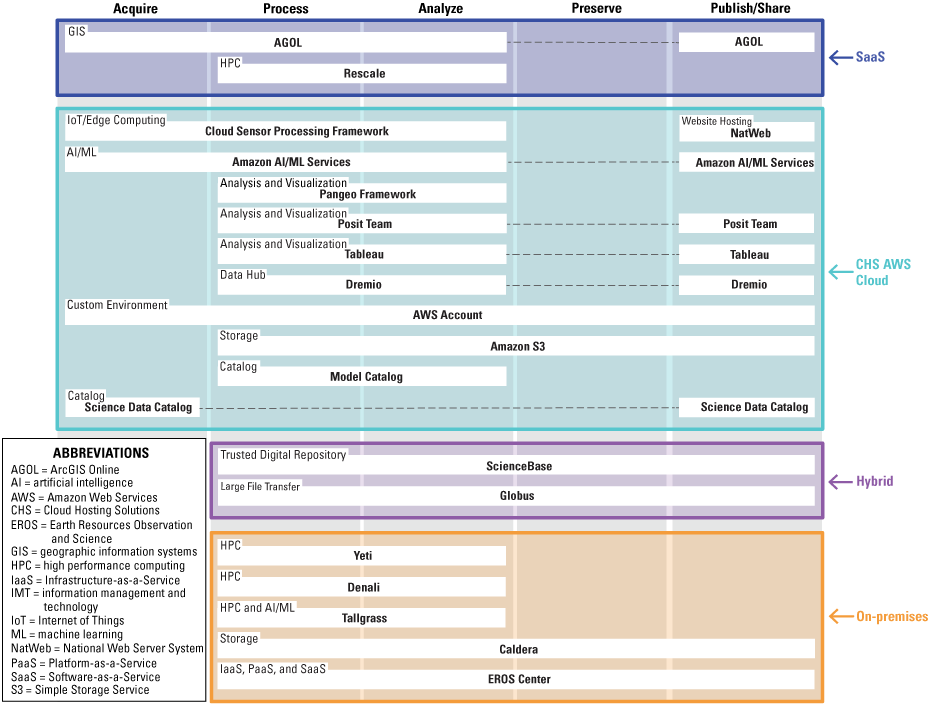
Diagram identifying science-centric enterprise information management and technology (IMT) resources for the Colorado River Basin Actionable and Strategic Integrated Science and Technology (ASIST) pilot project.
Exploration of Data Management and Principles
Exponential growth in the volume, variety, and complexity of data highlights the need to consider data-management strategies. As such, data-management plans are now required by USGS policy (Fundamental Science Practices Section 502.6: Scientific Data Management Office of Science Quality and Integrity; November 10, 2021, at https://www.usgs.gov/survey-manual/5026-fundamental-science-practices-scientific-data-management). The Fundamental Science Practices provide brief descriptions and resources for generating data-management plans, which include reference to a USGS Data Management Plan website (https://www.usgs.gov/data-management). Data management plans can be structured according to the USGS Science Data Lifecycle Model, which describes the stages of data management and how data flow through a research project from conception through preservation and sharing (Faundeen and others, 2013). The USGS Data Management Plan website provides USGS data-management plan templates and external tools to help establish a data-management plan. The Data Management and Advanced Technology subgroup recommends that a data-management plan be established for all data generated during the project.
USGS data-management practices are guided by the Findability, Accessibility, Interoperability, and Reusability (FAIR) principles that serve to guide data producers and publishers in maximizing the added value gained by formal, scholarly digital publishing of data and algorithms, tools, and workflows that were used to produce data (Wilkinson and others, 2016; Lightsom and others, 2022). A major challenge identified during this assessment is a lack of knowledge on how and where to find selected types of data and models. In addition, differences in the formats and locations of USGS data limit their interoperability and reusability. Specifically, the time, location, and purpose of the data collection affect whether they are stored in enterprise or local systems. The Data Management and Advanced Technology subgroup recommends that future discussions be held regarding how various data repositories supported by the Bureau can be more unified and governed by the FAIR principles, as outlined by Lightsom and others (2022).
The USGS provides data to stakeholders through several mechanisms, and the particular delivery portal used may depend on data type, science discipline, or region in which the data were collected. The Data Management and Advanced Technology subgroup had discussions with USGS managers and database developers regarding several portals to learn more about their uses. ScienceBase was developed by the USGS to enhance and expand information sharing and sound data-management practices. ScienceBase is a USGS trusted digital repository and provides access to aggregated information derived from many data and information domains. Key elements of ScienceBase include the following: (1) data cataloging and collaborative data-management platform, (2) central search and discovery application, (3) web services facilitating other applications, and (4) research community catalogs. The USGS Science Data Catalog integrates with the USGS Thesaurus, which allows users to browse for data using topics identified in the thesaurus. The Science Data Catalog is yet another location to find data and information produced by the USGS. The Science Data Catalog is a search and discovery tool that allows for metadata retrieval, visualization, download, and linkages to data repositories. The Science Data Catalog helps the USGS meet open data reporting required by the White House, offers a single source for the USGS to serve metadata to https://www.doi.gov/data and data.gov, and serves as a member node to the National Science Foundation-sponsored Data Observation Network for Earth, or DataONE, project.
Analysis-ready data are preprocessed in a format that facilitates further analysis. Analysis-ready data, therefore, can increase the speed of returning scientific results to stakeholders and decision makers. The USGS has recognized the importance of analysis-ready data, and substantial work was done to make the Landsat data archive analysis-ready. This allows users to quickly produce Landsat-based maps of land cover and change, and other derived geophysical and biophysical products (Dwyer and others, 2018). The Data Management and Advanced Technology subgroup recognizes the importance of analysis-ready data and recommends providing analysis-ready data in all cases to support integrated applications.
The increase in computing power has also led to an increase in data modeling. The USGS Model Catalog (https://data.usgs.gov/modelcatalog/) is led by the Core Science Systems Mission Area, Science Analytics and Synthesis Program, and Science Data Management Branch. The USGS Model Catalog includes models, tools, frameworks, and testbeds developed by the USGS or developed externally and used in USGS research. This catalog can increase awareness and facilitate model discovery, encourage reproducibility of science, and promote benefits of good model documentation. Compilation of the Model Catalog is ongoing, and at the time of writing, the Model Catalog focused on process-based scientific models rather than statistical, ML, or conceptual models. Entries for each model contain documentation recommended by the Open Modeling Foundation (https://www.openmodelingfoundation.org/standards/documentation/), including model version, related links, open-source license, and programming language.
Big data analytics and ML are applications that highlight the importance of data management, which were discussed by the subgroup and USGS scientists working in these fields. The artificial intelligence for environment and sustainability (ARIES) is an integrated modeling technology that is underlain by AI designed to help computers understand the data they are evaluating (found at https://aries.integratedmodelling.org/). The platform has been in development since 2007 by an international group of scientists. The system uses rules to guide the reasoning process and is highly dependent on semantics (consistent application of terminology) to give rigorous and logically consistent definitions. The Basin Characterization Model, an example of USGS big-data analytics, uses historical climate data, such as snow cover, snow water equivalent, evapotranspiration, soil moisture, and streamflow, to predict future water balances under differing climate and land-cover scenarios (Flint and others, 2013; Flint and others, 2021). The modeling outputs can be semantically described and used to inform other models, such as aquatic and terrestrial ecological models and reservoir management models, in AI applications like ARIES.
Advanced IMT and the Short-Term Colorado River Basin Projects
The ASIST pilot project selected 15 collaborative projects focused on drought to demonstrate their utility to help implement the EarthMAP concept for future science activities in the Colorado River Basin in 2020 (table 1). These projects had short-term deliverables that could be completed in fiscal year 2021 with support from the pilot project. The projects were further reviewed for their proposed and potential applications of advanced IMT to support and facilitate integrated science and deliver actionable information. For a select group of projects, the ACIO provided additional support and coordination to connect the projects with information technology (IT) specialists to discuss and provide recommendations for needed IT capabilities and expertise. Several projects proposed using advanced IMT resources, such as HPC, AI, ML, and analysis and visualization tools, or planned to use these resources in future phases of the projects. Full short-term project descriptions are available in the ASIST science strategy (Dahm and others, 2023).
Table 1.
List of Colorado River Basin integrated science pilot short-term use-case projects funded in fiscal year 2021 (Dahm and others, 2023).[PI, principal investigator; SBSC, Southwest Biological Science Center; AIFS, Advanced Integrated Fire Science; GECSC, Geosciences and Environmental Change Science Center; UTWSC, Utah Water Science Center; CAWSC, California Water Science Center; COWSC, Colorado Water Science Center; CERSC, Central Energy Resources Science Center; EMMA, Energy and Minerals Mission Area; SPARROW, SPAtially Referenced Regressions On Watershed Attributes; NVWSC, Nevada Water Science Center]
Science and Technology Collaboration Meetings
In the summer of 2021, the ASIST pilot project organized 12 collaboration meetings that brought together interested USGS staff with diverse perspectives to discuss science and technology challenges, existing capabilities, example applications, knowledge gaps, and actions. The goals of the meetings were to (1) provide opportunities to meet with others working on similar topics in different science centers or programs; (2) identify science and technology applications in the Colorado River Basin, stakeholder science and technology needs, existing USGS expertise and capabilities, and knowledge gaps; and (3) discuss science integration and technology implementation strategies to address knowledge gaps and actions to support implementation of new approaches for the USGS. In nearly all meetings, advanced IMT capabilities were mentioned, highlighting the importance of integrating technologies into future project workflows. The collaboration meetings that specifically focused on advanced IMT included the following topics:
Building EarthMAP Capacity and the Analysis-Ready Data/Dremio Working Group
The Building EarthMAP Capacity project was initiated in July 2020 to support EarthMAP projects and perform a rapid assessment of cyberinfrastructure and integrated modeling capabilities in the USGS. The Building EarthMAP Capacity team established eight working groups to evaluate and provide recommendations for the following thematic areas: analysis-ready data and data lake; analysis and visualization; communities of practice and partnerships; infrastructure as code and development and operations; advanced scientific computing; large data transfer, network, and storage capacity; Model Catalog; and computational testbeds. Identified gaps and recommendations were summarized in an internal USGS report released in November 2020.
In June 2021, members of the ASIST pilot project, ACIO, and CSS established a working group to build on the Building EarthMAP Capacity “analysis-ready data and data lake” recommendations and demonstrate workflows to connect science across disciplines and systems and improve the service of analysis-ready data for direct consumption. Specifically, this project’s participants plan to design, test, and implement a workflow for accessing scientific data held in USGS data repositories (for example, ScienceBase) using the CHS data hub service, Dremio, for analysis and synthesis, with submittal back to the repository for release. Dremio is a Structured Query Language, or SQL, lakehouse platform that leverages existing data repository structure and reduces data complexities and accelerates querying of tabular data to deliver fast and efficient results to end users. A project product will be a data governance strategy to support this data workflow scenario.
Lessons Learned
The USGS initiated the ASIST pilot project to accelerate interdisciplinary science carried out in the Colorado River Basin and apply advanced IMT solutions. The pilot project team was tasked with developing integrated predictive capabilities for decision making through advanced technology. Historically, the USGS developed data workflows within individual disciplines; however, 21st-century predictive science requires tools and technologies that span multiple disciplines and support science projects led by staff across the entire Bureau. The work during the first year of the ASIST pilot project focused on identifying IMT resources; documenting monitoring, analysis, and prediction capacities; and hosting scientific collaboration meetings. The lessons learned through those first-year activities helped focus and define future efforts. The lessons learned from the Data Management and Advanced Technology subgroup include the following:
-
• There is no easy way to quickly understand the breadth of existing USGS IMT and data capabilities—such understanding requires data calls because published data libraries are scattered, internal data libraries are not cataloged, and real-time data capabilities are challenged.
-
• There is no easy way to compile existing USGS staff capabilities.
-
• There is no easy way to understand existing (ongoing and nonactive) USGS research projects in a particular geographic setting that spans multiple USGS regions and science centers.
-
• It is important to take into account the following structures and systems:
-
• Organizational and communication challenges that hinder needed implementation of advanced IMT resources.
ASIST Data Management and Advanced Technology Working Group Action Plan for Fiscal Years 2022–26
Building upon fiscal year 2021 activities and lessons learned, the Data Management and Advanced Technology subgroup of the ASIST pilot project outlined a multiyear approach to build capacity for supporting integrated science projects in the Colorado River Basin from 2022 to 2026 (table 2). Future activities of the subgroup will focus on building support to implement IMT at multiple scales with consistent interoperable solutions for the community of scientists working on integrated science efforts in the basin. Based on the assessment performed in 2021, short-term activities will focus on initiating the implementation of advanced IMT solutions to improve collaborations and connections among scientists, stakeholders, and partners who have a need for integrated science solutions. Long-term activities will further develop and integrate technologies and provide documentation and support for leveraging the advanced IMT solutions. Such an advanced IMT framework is needed for science in the Colorado River Basin through which USGS projects, programs, and platforms can interact and reside independently to provide maximum flexibility and autonomy for individual projects while streamlining connections and improving collaboration. Implementation of IMT resources in a usable framework for the Colorado River Basin is expected to reduce the cost, improve efficiency, and expedite delivery of science.
Table 2.
Multiyear approach to build capacity for supporting integrated science projects in the Colorado River Basin from 2022 to 2026.[FY22, fiscal year 2022; IMT, information management and technology; SQL, Structured Query Language; USGS, U.S. Geological Survey; IoT, Internet of Things; AI/ML, artificial intelligence and machine learning; HPC, high performance computing]
References Cited
Dahm, K.G., Hawbaker, T.J., Frus, R.J., Monroe, A.P., Bradford, J.B., Andrews, W.J., Torregrosa, A., Anderson, E.D., Dean, D.J., and Qi, S.L., 2023, Colorado River Basin Actionable and Strategic Integrated Science and Technology Project—Science strategy: U.S. Geological Survey Circular 1502, 57 p., https://doi.org/10.3133/cir1502.
Dwyer, J.L., Roy, D.P., Sauer, B., Jenkerson, C.B., Zhang, H.K., and Lymburner, L., 2018, Analysis ready data—Enabling analysis of the Landsat archive: Remote Sensing, v. 10, no. 9, article 1363, 19 p., accessed October 15, 2021, at https://doi.org/10.3390/rs10091363.
Faundeen, J.L., Burley, T.E., Carlino, J.A., Govoni, D.L., Henkel, H.S., Holl, S.L., Hutchison, V.B., Martín, E., Montgomery, E.T., Ladino, C.C., Tessler, S., and Zolly, L.S., 2013, The U.S. Geological Survey science data lifecycle model: U.S. Geological Survey Open-File Report 2013–1265, 4 p., accessed October 15, 2021, at https://doi.org/10.3133/ofr20131265.
Flint, L.E., Flint, A.L., Thorne, J.H., and Boynton, R., 2013, Fine-scale hydrologic modeling for regional landscape applications—the California Basin Characterization Model development and performance: Ecological Processes, v. 2, article 25, 21 p., accessed November 10, 2021, at https://doi.org/10.1186/2192-1709-2-25.
Flint, L.E., Flint, A.L., and Stern, M.A., 2021, The Basin Characterization Model—A regional water balance software package: U.S. Geological Survey Techniques and Methods 6–H1, 85 p., accessed November 10, 2021, at https://doi.org/10.3133/tm6H1.
Jenni, K.E., Goldhaber, M.B., Betancourt, J.L., Baron, J.S., Bristol, R.S., Cantrill, M., Exter, P.E., Focazio, M.J., Haines, J.W., Hay, L.E., Hsu, L., Labson, V.F., Lafferty, K.D., Ludwig, K.A., Milly, P.C.D., Morelli, T.L., Morman, S.A., Nassar, N.T., Newman, T.R., Ostroff, A.C., Read, J.S., Reed, S.C., Shapiro, C.D., Smith, R.A., Sanford, W.E., Sohl, T.L., Stets, E.G., Terando, A.J., Tillitt, D.E., Tischler, M.A., Toccalino, P.L., Wald, D.J., Waldrop, M.P., Wein, A., Weltzin, J.F., and Zimmerman, C.E., 2017, Grand challenges for integrated USGS science—A workshop report: U.S. Geological Survey Open-File Report 2017–1076, 94 p., accessed November 10, 2021, at https://doi.org/10.3133/ofr20171076.
Lightsom, F.L., Hutchison, V.B., Bishop, B., Debrewer, L.M., Govoni, D.L., Latysh, N., and Stall, S., 2022, Opportunities to improve alignment with the FAIR Principles for U.S. Geological Survey data: U.S. Geological Survey Open-File Report 2022–1043, 23 p., accessed October 10, 2022, at https://doi.org/10.3133/ofr20221043.
Lukas, J., and Payton, E., eds., 2020, Colorado River Basin climate and hydrology—State of the Science: Boulder, Colo., Western Water Assessment, University of Colorado Boulder, 519 p., accessed March 20, 2021, at https://doi.org/10.25810/3hcv-w477.
U.S. Geological Survey, 2021, U.S. Geological Survey 21st-century science strategy 2020–2030: U.S. Geological Survey Circular 1476, 20 p., accessed November 20, 2021, at https://doi.org/10.3133/cir1476.
Wilkinson, M.D., Dumontier, M., Aalbersberg, I.J., Appleton, G., Axton, M., Baak, A., Blomberg, N., Boiten, J.-W., da Silva Santos, L.B., Bourne, P.E., Bouwman, J., Brookes, A.J., Clark, T., Crosas, M., Dillo, I., Dumon, O., Edmunds, S., Evelo, C.T., Finkers, R., Gonzalez-Beltran, A., Gray, A.J.G., Groth, P., Goble, C., Grethe, J.S., Heringa, J., ’t Hoen, P.A.C., Hooft, R., Kuhn, T., Kok, R., Kok, J., Lusher, S.J., Martone, M.E., Mons, A., Packer, A.L., Persson, B., Rocca-Serra, P., Roos, M., van Schaik, R., Sansone, S.-A., Schultes, E., Sengstag, T., Slater, T., Strawn, G., Swertz, M.A., Thompson, M., van der Lei, J., van Mulligen, E., Velterop, J., Waagmeester, A., Wittenburg, P., Wolstencroft, K., Zhao, J., and Mons, B., 2016, The FAIR guiding principles for scientific data management and stewardship: Scientific Data, v. 3, article 160018, 9 p., accessed October 10, 2021, at https://doi.org/10.1038/sdata.2016.18.
Appendix 1. Advanced IMT Resources
Table 1.1.
Summary of advanced information management and technology resources.[AI/ML, artificial intelligence and machine learning; CHS, Cloud Hosting Solutions; AWS, Amazon Web Services; ACIO, Associate Chief Information Officer; USGS, U.S. Geological Survey; CSS, Core Science Systems; SAS, Science Analytics and Synthesis; ARC, Advanced Research Computing; HPC, high performance computing; GPU, general processing unit; CUDA, compute unified device architecture; SDM, science data management; SDC, Science Data Catalog; OMB, Office of Management and Budget; SQL, Structured Query Language; NoSQL, nonrelational databases; GIS, geographic information systems; AGOL, ArcGIS Online; Esri, Environmental Systems Research Institute; CPU, central processing unit; RAM, random access memory; TB, terabyte; 3D, three dimensional; EROS, Earth Resources Observation and Science; IaaS, Infrastructure-as-a-Service; PaaS, Platform-as-a-Service; SaaS, Software-as-a-Service; IT, information technology; IoT, Internet of Things; ~, about; PB, petabyte; S3, simple storage service; NatWeb, National Web Server System; LAMP, Linux Apache MySQL PHP]
Abbreviations
ACIO
Associate Chief Information Officer
AGOL
ArcGIS Online
AI
artificial intelligence
ARC
Advanced Research Computing
ARIES
artificial intelligence for environment and sustainability
ASIST
Actionable and Strategic Integrated Science and Technology
AWS
Amazon Web Services
CDI
Community for Data Integration
CHS
Cloud Hosting Solutions
CPU
central processing unit
CSS
Core Science Systems
CUDA
compute unified device architecture
DOD
Department of Defense
EarthMAP
Earth Monitoring, Analyses, and Projections
EPA
U.S. Environmental Protection Agency
EROS
Earth Resources Observation and Science
Esri
Environmental Systems Research Institute
GIS
geographic information systems
GPU
general processing unit
HPC
high performance computing
HTC
high throughput computing
IaaS
Infrastructure-as-a-Service
IMT
information management and technology
IoT
Internet of Things
IT
information technology
ML
machine learning
NatWeb
National Web Server System
OMB
Office of Management and Budget
PaaS
Platform-as-a-Service
PB
petabyte
ppm
parts per million
RAM
random access memory
SaaS
Software-as-a-service
SAS
Science Analytics and Synthesis
SDC
Science Data Catalog
SDM
science data management
SQL
structured query language
TB
terabyte
USGS
U.S. Geological Survey
Disclaimers
Any use of trade, firm, or product names is for descriptive purposes only and does not imply endorsement by the U.S. Government.
Any use of trade, firm, or product names is for descriptive purposes only and does not imply endorsement by the U.S. Government.
Although this information product, for the most part, is in the public domain, it also may contain copyrighted materials as noted in the text. Permission to reproduce copyrighted items must be secured from the copyright owner.
Suggested Citation
Anderson, E.D., Erxleben, J.R., Qi, S.L., Monroe, A.P., and Dahm, K.G., 2023, Colorado River Basin Actionable and Strategic Integrated Science and Technology (ASIST) pilot project progress toward an information management and technology plan: U.S. Geological Survey Circular 1510, 11 p., https://doi.org/10.3133/cir1510.
ISSN: 2330-5703 (online)
Study Area
Publication type | Report |
---|---|
Publication Subtype | USGS Numbered Series |
Title | Colorado River Basin Actionable and Strategic Integrated Science and Technology (ASIST) pilot project progress toward an information management and technology plan |
Series title | Circular |
Series number | 1510 |
DOI | 10.3133/cir1510 |
Year Published | 2023 |
Language | English |
Publisher | U.S. Geological Survey |
Publisher location | Reston VA |
Contributing office(s) | Rocky Mountain Region Director’s Office |
Description | viii, 10 p. |
Country | United States |
Other Geospatial | Colorado River basin |
Online Only (Y/N) | Y |
Google Analytic Metrics | Metrics page |