Status of Greater Sage-Grouse in the Bi-State Distinct Population Segment—An Evaluation of Population Trends, Habitat Selection, and Efficacy of Conservation Actions
Links
- Document: Report (9 MB pdf) , HTML , XML
- Data Releases:
- USGS Data Release - Rasters and tables for selection and survival of greater sage-grouse nests and broods in the Bi-State Distinct Population Segment of California and Nevada
- USGS Data Release - Greater sage-grouse habitat selection, survival, abundance, and space-use in the Bi-State Distinct Population Segment of California and Nevada
- Download citation as: RIS | Dublin Core
Acknowledgments
We coordinated this project in close consultation with the U.S. Fish and Wildlife Service (USFWS), the Nevada Department of Wildlife (NDOW), the California Department of Fish and Wildlife (CDFW), the Bureau of Land Management (BLM), the U.S. Forest Service (USFS), and the Bi-State Technical Advisory Committee. We would also like to acknowledge the Bi-State Executive Oversight Committee, Bi-State Traditional Natural Resources Committee, and Bi-State Local Area Working Group. We thank M. Ricca (U.S. Geological Survey [USGS]) and J. Small (NDOW) for helpful comments in reviewing the report in its entirety. We appreciate the efforts of J. Atkinson (USGS) for assisting with report preparation; and K. Calvert (USGS) and K. Engelking (USGS) for editing, formatting, and final production of this report. We thank S. Dettenmaier (USGS), K. McGowan, A. Kosic, P. Winters, P. Fuselier, D. Dekelaita, K. Krause (BLM), M. Nelson, K. Schlick, N. Sill (USFS), S. Gardner (CDFW), K. Steele (Nevada State Sagebrush Ecosystem Technical Team), J. Barrett (USFWS), and R. Tucker (Los Angeles Department of Water and Power) for their input throughout the study. We thank the NDOW and CDFW for granting permits to the USGS for marking and tracking sage-grouse. This project could not have been completed without the financial support of the BLM, USGS Ecosystems Mission Area, USFWS, USFS, NDOW, and CDFW.
Preface
This study was completed to provide timely scientific information regarding greater sage-grouse population trends, habitat selection, and the efficacy of previous conservation actions implemented to benefit the Bi-State Distinct Population Segment (DPS). Specifically, we provide these analyses to inform the current (2024) status review and pending listing decision for the DPS being undertaken by the U.S. Fish and Wildlife Service. These findings provide updated, detailed, and comprehensive information regarding the status of a geographically isolated and genetically distinct population of a species of high conservation concern and their habitat. Importantly, this report also provides information on the efficacy of previously implemented conservation actions targeting the Bi-State DPS in a framework that is transferable throughout the species’ range.
Executive Summary
As a sagebrush obligate and indicator species for increasingly threatened sagebrush ecosystems, greater sage-grouse (Centrocercus urophasianus; hereinafter sage-grouse) have become central to land management policy throughout the western United States. The integral role of sage-grouse in guiding land management is exemplified by the conservation and management of populations inhabiting the southwestern extent of the species’ range, along the border of California and Nevada. Sage-grouse in this region are recognized as the Bi-State Distinct Population Segment (DPS) based on both geographic isolation and genetic distinctiveness. As such, the U.S. Fish and Wildlife Service (USFWS) has separately evaluated the Bi-State DPS for listing under the Endangered Species Act (ESA) since first being petitioned in 2002. The USFWS determined the Bi-State DPS to be “warranted but precluded for listing” in a 2010 decision that highlighted multiple threats and prompted collaborative efforts to implement science-based adaptive management actions. The USFWS determined that the Bi-State DPS did not warrant listing under the ESA in 2015 and again in 2020 due to the demonstrated commitment to the conservation of the Bi-State DPS and the execution of a suite of conservation actions. However, these decisions were vacated by the U.S. District Court in 2018 and 2022, respectively. Hence, in April 2023 the USFWS reopened the comment period on the 2013 proposed rules to list the DPS as threatened and to designate critical habitat under the ESA. To provide timely science to inform ongoing conservation efforts within the Bi-State, the current (2024) USFWS status review, and pending listing decision, we report results of three primary study objectives:
-
1. Map Sage-Grouse Habitat Selection and Demographic Performance within the Bi-State Distinct Population Segment
Purpose: Understanding the spatial relationship between habitat selection and demographic performance is important to inform management decisions because habitat selection is not always adaptive. Evaluating the spatial congruence of resources associated with both habitat selection and demographic performance can highlight areas (1) supporting both high habitat selection and survival to prioritize for conservation and (2) areas of misalignment between selection and survival to target for restoration or habitat improvement. We provide a quantitative approach to differentiate productive habitats supporting high selection and survival from areas of maladaptive selection where selection and survival are misaligned at large spatial scales. We implement this approach and map combined selection-survival indices across the Bi-State region. These combined selection-survival indices and associated maps provide a more complete understanding of habitat relationships and facilitate spatially explicit prioritization of areas for conservation or restoration.
Methods: We used movement and demographic data to simultaneously evaluate habitat selection by sage-grouse across multiple seasons, and measures of survival during key reproductive life stages (nesting and brood-rearing) to identify priority habitat by linking resource selection to demographic performance. We calculated and mapped composite selection and survival indices across the Bi-State region to differentiate productive habitat that supported high selection and survival compared to areas of maladaptive selection where selection and survival were misaligned.
Results: Our findings indicate competing resource demands varied across time, with predation risk and protective cover being dominant drivers of habitat selection during nesting and during early brood-rearing periods when chicks were smaller and flightless, whereas access to forage resources was more important during late brood-rearing when those resources were more limited. Within the Bi-State region, 5.3 percent (844 square kilometers [km2]) of the landscape was subject to high selection, 15.2 percent (2,419 km2) to moderate selection, 41.2 percent (6,536 km2) to low selection, and 38.3 percent (6,325 km2) was considered non-habitat for sage-grouse. Of the area classified as habitat during reproductive life stages, 17.2 percent (1,684 km2) of the Bi-State region was classified as high survival, 19.9 percent (1,947 km2) as moderate survival, 14.8 percent (1,445 km2) as low survival, and 48.1 percent (4,621 km2) as very low survival. We also created combined selection-survival maps across the study area by overlaying categorized selection and survival maps from reproductive life stages. Productive habitat (high or moderate selection paired with high or moderate survival) accounted for 14.1 percent (1,375 km2) of the Bi-State region, whereas areas with potentially maladaptive habitat selection (high or moderate selection paired with low or extremely low survival) represented 19.3 percent (1,888 km2).
-
2. Evaluate Population Abundance, Trends, and Distribution of Sage-Grouse within the Bi-State Distinct Population Segment
Purpose: Trends and status of wildlife populations are typically evaluated using either changes in distribution or abundance, independently, under the assumption that these measures are correlated. However, distribution and abundance, particularly in a metapopulation, can become disconnected if subpopulations over- or underperform relative to overall population trends. If this disconnect occurs, overall population trends can remain stable even though distribution declines. Therefore, focusing only on total abundance can mask important losses in distribution that could affect metapopulation dynamics and lead to long-term instability.
Methods: Using lek counts, demographic data, and information on space use from marked individuals, we evaluated changes in population abundance for greater sage-grouse within the Bi-State DPS from 1969 to 2019 using a state-space model and from 2001 to 2021 using an integrated population model. We used the two different modeling approaches to align with and make best use of available data sources. We evaluated changes in total population abundance from nadir to nadir (that is, between population minimums spanning different population cycles) over six time periods: long (1969–2019), medium/long (1978–2019), medium (1983–2019), medium/short (1995–2019), short (2002–19), and recent term (2008–19). We also evaluated changes in distribution from 1995 to 2019.
Results: The Bi-State DPS demonstrated evidence of declines of approximately (~) 1.2–2.5 percent annually over the long-term (=0.979, 95-percent CRI: 0.968, 0.987), medium/long-term (=0.977, 95-percent CRI: 0.963, 0.987), medium-term (=0.985, 95-percent CRI: 0.969, 0.995), short/medium-term (=0.987, 95-percent CRI: 0.970, 0.999), short-term (=0.975, 95-percent CRI: 0.963, 0.985), and recent-term (=0.988, 95-percent CRI: 0.973, 1.001) based on the state-space model. Estimated trends over the short-term (=0.980, 95-percent CRI: 0.971, 0.990) and recent-term (=0.985, 95-percent CRI: 0.973, 0.997) from the integrated population model were similar to estimates from the state-space model, although there were slight differences in the estimated nadir years and credible intervals were smaller due to the inclusion of demographic data. Since 1995, the distribution of occupied sage-grouse habitat in the Bi-State DPS shifted among subpopulations, with gains in three subpopulations insufficient to offset losses in six subpopulations. As a result, there was a net loss in occupied distribution across the Bi-State DPS, with the amount of occupied area contracting by ~156 km2.
-
3. Evaluate Efficacy of Ongoing Conservation Actions Targeting Sage-Grouse within the Bi-State Distinct Population Segment
Purpose: Successful conservation efforts require effective monitoring and analytical frameworks that incorporate robust assessments of conservation actions in relation to target species’ population viability. However, the complex and dynamic nature of interacting ecosystem processes and practical limitations in assessing population dynamics pose challenges to quantifying conservation efficacy.
Methods: We used a newly developed web-based repository of conservation actions within the Bi-State DPS and other restoration databases to examine whether management actions translated to improvements in sage-grouse population performance. We used hierarchical population model estimates of interannual abundance () of sage-grouse as inputs for analysis of change in abundance relative to a suite of conservation actions that we structured using a progressive change before-after-control-impact paired-series (PC BACIPS) study design. We incorporated data from 57 leks monitored between 2003 and 2021 and 85 individual conservation actions implemented between 2012 and 2019. The range of years chosen for both datasets resulted in a minimum of 10 years of before data and a maximum of 10 years of after data. Inclusion of count data through 2021 allowed sufficient post-treatment years to assess conservation actions implemented during 2019.
Results: Overall, there were average annual increases of 4.4 percent in population abundance across the study area, relative to controls, and a 37.4 percent cumulative increase since 2012, with evidence of conservation effectiveness supported by BACIPS results (for example, more improvement in areas with conservation actions relative to areas without actions). Population gains varied relative to both the type of conservation effort and the number of lag years since its implementation. For example, management actions including sagebrush restoration and road closures resulted in improved growth rates each year after implementation, and hand cutting and mechanically removing conifers, including pinyon and juniper trees, had immediate positive effects on population growth.
Background
Greater sage-grouse (Centrocercus urophasianus; hereinafter sage-grouse) is a sagebrush obligate species and widely considered an indicator species for sagebrush ecosystems and other sagebrush-dependent species (Hanser and Knick, 2011; Prochazka and others, 2023). Sagebrush ecosystems are threatened by a wide range of disturbances and anthropogenic factors, including climate change, severe drought, altered wildfire regimes, expansion of invasive species, and anthropogenic development. Collectively, these threats have led to reduced ecological integrity and sage-grouse habitat quality within the sagebrush biome (Doherty and others, 2022). Steady and long-term declines in sage-grouse populations have led to large-scale efforts to improve population performance and prevent additional loss of habitat for sage-grouse and other sagebrush-dependent species (Coates and others, 2021). Due to their complex space use and habitat selection patterns during different life stages, requirements for large intact tracts of sagebrush, declining population trends, and status as a proposed protected species, sage-grouse have become integral to land management and conservation policy throughout the western United States (Western Association of Fish and Wildlife Agencies, 2015; Doherty and others, 2022).
The conservation and management of sage-grouse populations inhabiting the southwestern extent of the species’ range along the border of California and Nevada demonstrate the central role of the species to land management efforts (Duvall and others, 2017). Both geographic isolation and the absence of continuous sagebrush communities resulted in significant genetic divergence between this metapopulation and neighboring populations in the Great Basin (Oyler-McCance and others, 2014, 2015106; Zimmerman and others, 2023). As a result of this distinctiveness, the population was recognized as the Bi-State Distinct Population Segment (DPS), evaluated separately for listing under the Endangered Species Act (ESA; U.S. Fish and Wildlife Service, 2010, 2013, 2023). The sage-grouse, and the Bi-State DPS in particular, have been the subjects of a long and complex regulatory history. The Bi-State DPS has been repeatedly petitioned for listing as threatened or endangered under the ESA (U.S. Fish and Wildlife Service, 2002, 2006, 2008). However, the Bi-State DPS was determined to be “warranted but precluded” (that is, by higher priority species) for listing in 2010 (U.S. Fish and Wildlife Service, 2010).
The U.S. Fish and Wildlife Service (USFWS) status review and 2010 listing decision highlighted multiple threats to the Bi-State DPS. These threats included population declines and low abundances in some subpopulations, and expansion of pinyon-juniper cover (consisting primarily of single-leaf pinyon pine [Pinus monophylla] and juniper [Juniperus spp.]) into sagebrush communities. Other threats included climate-change related effects, such as drought, which affect productivity and hydrology of sagebrush ecosystems. Changes in predator communities due to anthropogenic effects, and a positive feedback loop between wildfire and exotic annual grass invasion also have contributed to cumulative loss of sagebrush over time.
Coordinated efforts to address these threats began in 2000 with the formation of the Nevada Governor’s Sage Grouse Conservation Team followed by the Bi-State Local Area Working Group (LAWG), comprised of federal and state natural resource agency representatives, private entities and other stakeholders. The LAWG emphasized local community involvement and produced the Greater Sage-Grouse Conservation Plan for the Bi-State Plan Area of Nevada and Eastern California (Nevada Governor’s Sage-Grouse Conservation Team, 2004), which initially identified key threats to the Bi-State DPS and strategies to address those threats. Following the 2010 decision that ESA listing was warranted but precluded, these efforts expanded with the formation of the Executive Oversight Committee (EOC), comprised of top-level state and federal agency officials, and the Technical Advisory Committee (TAC), comprised of technical experts from federal and state agencies and non-governmental organizations. The TAC was then tasked with collaboratively developing the Bi-State Action Plan (Bi-State Technical Advisory Committee, 2012). The Bi-State Action Plan had two main objectives, as mandated by the TAC: (1) to document and summarize conservation actions that had been implemented to benefit the Bi-State DPS since 2004 and (2) to develop an adaptive management strategy to identify and prioritize future conservation actions intended to ensure the long-term conservation of the Bi-State DPS (Bi-State Technical Advisory Committee, 2012). Based on this plan, multiple conservation actions were implemented in the Bi-State region, including widespread conifer removal, sagebrush restoration, fence removal, and treatments to reduce invasive weeds. The Bi-State Action Plan is now nationally recognized as an example of effective collaborative and community-based conservation (Duvall and others, 2017) and is currently (2024) being revised as part of a 10-year update.
In response to ongoing legal challenges and given the demonstrated commitment to the conservation of the Bi-State DPS, as expressed by the formation of the LAWG, TAC, and Bi-State Action Plan, the USFWS concluded that the Bi-State DPS did not warrant listing under the ESA in 2015 and again in 2020 (U.S. Fish and Wildlife Service, 2015, 2020). The 2015 decision withdrawing the proposed rule to list the Bi-State DPS as threatened, stated that, “…the best scientific and commercial data available indicate that the threats to the DPS and its habitat, given current and future conservation efforts, are reduced below the statutory definition of threatened or endangered” (U.S. Fish and Wildlife Service, 2015). However, the U.S. District Court subsequently vacated both the 2015 and 2020 withdrawals of the proposed rules to list the Bi-State DPS (U.S. Fish and Wildlife Service, 2019, 2023), requiring the USFWS to undertake a renewed Bi-State DPS status review and issue a new listing decision, which the USFWS intends to complete by May 2024 (U.S. Fish and Wildlife Service, 2023). Current (2024) timely information regarding short- and long-term sage-grouse population trends, changes in subpopulation status and distribution, and the efficacy of the suite of previously implemented conservation actions in the Bi-State region are required to inform the current (2024) USFWS status review. The U.S. Geological Survey (USGS), state, federal, and university partners have been overseeing the long-term monitoring and research of the Bi-State DPS through both standardized population (lek) counts and tracking of sage-grouse marked with radio and GPS (Global Positioning System) transmitters. This report was prepared in cooperation with the USFWS, Nevada Department of Wildlife, California Department of Fish and Wildlife, Bureau of Land Management, Great Basin Bird Observatory, and U.S. Forest Service and summarizes those data to help inform the USFWS rule-making process. This report, along with data and analyses provided by the USGS in 2020 (Coates and others, 2019), provides the best available data and science regarding population status, trends, and threat abatement in the Bi-State region since the Bi-State DPS was initially proposed for listing in 2013, a date that has been used as a benchmark for subsequent status reviews and conservation effectiveness. Such information can be useful for determining sage-grouse status in the Bi-State DPS while contributing evidence for relative effectiveness of a suite of potential conservation actions aimed at maintaining future stability and persistence of the species.
Objectives
Our primary objective was to provide timely science to inform greater sage-grouse conservation within the Bi-State region to support the current (2024) status review and pending listing decision. Our specific objectives were:
-
1. Evaluate landcover and habitat characteristics associated with habitat selection and reproductive performance of sage-grouse within the Bi-State region (2003–19). Then, identify areas with both high habitat selection and demographic performance to prioritize for conservation and further identify areas of mismatched selection and demographic performance to target for restoration or habitat improvement.
-
2. Concurrently estimate changes in the abundance and distribution of sage-grouse subpopulations within the Bi-State, over three nadir-to-nadir population cycles (1995–2019) and evaluate trends in population rate of change over six population cycles (1969–2019).
-
3. Evaluate the efficacy of conservation actions implemented between 2012 and 2019 within the Bi-State region using a BACIPS study design to estimate concurrent changes in sage-grouse population abundance relative to those not affected by conservation efforts.
Study Area
We carried out this research across a 1.8 million-hectare (ha) region occupied by the Bi-State DPS of sage-grouse (the Bi-State region), along the border of east-central California and western Nevada (fig. 1). We included all leks and subpopulations associated with the following sage-grouse Population Management Units (PMUs): Bodie Hills, Desert Creek/Fales, Long Valley, Mount Grant, Pine Nut, South Mono, and White Mountains (fig. 1A). This topographically diverse region is along the western edge of the southern Great Basin and the eastern side of the Sierra Nevada Mountains, with elevations ranging from 1,386 to 4,344 meters (m). Elevations below 2,100 m are predominantly characterized by sagebrush communities comprised of multiple species of sagebrush (Artemisia spp.) and the understory vegetation is predominantly comprised of native bunchgrasses and perennial forbs. The invasive annual grass cheatgrass (Bromus tectorum) exists throughout the region, and pinyon-juniper woodlands are common at elevations of 1,850–3,000 m.
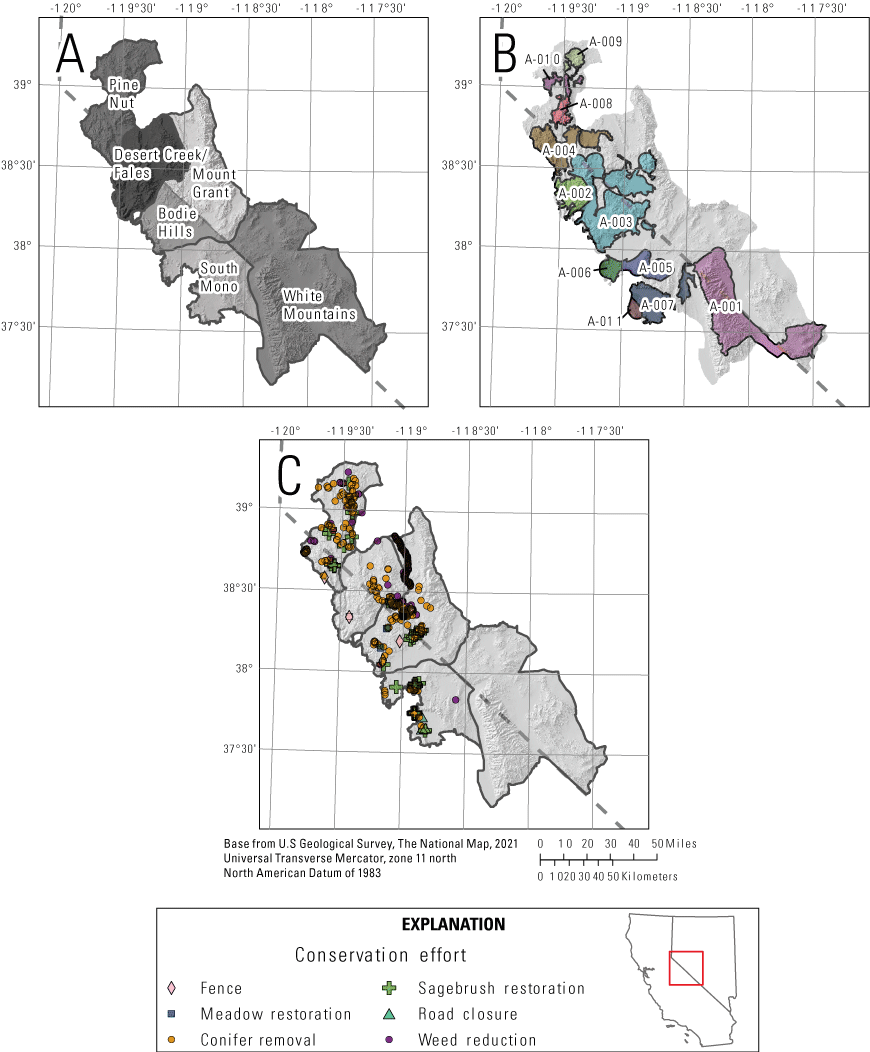
A, Population management units in the Bi-State Distinct Population Segment (DPS) in which greater sage-grouse were monitored from 2003 to 2019 to model and map habitat; B, subpopulations in the Bi-State DPS in which trends in sage-grouse abundance and distribution were monitored from 1995 to 2019; and C, conservation efforts carried out between 2012 and 2019 within the Bi-State DPS.
Field Methods
Personnel from the USGS, Bureau of Land Management, Nevada Department of Wildlife, California Department of Fish and Wildlife, Idaho State University, and Great Basin Bird Observatory carried out annual standardized lek count surveys using established protocols (Connelly and others, 2003). We completed lek counts between 30 minutes before and 90 minutes after sunrise from early March to late April, which spanned the period of peak lek attendance by males. From a suitable viewing location, surveyors used binoculars or spotting scopes to perform three separate counts at 10-minute intervals and recorded the highest male count. A ‘saturation count’ survey has been employed in recent years within portions of the Bodie Hills and South Mono population management units, where all leks are surveyed simultaneously to prevent miscounting individuals that switch between leks over time (Coates and others, 2018).
We captured sage-grouse across the Bi-State region during the spring (March to May) and fall (September to November) from 2003 to 2022 using standard spotlighting techniques (Wakkinen and others, 1992). We fitted each captured sage-grouse with either a necklace-style (very-high frequency; VHF) transmitter (less than 3 percent body mass; Advanced Telemetry Systems, Inc., Isanti, Minnesota) equipped with a mortality sensor that would activate after 8 hours of inactivity or a rump-mounted GPS platform transmitting terminal (less than 5 percent body mass; GPS; GeoTrak, Inc., Apex, North Carolina) equipped with both GPS and VHF transmitters. We classified sage-grouse by age and sex using published methods (Ammann, 1944). We managed all sage-grouse capture and handling in accordance with the USGS Western Ecological Research Center (WERC) Animal Care and Use Protocol WERC-2015-02.
We tracked radio-marked sage-grouse using a three-element Yagi antenna (Advanced Telemetry Systems, Inc., Isanti, Minnesota) and a portable receiver (Communication Specialist, Inc., Orange, California) at least two times per week during reproductive periods. During the fall and winter, we obtained location data for radio-marked individuals at approximate monthly intervals using fixed-wing aircraft. Sage-grouse were tracked to determine both reproductive status and mortality. If a female bird occupied the same location during two consecutive telemetry fixes, we visually confirmed nesting status, and nests were considered successful if at least one chick hatched. Females who nested successfully were then located and checked for brood presence every 10 days for as many as 50 days. Some checks were done at night, allowing the presence or absence of chicks with the marked female to be confirmed with spotlights. At 28 (2010–11), 35 (2007–09, 2021–22), or 50 (2003–05) days post-hatch, depending on the monitoring year, the entire brood was flushed and the number of chicks counted to determine a final estimate of each brood size.
Objective 1. Map Sage-Grouse Habitat Selection and Demographic Performance within the Bi-State Distinct Population Segment
Purpose
Mapping sage-grouse habitat suitability can guide conservation and restoration efforts in threatened sagebrush ecosystems. However, habitat modeling has typically focused on resource selection models that analyze known locations of individual birds relative to random locations. One weakness of such an approach that relies exclusively on location data is that it lacks information on species demographic performance (for example, survival or reproduction). An additional consideration is the concept of seasonal or life stage-specific responses, where habitat needs may differ depending on the time of year or whether an individual is reproductively active. Finally, approaches that delineate habitat suitability should also consider the distribution of current occupancy, which can be combined with fine-scale data on habitat potential to delineate areas for preservation or restoration. Our objective was to model and map habitat selection patterns of sage-grouse during specific seasons and life stages, while also incorporating measures of demographic performance to identify habitat suitability. Incorporating demographic performance into mapping products builds upon previous modeling efforts completed for the Bi-State DPS. By intersecting the composite indices of selection and performance with the current distribution of sage-grouse, we provide an example of how agencies can use these mapping products to categorize the landscape according to management priority and inform conservation and restoration. These data are needed to help identify priority habitat for sage-grouse in the Bi-State region and to assess whether ongoing conservation efforts are effectively targeting priority areas for conservation and restoration.
Methods
Spatial Data Compilation
We compiled multiple spatially and temporally explicit remotely sensed data layers to evaluate the effects of environmental characteristics on sage-grouse habitat selection and demographic performance across reproductive life stages and seasons (table 1). We used data from the year each radio-marked bird was monitored for temporally varying covariates. Because animals commonly select habitat in a scale-dependent manner (Boyce, 2006), we evaluated the effects of land cover variables at multiple spatial scales. We first calculated the proportion of each land cover covariate or density of linear features within circular moving windows with radii representing the averages of minimum (167.9 m), mean (439.5 m), and maximum (1,451.7 m) daily distances typically traveled by sage-grouse (Coates and others, 2016a). Movement patterns may differ during reproductive life stages, when a female is associated with either a single nest site or a brood with limited mobility. Therefore, we included additional spatial scales for nesting and brood-rearing analyses, including a finer scale (75 m), representing the core (that is, most consistent) area used by nesting females during incubation recesses, and an intermediate scale (260 m), representing the average distance moved during incubation recesses (Dudko and others, 2019). An additional scale captured the median movement distance of a female with a brood during either the early (260 m) or late (370 m) brood-rearing period (Coates and others, 2024a). We transformed distance-based predictors using an exponential decay function where e=exp(−d/α) and α represented the mean value at all locations for a given analysis (Coates and others, 2016a), which allowed for the effect to decay with increasing distance. We scaled and centered all variables before analysis.
Table 1.
Environmental variables derived from remotely sensed land cover products evaluated in analyses examining habitat selection and survival for greater sage-grouse in the Bi-State Distinct Population Segment from 2003 to 2019.[Temporally varying variables were available across the entire study period. The spatial resolution for all variables was 900 square meters unless otherwise noted. Abbreviations: RCMAP, Rangeland Condition Monitoring Assessment and Projection; Y, yes; N, no; CC1, phase 1 conifer encroachment; CC2, phase 2 conifer encroachment; USFWS, U.S. Fish and Wildlife Service; DEM, digital elevation model]
Variable | Category | Description/source | Time- varying? |
References |
---|---|---|---|---|
Percentage sagebrush | Shrubs | RCMAP time-series layers | Y | Rigge and others (2021) |
Sagebrush height | Shrubs | RCMAP basemap | N | Rigge and others (2020) |
Percentage shrubs | Shrubs | RCMAP time-series | Y | Rigge and others (2021) |
Shrub height | Shrubs | RCMAP basemap | N | Rigge and others (2020) |
Percentage herbaceous vegetation | Herbaceous/wet meadows | RCMAP time-series | Y | Rigge and others (2021) |
Percentage bare ground | Bare ground | RCMAP time-series | Y | Rigge and others (2021) |
Annual grass cover1 | Annual grass | Invasive annual grass cover | Y | Boyte and Wylie (2016) |
Pinyon-juniper cover | Conifer cover | Two cover classes of pinyon-juniper cover (Phase 1/CC1=0−10-percent tree canopy cover, Phase 2/CC2=10−20-percent tree canopy cover) | N | Gustafson and others (2018) |
Annual burned area1 | Burned area | Annual burned area with simulated recovery | Y | O’Neil and others (2020) |
Streams | Streams | National Hydrography Dataset | N | U.S. Geological Survey (2017) |
Springs | Springs | National Hydrography Dataset | N | U.S. Geological Survey (2017) |
Wet meadows | Herbaceous/wet meadows | USFWS National Wetland Inventory | N | U.S. Fish and Wildlife Service (2022) |
Saline lakes | Saline lakes | National Hydrography Dataset | N | U.S. Geological Survey (2017) |
Elevation | Elevation | Extracted from DEM | N | Gesch and others (2009) |
Slope | Topography | Calculated from DEM | N | Evans and others (2014) |
Topographic roughness | Topography | Calculated from DEM | N | Evans and others (2014) |
Heat load index | Temperature/moisture | Calculated from DEM | N | Evans and others (2014) |
Compound topographic index | Temperature/moisture | Calculated from DEM | N | Evans and others (2014) |
Transformed aspect | Temperature/moisture | Calculated from DEM | N | Evans and others (2014) |
Analysis
Our analysis assessed both habitat selection and survival for three seasons (spring, summer, and winter) and two reproductive life stages, nesting and brood-rearing, with the latter stage further divided into early (less than or equal to 21 days post-hatch) and late (greater than 21 days post-hatch) brood-rearing periods (Blomberg and others, 2014). We defined spring as 16 March to 30 June, summer as 1 July to 15 October, and winter as 16 October to 15 March. For habitat selection analyses, we used resource selection functions (RSFs) to compare used and available points (Manly and others, 2002), and our selection model (eq. 1) took the following form:
whereY
represents whether a point was used or available,
β0
is the baseline intercept,
Xβ
is a vector of selection coefficients multiplied by the matrix of fixed environmental covariates, and
κ, η, and υ
are random effects for site, year, and individual bird or brood, respectively.
We used logistic-exposure models in a Bayesian framework to model survival for each reproductive life stage (Shaffer, 2004; Sinnott and others, 2022). We calculated daily survival (eq. 2) using the following form:
whereDS
is the daily survival probability over interval i,
γ0
is the baseline intercept,
Xβ
is a vector of selection coefficients multiplied by the matrix of fixed environmental covariates, and
κ and η
are random effects for site and year, respectively.
A single “best” scale for all habitat variables rarely exists because relationships between wildlife and habitat are typically scale-dependent (Stuber and Fontaine, 2019). To address this, we used Bayesian latent indicator variable scale selection (BLISS; Stuber and others, 2017) to evaluate the most influential scale among each group of variables (see the “Category” column in table 1). BLISS estimates latent scale indicator variables with reversible-jump Markov chain Monte Carlo (MCMC) sampling to evaluate the scale with highest statistical support within a group, without issues of collinearity (Stuber and others, 2017). We grouped variables into 12 categories to represent similar features and correlated variables (|r|≥0.5), such that no variables between groups were highly correlated while co-occurring in the model (table 1). Full results of variable selection analyses are reported in appendix 1.
We fit a final model to include only the most influential variables from each group to allow for straightforward inferences. To evaluate statistical support, we calculated the probability of direction (P(|β|>0; Makowski and others, 2019) and considered probabilities ≥0.85 and ≥0.95 to represent moderate and strong evidence of effects, respectively. We also calculated relative selection strengths (RSS), which provide an estimate of the relative intensity of two locations differing by one standard deviation and assuming that both locations were equally available (Avgar and others, 2017). Values greater than one represented a positive effect on habitat selection, whereas values less than one represented a negative effect.
All models were fit using MCMC simulations with Just Another Gibbs Sampler (JAGS version 4.3.0, mcmc-jags.sourceforge.net, accessed March 2021) in the “R2Jags’ package (Su and Yajima, 2015). We included vague normal priors for random effects and their measures of error (Kéry, 2010), and to prevent overfitting, we specified Lasso (that is, Laplace) prior distributions for each habitat covariate specifying an uninformative hyperprior for the tuning parameter lambda (Park and Casella, 2008; Hooten and Hobbs, 2015). We ran each model for 30,000 iterations with a thinning factor of 5, discarded the first 20,000 samples, and made inferences based on the remaining 6,000 samples from 3 independent MCMC chains. To evaluate convergence, we assessed MCMC chain mixing visually and based on Gelman-Rubin convergence statistics (<1.1; Gelman and Hill, 2006).
Model Validation
We used independent testing data from 50 individual birds or broods randomly withheld to validate each model’s predictive ability. To validate selection models, we used cross-validation methods for RSFs (Johnson and others, 2006) and used-habitat calibration (UHC) plots (Fieberg and others, 2018). The UHC plots compare observed distributions for each habitat variable to the expected distribution based on the model (Fieberg and others, 2018) and use the fitted RSF to generate a predictive distribution of used habitat that is compared to the independent testing data for each habitat variable. To validate survival models, we generated survival predictions based on the posterior distributions from the final model. We then created predicted survival curves that were compared to the observed survival curves from the independent testing data and calculated a post-hoc Bayesian predictive P-value (Gelman and others, 2013), with values near 0 or 1 indicating poor model fit (Schmidt and others, 2010).
Selection and Survival Mapping
We mapped predicted selection and survival for each reproductive life stage and season across the Bi-State region using estimates from the final models and the spatial layers described above (Coates and others, 2024a). We used the median value of the posterior distribution and applied those in the model equations outlined above, where the matrix X represented the raster values for each covariate across each 900-square meter (m2) pixel. We used raster values from 2021 for time-varying covariates because that represented the most recent nadir in population abundance based on an integrated population model (see the “Objective 2” section). Distance to lek or nest was not included in the habitat selection maps because we were interested in the spatial distribution of areas suitable for sage-grouse based on underlying habitat characteristics, whereas distance to lek would provide information on the distribution of occupied habitat that was addressed through integration with an abundance and space use index (ASUI; see below) following Coates and others (2016a).
To create selection maps, we transformed estimates using a habitat selection index (HSI), where and indicates habitat use proportional to availability on a scale of 0‒1 (Coates and others, 2016a). We then implemented the isopleth method at used locations with cutoff values at the 50th, 25th, and 5th percentiles to categorize the continuous selection surface into four categories (non-habitat, low, moderate, and high selection; Doherty and others, 2016; O’Neil and others, 2020). For survival maps, we exponentiated values of daily survival based on the number of days in either the nesting or brood-rearing periods (nesting=38 days, early brood-rearing=21 days, late brood-rearing=28 days) to calculate cumulative survival. We then categorized the continuous survival surfaces into four categories based on the distribution of values at failed and successful points following O’Neil and others (2020).
We then calculated reproductive selection and survival indices using the continuous selection and survival surfaces for each reproductive life stage to represent the entire reproductive period. We first relativized the habitat selection indices for each life stage by dividing by the maximum value in that life stage (Coates and others, 2019), thus weighting all reproductive life stages equally, and then multiplied the three relativized surfaces together. We categorized the reproductive selection surface into four categories as described for the individual selection layers. To calculate the survival index, we did not relativize values because the outputs represented true survival probabilities. We therefore multiplied the exponentiated survival surfaces during each life stage to create the reproductive survival index, which we categorized using the same methods for individual survival maps. We did not calculate the survival index for pixels that were considered non-habitat based on the reproductive selection index.
For each reproductive life stage, we created a ranked index that represented the overlap between the categorized selection and survival maps for each life stage and the reproductive indices. Pixels that had both high selection and survival constituted the highest habitat rank and were assumed to represent productive habitat for that life stage. In contrast, pixels that had high selection but low predicted survival were assigned the lowest rank because they could represent maladaptive habitat selection, potentially contributing to ecological traps.
We also created maps of example habitat management categories as an example for managers, which represented the overlap between the reproductive selection index, reproductive source habitat, and an ASUI that was calculated from breeding sage-grouse (lek count) surveys (Coates and others, 2016a) and allowed us to delineate high-quality habitat occupied by sage-grouse. We included the ASUI to represent the distribution of habitat that was predicted to be occupied by sage-grouse after accounting for the configuration of leks, the distance to leks, and the predicted abundance at each lek over time. We combined the ASUI with the reproductive selection map and identified source habitat (high selection and high survival in any reproductive life stage) to differentiate between likely occupied habitat and potential habitat that had zero or low occupancy. We delineated four example habitat management categories: (1) priority+, assumed to represent important source habitat for each reproductive life stage supporting both high selection and high survival within high use areas based on the ASUI; (2) priority areas that had either predicted high, moderate, or low selection (but excluded non-habitat) within high use areas based on the ASUI or were source areas (that is, high selection and high survival) outside of high use areas; (3) general areas that represented either the intersection between high selection and low to no use from the ASUI or non-habitat within high use areas based on the ASUI; and (4) other areas where there was moderate selection combined with low to no use from the ASUI.
Finally, we created separate habitat layers for each population nadir (1995, 2001, 2008, and 2021) estimated from an integrated population model (see the “Objective 2” section) for each life stage and season using time-varying remotely sensed vegetation cover layers to capture changes in habitat over time (Coates and others, 2024b). Time-varying covariates included sagebrush cover, shrub cover, herbaceous cover, bare ground, annual grass cover, and cumulative burned area (table 1). We also calculated composite selection, survival, and habitat suitability indices to capture overall changes in time. To create the annual composite selection index, we first calculated seasonal composite selection layers, where the spring composite selection index was a combination of seasonal spring, nest, and early brood-rearing selection layers, the summer composite selection index included seasonal summer and late brood-rearing selection layers, and the winter composite selection index only included the seasonal winter selection layer. To calculate the seasonal composite selection indices, we first relativized each layer by dividing by its maximum value and then added the individual selection components together. We then relativized the seasonal composite selection indices, added them together, and scaled the resulting layers between 0 and 1 to calculate the annual composite selection index. To create the annual composite survival index, we followed the same procedure but only created seasonal composite survival indices for spring, which included nest and early brood-rearing survival layers, and summer, which only included the late brood-rearing survival layer. To create the annual composite habitat suitability index, we first added the seasonal composite selection and survival indices together and relativized them to create seasonal composite suitability indices for spring, summer, and winter. We then added the three seasonal composite suitability layers together and scaled the resulting layer between 0 and 1 to calculate the annual composite habitat suitability index. For each selection layer (spring, summer, winter, nest, early brood, and late brood), survival layer (nest, early brood, and late brood), and the composite indices (selection, survival, and suitability), we calculated two metrics: (1) the percentage change in area excluding the lowest category (that is, excluding non-habitat for selection layers and very low survival for survival layers) based on the categorized surface from three previous population nadirs (1995, 2001, and 2008) to the most recent nadir (2021) based on an integrated population model (see the “Objective 2” section) and (2) the percentage change in the median value of the continuous surface between the three previous population nadirs and the most recent nadir. All values are reported in appendix 2. We also calculated the percentage change in distribution between previous population nadirs and the most recent nadir, where distribution was calculated as the intersection between the annual composite selection index after excluding non-habitat and the abundance and space use index, with values reported in appendix 2.
Results: Objective 1
We monitored 579 nests belonging to 419 females between 2003 and 2019. Over the 38-day laying and incubation period, average nest survival (95-percent credible interval [CRI]) was 0.38 (0.31–0.47). We monitored 285 broods during the early period (≤21 days post-hatch) and 209 during the late period, with survival across the 50-day brood-rearing period estimated as 0.74 (0.45–0.91).
Nest Selection and Survival
During the nesting life stage, the relative probability of selection increased with taller sagebrush and at higher elevations and decreased with greater herbaceous cover, pinyon-juniper cover, bare ground, burned area, streams, springs, saline lakes, and in areas with south-southwesterly aspects (fig. 2; table 2). Model validation indicated that the nest selection model had reasonable out-of-sample predictive capabilities (Spearman’s rank ρ=0.98, R2=0.85, βpredict=0.64), and UHC plots demonstrated that model-predicted habitat was consistent with observed nest locations.
Table 2.
Coefficient estimates (β) for nest site selection and nest survival for greater sage-grouse in the Bi-State Distinct Population Segment from 2003 to 2019.[The 95-percent credible intervals and the best selected scale for each group are noted parenthetically. Abbreviations: %, percent; CRI, credible intervals; P, probability; |β|, beta, the absolute value of the coefficient estimate; >, greater than; hgt, height; m, meters; CC1, phase 1 conifer encroachment; CC2, phase 2 conifer encroachment; CBA, cumulative burned area; CTI, compound topographic index; —, not applicable]
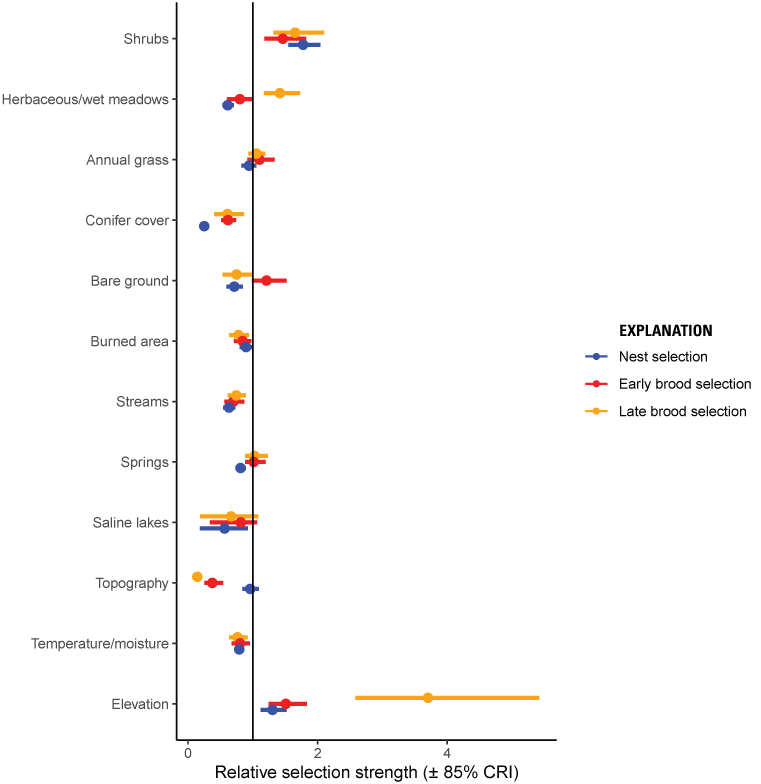
Relative selection strength (plus or minus [±] 85-percent [%] credible intervals [CRI]) of variables predicting nest (blue), early brood (red), and late brood selection (orange) for greater sage-grouse in the Bi-State Distinct Population Segment from 2003 to 2019. Values above one (vertical black line) correspond to selection and values below one correspond to avoidance. For distance variables, estimates below one correspond to higher selection closer to the feature.
Survival of nests increased with more burned area, shrubs, more topographic roughness, and at higher elevation (fig. 3; table 2). Although the positive effects of burned area and shrubs may appear contradictory, they were operating at different spatial scales and a positive effect of burned area at 1,451 m does not necessary imply less shrub cover within 75 m of a specific location. In addition, selection and survival were misaligned for burned areas with apparent avoidance but higher survival in burned areas. However, only 2 percent of nests were within a fire perimeter, so the moderate positive relationship with burned areas was interpreted with caution. Model validation indicated that predicted survival curves were consistent with testing data (Bayesian P-value=0.55).
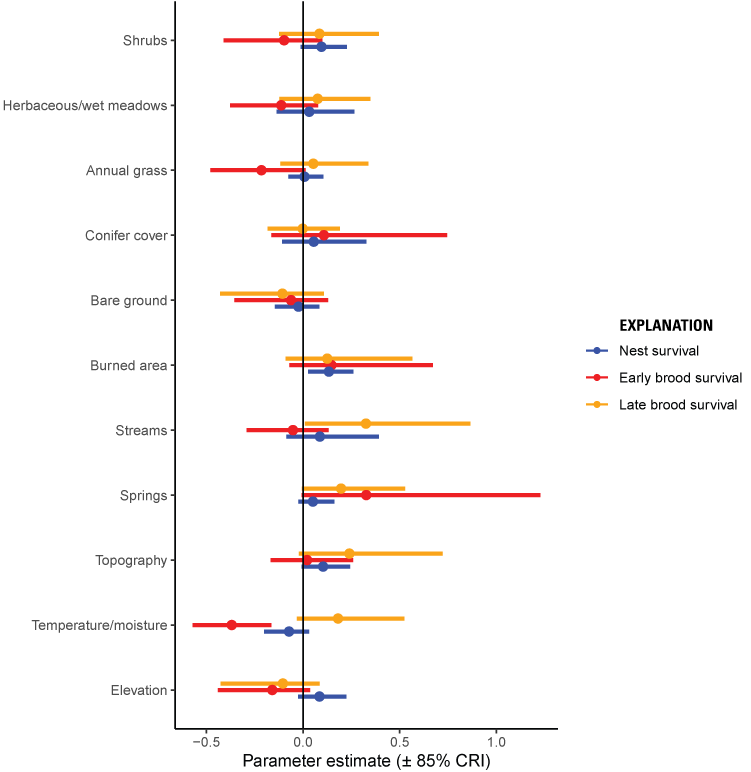
Parameter estimates (plus or minus [±] 85-percent [%] credible intervals [CRI]) of variables predicting nest (blue), early brood (red), and late brood survival (orange) for greater sage-grouse in the Bi-State Distinct Population Segment from 2003 to 2019. Positive estimates for distance variables correspond to higher survival closer to the feature.
Early Brood Selection and Survival
During the early brood-rearing period, the relative probability of selection increased with taller sagebrush, more bare ground, and higher elevations, but decreased with more wet meadows, pinyon-juniper cover, more cumulative burned area, more perennial streams, greater topographic roughness, and higher heat load index (fig. 2; table 3). Model validation indicated that the early brood selection model had strong out-of-sample predictive capabilities (Spearman’s rank ρ=0.99, R2=0.96, βpredict=1.00), and UHC plots showed that model-predicted habitat was consistent with observed early brood locations.
Table 3.
Coefficient estimates (β) for early brood selection and survival for greater sage-grouse in the Bi-State Distinct Population Segment from 2003 to 2019.[The 95-percent credible intervals and the best selected scale for each group are noted parenthetically. Abbreviations: %, percent; CRI, credible intervals; P, probability; |β|, beta, the absolute value of the coefficient estimate; >, greater than; hgt, height; m, meters; CC1, phase 1 conifer encroachment; CC2, phase 2 conifer encroachment; CBA, cumulative burned area; HLI, heat load index; —, not applicable]
During the early brood-rearing phase, survival was predicted to increase with more burned area and closer to conifer cover and decrease with more shrubs and more perennial streams (fig. 3; table 3). Model validation indicated that predicted survival curves were consistent with testing data (Bayesian P-value=0.55). Selection and survival only differed for shrubs, burned area, and conifer cover, with moderate or strong effects. Early broods tended to select areas with taller shrubs, but experienced higher survival in areas with lower shrub density. Early broods also tended to select areas with less burned area closer to conifer cover, which was associated with lower survival, although there was high uncertainty around the effect of conifer cover on early brood survival.
Late Brood Selection and Survival
During the late brood-rearing period, the relative probability of selection was higher with taller sagebrush, more herbaceous cover, and at high elevations; however, it was lower with more pinyon-juniper cover, bare ground, cumulative burned area, perennial streams, saline lakes, steeper slopes, and south-southwesterly aspects (fig. 2; table 4). Model validation indicated that our late brood selection model had reasonable out-of-sample predictive capabilities (Spearman’s rank ρ=0.95, R2=0.62, βpredict=0.60), and UHC plots demonstrated that model-predicted habitat was consistent with observed late brood locations.
Table 4.
Coefficient estimates (β) for late brood selection and survival for greater sage-grouse in the Bi-State Distinct Population Segment from 2003 to 2019.[The 95-percent credible intervals and the best selected scale for each group are noted parenthetically. Abbreviations: %, percent; CRI, credible intervals; P, probability; |β|, beta, the absolute value of the coefficient estimate; >, greater than; hgt, height; m, meters; CC1, phase 1 conifer encroachment; CBA, cumulative burned area; CTI, compound topographic index; —, not applicable]
Survival during the late brood-rearing period increased with more intermittent streams, more springs, steeper slopes, and higher values of the compound topographic index (fig. 3; table 4). Model validation indicated that predicted survival curves were consistent with testing data (Bayesian P-value=0.55).
Selection and survival differed for two variables with moderate or strong effects during the late brood-rearing period: streams and slope. Selection was lower but survival higher in places with more intermittent or perennial streams. Selection was also higher but survival lower with steeper slopes.
Spring Selection
The relative probability of selection during the spring increased with more sagebrush cover, annual grass cover, and at higher elevations (fig. 4; table 5). The relative probability of selection decreased with greater pinyon-juniper cover, more wet meadows, more cumulative burned area, more springs and streams, greater topographic roughness, more south-southwesterly aspects, and more saline lakes (fig. 4; table 5). Our spring selection model had reasonable out-of-sample predictive capabilities (Spearman’s rank ρ=0.99, R2=0.85, βpredict=0.79), and UHC plots demonstrated that model-predicted habitat was consistent with observed spring locations.
Table 5.
Coefficient estimates (β) for spring, summer, and winter selection for greater sage-grouse in the Bi-State Distinct Population Segment from 2003 to 2019.[The 95-percent credible intervals and the best selected scale for each group are noted parenthetically. Abbreviations: %, percent; CRI, credible intervals; P, probability; |β|, beta, the absolute value of the coefficient estimate; >, greater than; m, meters; hgt, height; CC1, phase 1 conifer encroachment; CBA, cumulative burned area; HLI, heat load index]
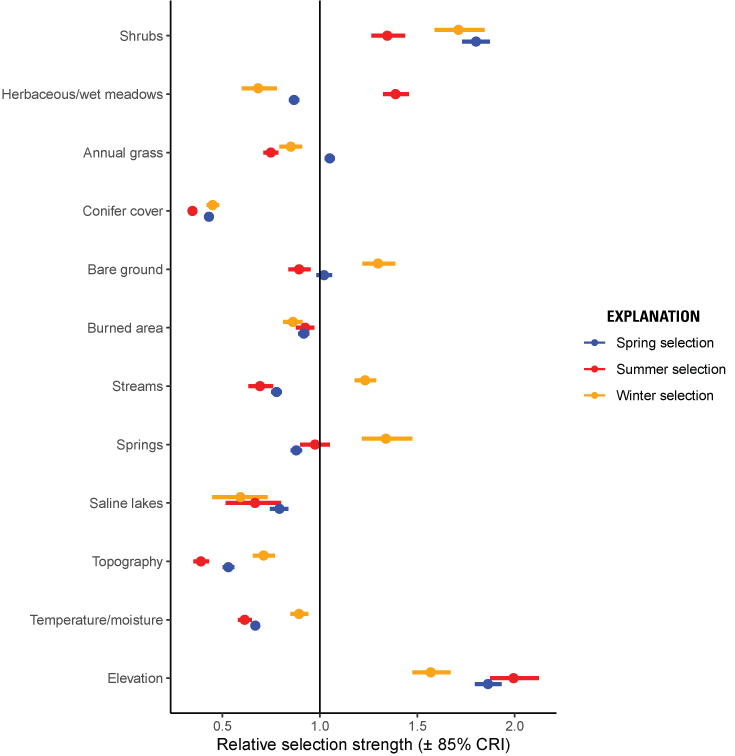
Relative selection strength (plus or minus [±] 85-percent [%] credible intervals [CRI]) of variables predicting spring (blue), summer (red), and winter (orange) selection for greater sage-grouse in the Bi-State Distinct Population Segment from 2003 to 2019. Values above one (vertical black line) correspond to selection, and values below one corresponds to avoidance. For distance variables, estimates below one correspond to higher selection closer to the feature.
Summer Selection
The relative probability of selection during the summer increased with more sagebrush cover, more wet meadows, at higher elevations, and closer to perennial streams (fig. 4; table 5). During the summer, the relative probability of selection decreased with more annual grass cover, more pinyon-juniper cover, more cumulative burned area, more bare ground, higher heat load index, greater topographic roughness, and more saline lakes (fig. 4; table 5). Our summer selection model had reasonable out-of-sample predictive capabilities (Spearman’s rank ρ=0.98, R2=0.85, βpredict=0.83), and UHC plots demonstrated that model-predicted habitat was consistent with observed summer locations.
Winter Selection
The relative probability of selection during the winter increased with greater sagebrush height, more bare ground, more intermittent streams, higher elevations, and greater proximity to wet meadows (fig. 4; table 5). During the winter, the relative probability of selection decreased with more annual grass cover, more pinyon-juniper cover, more cumulative burned area, greater topographic roughness, more south-southwesterly aspects, more saline lakes, and greater proximity to springs (fig. 4; table 5). Our winter selection model had reasonable out-of-sample predictive capabilities (Spearman’s rank ρ=0.98, R2=0.84, βpredict=0.86), and UHC plots demonstrated that model-predicted habitat was consistent with observed winter locations.
Mapping
We mapped habitat selection (fig. 5) and survival (fig. 6) for reproductive life stages and three seasons (fig. 7), and we created combined selection-survival maps for the reproductive life stages (fig. 8). For the reproductive period, we calculated composite selection and survival indices across the Bi-State region by combining the selection and survival maps for the three reproductive life stages. Based on the composite reproductive selection index, 5.3 percent of the Bi-State region was classified as high selection (844 km2), 15.2 percent as moderate (2,419 km2), 41.2 percent as low selection (6,536 km2), and 38.3 percent as non-habitat (6,325 km2; fig. 5). Of the area classified as habitat, a total of 17.2 percent of the Bi-State region was classified as high survival (1,684 km2), 19.9 percent as moderate (1,947 km2), 14.8 percent as low (1,445 km2), and 48.1 percent as very low (4,621 km2; fig. 6). Based on the ranked index that combined selection and survival maps during reproductive life stages, productive habitat (high or moderate selection paired with high or moderate survival) represented 14.1 percent (1,375 km2), whereas areas with potentially maladaptive selection (high or moderate selection paired with low or extremely low survival) represented 19.3 percent of the Bi-State DPS (1,888 km2; fig. 8). We also delineated example habitat management categories as an example for managers using the reproductive selection map and the ASUI, with priority+ representing 3.3 percent (559 km2), priority areas representing 16.8 percent (2,829 km2), general areas representing 7.4 percent (1,243 km2), and other areas representing 6.1 percent (1,033 km2) of the Bi-State region (fig. 9).
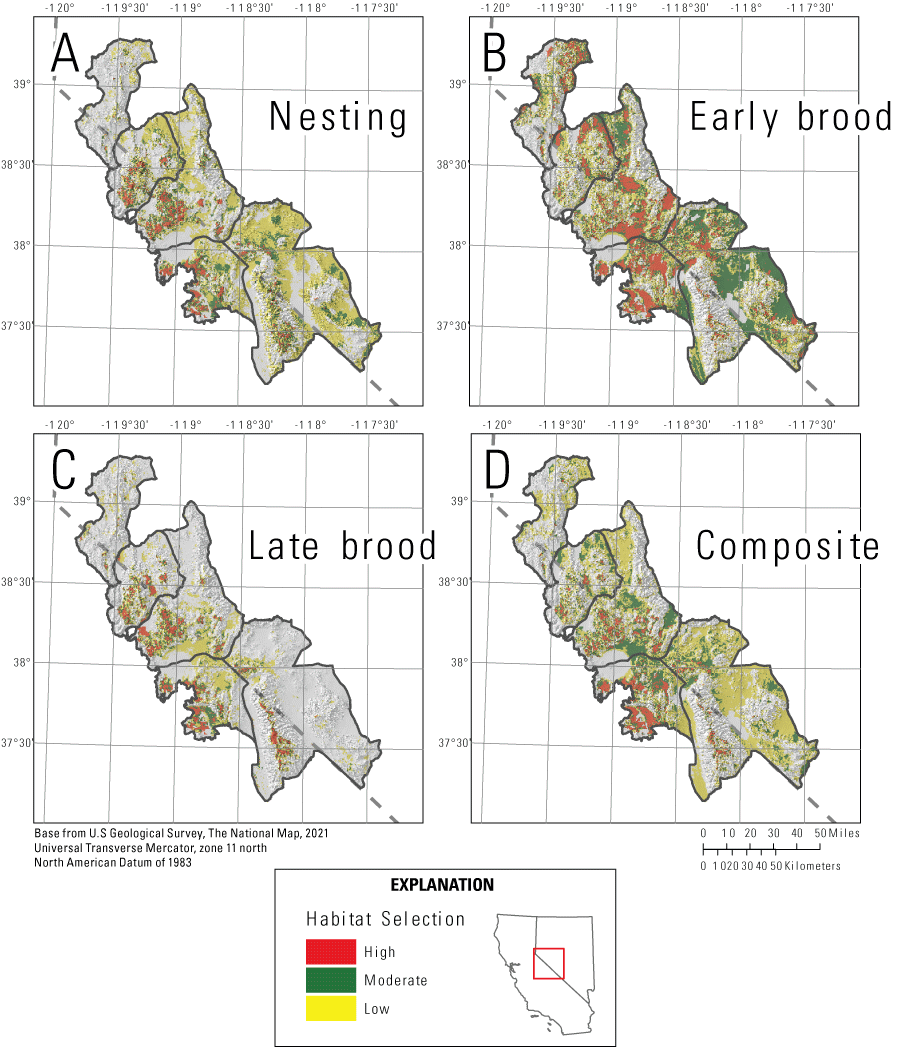
Maps of categorized habitat selection for greater sage-grouse in the Bi-State Distinct Population Segment from 2003 to 2019 during A, nesting; B, early brood-rearing; C, late brood-rearing; and D, a reproductive selection index combining the three life stages.
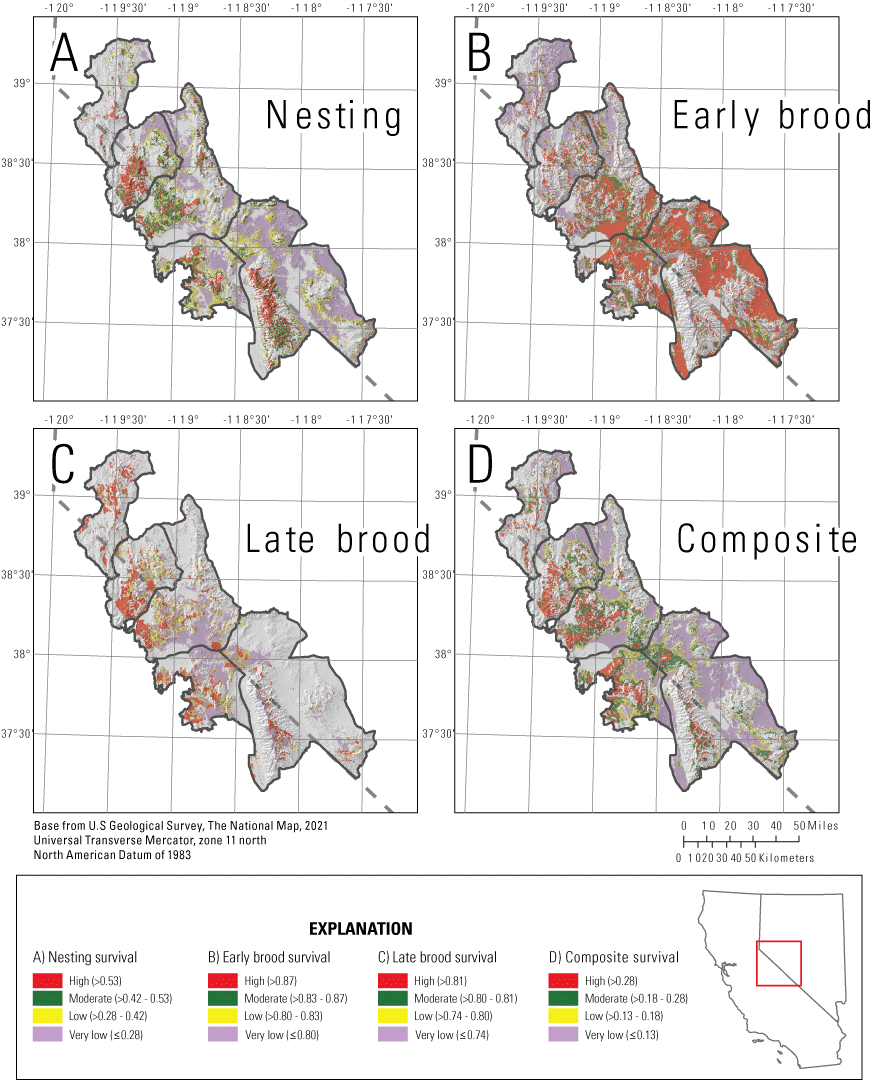
Maps of categorized A, nesting survival; B, early brood survival; C, late brood survival; and D, a reproductive survival index combining the three life stages for greater sage-grouse in the Bi-State Distinct Population Segment from 2003 to 2019. Survival rates are noted parenthetically as greater than (>) or less than or equal to (≤). Survival was not evaluated in areas considered non-habitat based on selection.
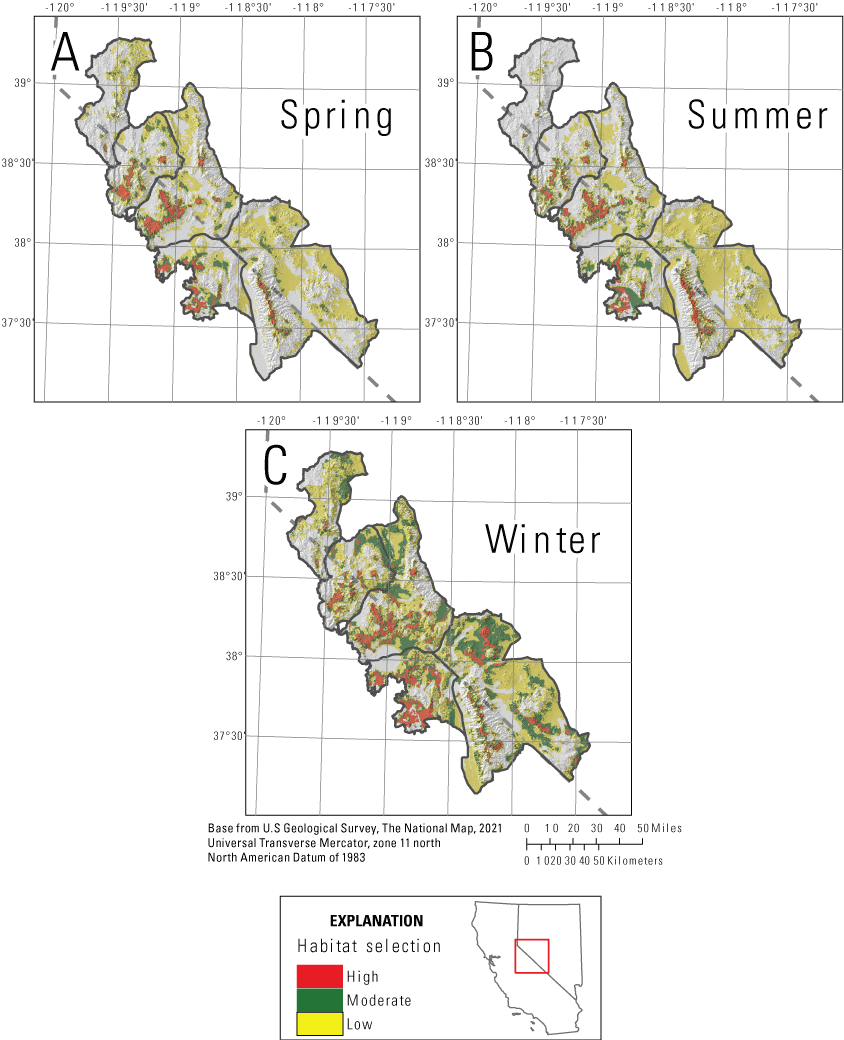
Maps of categorized habitat selection for greater sage-grouse in the Bi-State Distinct Population Segment from 2003 to 2019 during A, spring; B, summer; and C, winter.
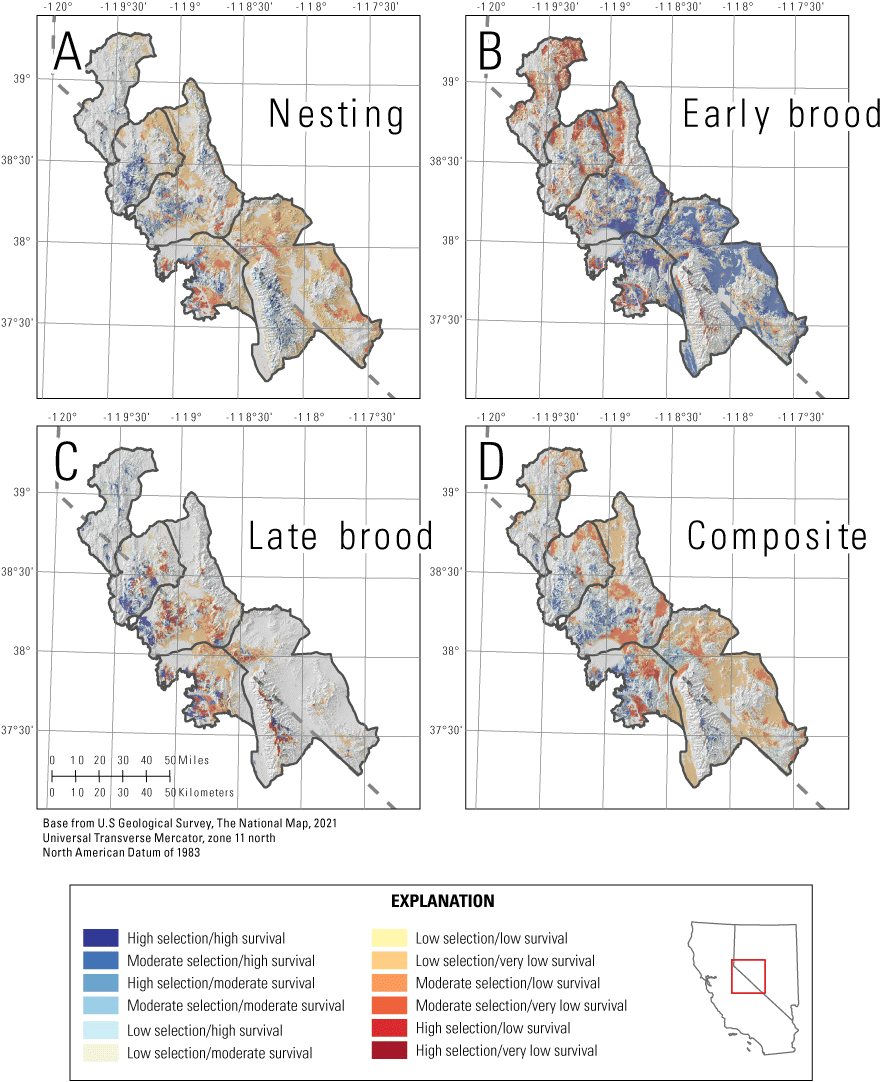
Maps of the ranked index for greater sage-grouse A, nesting; B, early broods; C, late broods; and D, a reproductive index combining the three life stages in the Bi-State Distinct Population Segment from 2003 to 2019. Habitat is ranked from high (blue; high or moderate selection and high or moderate survival) to low (red; high or moderate selection and low or very low survival).
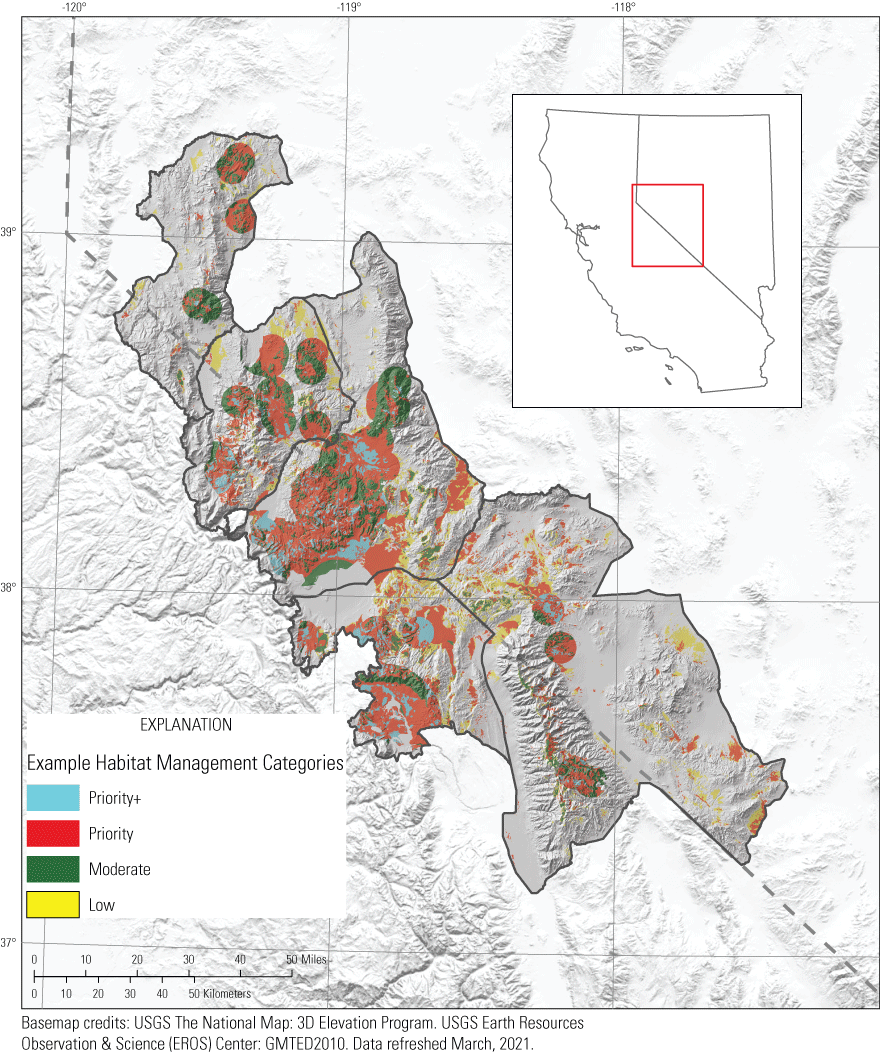
Example habitat management categories based on reproductive habitat selection, reproductive source habitat, and the abundance and space use index of greater sage-grouse in the Bi-State Distinct Population Segment from 2003 to 2019.
Objective 2. Evaluate Population Abundance, Trends, and Distribution of Sage-Grouse within the Bi-State Distinct Population Segment
Purpose
Changes in abundance are commonly used to evaluate trends and status of wildlife populations (Krebs, 2001), but focusing only on abundance can mask important losses in distribution that could lead to long-term population instability, such as when peripheral or satellite populations become more vulnerable to extirpation (Ehrlén and Morris, 2015). Declines in sage-grouse populations have resulted from a wide range of disturbances and land cover changes, which have collectively reduced ecological integrity, wildlife habitat availability, and habitat suitability within the sagebrush biome (Doherty and others, 2022). Sage-grouse population losses are assumed to be due to both habitat loss, resulting in a smaller area occupied, and habitat degradation, resulting in reduced population performance in occupied habitat (Schroeder and others, 2004); however, the relative contributions of these processes to overall population decline remain poorly understood. Our objective was to concurrently estimate changes in both population abundance and spatial distribution over time using population counts, demographic data, and information on space use from marked individuals. A better understanding of trends in both abundance and distribution of sage-grouse in the Bi-State DPS can inform the current (2024) USFWS status review and upcoming decision whether to list the Bi-State DPS under the ESA. It could also inform future conservation actions to reduce declines and reverse long-term negative population trends.
Methods
Data Compilation
We cleaned and filtered lek count data following O’Donnell and others (2021) and Coates and others (2021), restricting analyses to leks for which there were sufficient data to reliably estimate trends. Sage-grouse populations demonstrate cyclic patterns in abundance, and estimates of population change can be sensitive to where the start and end points occur relative to population cycles (Garton and others, 2011; Edmunds and others, 2018; Coates and others, 2021). Therefore, we used lek counts spanning 1960–2023 to identify nadirs in population abundance. Analyses were necessarily restricted to data from known leks and unknown leks may occur throughout the Bi-State DPS, particularly in the White Mountains which are difficult to access reliably in the spring. However, we accounted for unknown leks by applying a correction factor for lek detection when calculating total abundance in the analyses described below.
Following Coates and others (2021) and O’Donnell and others (2022), we assigned all modeled leks and radio-marked sage-grouse to a subpopulation based on their location and capture location, respectively. Subpopulations within the Bi-State DPS were developed using spatial clustering algorithms delineating the geographic structure of sage-grouse distributions based on the spatial arrangement of leks and barriers to connectivity (O’Donnell and others, 2022). Subpopulations were assumed to represent closed population units, wherein immigration and emigration were negligible and changes in abundance were more likely to be driven by changes in demographic rates than migration. The Bi-State DPS included 11 subpopulations, and we had sufficient data collected from leks and individually marked birds within 9 of those subpopulations (fig. 1).
Methods: Population Modeling and Estimated Abundance
To model trends in population abundance, we used two models that spanned different time frames to align with and make best use of available data sources. Because reliable population counts were available starting in 1960, we used a population state-space model (SSM; Royle and Dorazio, 2009; Kéry and Schaub, 2012) to estimate the population rate of change () over six distinct nadir-to-nadir cycles: long-term (1969–2019), medium/long-term (1978–2019), medium-term (1983–2019), short/medium-term (1995–2019), short-term (2002–19), and recent-term (2008–19) following Coates and others (2023). Consistent demographic data from marked birds was available starting in 2003 and we therefore also used an integrated population model, which combines multiple sources of data and can provide increased precision over models that only consider population counts (Schaub and Abadi, 2011; Kéry and Schaub, 2012; Coates and others, 2019), to estimate over the two most recent nadir-to-nadir cycles. Nadir-to-nadir cycles differed slightly for the nadirs estimated using the integrated population model (IPM): short-term (2001–21) and recent-term (2008–21).
We used SSMs (Royle and Dorazio, 2009; Kéry and Schaub, 2012) within a Bayesian hierarchical framework to estimate at each lek from 1969 to 2019. State-space models separate variance among the observation process and the state process, thus accounting for observation error (such as, imperfect detection). In addition, SSMs are well-suited for modeling time series data (Kéry and Schaub, 2012; Aeberhard and others, 2018) and have previously been used to model range-wide population trends of sage-grouse (Coates and others, 2021). We modeled the number of males per lek (l) and time (t) as a function of the number of males the prior year and the intrinsic rate of change (r) using the following equations (eqs. 3–10):
whereis the estimated abundance at lek l in time t,
is the estimated intrinsic rate of change at lek l in time t,
is the estimated intrinsic rate of change at subpopulation i in time t,
is the standard deviation for ,
is the estimated intrinsic rate of change for the Bi-State DPS in time t,
is the standard deviation for ,
is the standard deviation for , and
is the mean hyperparameter for .
yl,t
is the observed count data at lek l in time t, and
is the estimated abundance at lek l in time t.
Estimated abundance () for subpopulation i in time t was calculated as the sum of at all leks within subpopulation i in time t after correcting for lek detection (probability of 0.95), average detection bias in sightability (probability of 0.86; Coates and others, 2019) and average fluctuations in attendance by males (probability of 0.85; Wann and others, 2019). In addition, we included a correction on lek counts to estimate the number of females in the population using sex ratios (; Braun and others, 2015), which ultimately provided an estimate of the total abundance per subpopulation (Coates and others, 2024b). We also summed the annual abundance estimates across subpopulations to calculate an annual estimate of across the Bi-State DPS and estimate regional . We summarized all posterior distributions for parameters using the median and 95-percent CRI. We used the “rjags” package (Plummer, 2016) to fit the SSM. We ran the model on three chains of 100,000 iterations each, discarded the first 50,000 iterations from each chain as burn-in, and used a thinning rate set to 10, retaining 15,000 posterior samples for all parameters monitored. We evaluated chain convergence based on a potential scale reduction factor value less than 1.2 (Gelman and Rubin, 1992; Brooks and Gelman, 1998).
For nadir-to-nadir cycles for which we had consistent demographic data, we used an IPM within a Bayesian hierarchical framework, which combined multiple sources of data, including both lek counts and annual demographic data from individually marked birds, to estimate (Schaub and Abadi, 2011; Kéry and Schaub, 2012; Coates and others, 2019). Integrated population models have previously been used in the Bi-State DPS to evaluate population trends and the effects of climate on population processes (Coates and others, 2018, 2019). Within the IPM, the state process was structured as a stochastic demographic matrix model, which included two age classes (yearling and adult) and subcomponent models for individual vital rates (for example, annual survival and recruitment). Lek counts informed the observation process of the IPM and we generated estimates of the finite rate of population growth using a joint likelihood reflecting annual survival and recruitment. Our modeling framework also allowed for the inclusion of random effects for subpopulation and year, which further refined and facilitated variability in the demographic process models over space and time.
We separated the demographic process into annual survival and fecundity, which in turn were divided into multiple subcomponents (Coates and others, 2019). We assumed that migration among subpopulations was negligible (O’Donnell and others, 2022), but sage-grouse frequently move among leks within a subpopulation. Therefore, we assumed that leks within the same subpopulation shared similar demographic rates.
We estimated adult and yearling survival using frailty models (Halstead and others, 2012), wherein we assumed a constant hazard rate and used discrete monthly intervals created from live-dead encounter histories. Individuals with unknown final fates were right-censored, and sage-grouse were graduated to the next age class if they were alive through the month of March of the subsequent year. Monthly unit hazards (UH, eq. 12) were specified using the following model structure:
whereis the monthly unit hazard for age a, individual h, subpopulation i, year j, and month k,
is the baseline intercept,
is the age-specific log hazard ratio,
, , and
are subpopulation, year, and subpopulation-by-year log hazard ratios, respectively, and
, , and
are standard deviation hyperparameters for the subpopulation, year, and subpopulation-by-year log hazard ratios, respectively.
Following the modeling process, we derived the annual survival parameter (s; eq. 18) specific to age class, subpopulation, and year using the following equations:
whereis annual survival for age a, subpopulation i, and year j,
is the cumulative hazard calculated where T=12 months, and
is the monthly hazard for age a, subpopulation i, year j, and month k.
Inferences of survival models were made from VHF-marked sage-grouse due to reduced survival probabilities of GPS-marked sage-grouse (Severson and others, 2019). Juvenile sage-grouse (post-fledging, greater than 35 days old and less than 1 year old) were radio-marked in the fall, and we modeled juvenile survival separately following the same approach for adults and yearlings, less the age effect.
Telemetry data were insufficient to estimate parameters for nesting propensity, so we used estimates from Taylor and others (2012) of 0.96 (95-percent confidence interval [CI]: 0.94,0.97) and 0.89 (95-percent CI: 0.87,0.91) as informative priors for adults and yearlings, respectively. To estimate clutch size of first (cl1) and second (cl2) nests, we used a log-linear model. We assumed clutch size arose from a Poisson distribution (eq. 20) taking the form:
whereis observed clutch size for age a, clutch number c, individual h, subpopulation i, and year j,
μcl
mean clutch size,
is the baseline intercept,
is the fixed effect of nest attempt,
is the fixed effect of hen age,
, , and
are random effects for subpopulation, year, and subpopulation-by-year, respectively,
, , and
are standard deviation hyperparameters for the random effects of subpopulation, year, and subpopulation-by-year, respectively.
Thus, the log of the expected clutch size (μcl) was a linear combination of nest attempt (βattempt), age (βage), subpopulation (αi), year (γj), and subpopulation-by-year () effects, with intercept (eqs. 20–27). Subscript c represented clutch number (that is, first or second), and all other subscripts followed the adult survival equations above (eqs. 12–17).
Nest survival for first (ns1) and second (ns2) nests was modeled using the frailty models described above for adult survival (eqs. 12–19) at discrete daily intervals (t=1,2, …, T) to estimate cumulative survival (T=38) across both the laying and incubation phases. We included a fixed effect for nest attempt and an additional random effect for individual hen to account for individuals with multiple nests within or across seasons.
Egg hatchability (hatch) was modeled with data from successful nests (one or more egg hatched) using a binomial distribution (eq. 28) that took the following form:
whereis the number of successfully hatched eggs for age a, individual h, subpopulation i, and year j,
is the probability of an egg hatching successfully,
is the initial number of eggs in a clutch,
is the baseline intercept,
is the fixed effect of hen age,
, , and
are random effects for subpopulation, year, and subpopulation-by-year, respectively,
, , and
are standard deviation hyperparameters for the random effects of subpopulation, year, and subpopulation-by-year, respectively.
Through the logit-link, phatch,ahij was modeled as a linear function of age (), subpopulation (), year (), and subpopulation-by-year () effects, with intercept (eqs. 29–34).
The probability of a second nest attempt (np2) was modeled using a Binomial distribution (eq. 35) and took the form:
whererepresents the number of renests for age a, subpopulation i, and year j;
is the probability of a second nest attempt;
is the number of unsuccessful first nests;
is the baseline intercept;
is the fixed effect of hen age;
, , and
are random effects for subpopulation, year, and subpopulation-by-year, respectively; and
, , and
are standard deviation hyperparameters for the random effects of subpopulation, year, and subpopulation-by-year, respectively.
We derived chick survival (cs) probabilities from two brood counts with time intervals that varied across the study period. The maximum number of days that broods were observed was either 50 days (2003–05, 2012–19), 35 days (2007–09, 2021–22), or 28 days (2010–11). To account for these differences, we applied a correction factor to the numerator in the inverse-logit function based on the number of days d in the observation period for year j (). We then modeled chick survival from the brood count data (eq. 42) using the following equation:
whererepresents the number of chicks that survived for each brood, b, for hen age a, individual h, subpopulation i, and year j;
is the probability of a chick surviving the 35-day period;
is the initial number of chicks at hatch;
is the baseline intercept;
is the fixed effect of hen age;
, , and
are random effects for subpopulation, year, and subpopulation-by-year, respectively; and
, , and
are standard deviation hyperparameters for the random effects of subpopulation, year, and subpopulation-by-year, respectively.
Parameter terms and their definitions (eqs. 43–48) follow the same structure as for second nest propensity. The initial brood size at hatch served as the number of trials and the number of chicks that survived to the end of the observation period represented the number of successes.
We defined recruitment as the number of females added to the population on an annual time step from reproduction. For each age class a, year j, and subpopulation i, we multiplied the probabilities of all the demographic subcomponent models and multiplied that result by 0.5, assuming a balanced male:female ratio to estimate the number of females recruited into the population the following breeding season. Thus, recruitment (Raij; eq. 49) was specified using the following equation:
whererepresents recruitment for hen age a, subpopulation i, and year j,
is first nest propensity,
is clutch size for first nests,
is nest survival for first nests,
is hatchability,
is chick survival,
is juvenile survival,
is second nest propensity,
is clutch size for second nests, and
is nest survival for second nests.
To restore a critically small subpopulation (A-006), both male and female sage-grouse were translocated from 2017 to 2022, with all translocated grouse explicitly accounted for in the IPM. We estimated separate retention rates for translocated males and females by calculating the proportion of individuals that were present at the release site greater than 30 days after translocation. Within the IPM, males and females were removed from the capture subpopulation and added to the release subpopulation, with an associated probability of retention, in the state process functions (Kéry and Schaub, 2012). Translocated males were only included in subsequent lek counts if they remained and joined the subpopulation.
Within the IPM, an underlying SSM (Kéry and Schaub, 2012) estimated observation error using the observed lek count time series data (see the “Field Methods” section above) coupled with latent (estimated) total population size and annual population rates of change (). We applied the same corrections for sightability, lek attendance, and sex ratios described for the SSM above, which ultimately provided an estimate of the total abundance per subpopulation. Lek counts (y) informed latent abundance estimates (eqs. 50–51) using the following equation:
whereis the observed count data at lek l, subpopulation i, and year j,
is the estimated abundance, and
is the standard deviation hyperparameter for estimated abundance.
We then used joint likelihoods, where lek counts informed the observation process and demographic data informed the state process (Kéry and Schaub, 2012; Coates and others, 201832). Including random effects allowed us to estimate abundance () through time and across subpopulations, which were used to derive the estimated population rate of change, , (eq. 52) using the following form:
whereis the estimated population rate of change for subpopulation i and year j, and
is the estimated abundance of subpopulation i in year j.
Similar to the SSM described above, we summed the annual abundance estimates across the subpopulations to calculate an annual estimate of across the Bi-State DPS and estimate regional . We summarized all posterior distributions for parameters using the median and 95-percent CRI. We used the “rjags” package (Plummer, 2016) to fit the IPM. We ran the model on three chains of 100,000 iterations each, discarded the first 50,000 iterations from each chain as burn-in, and used a thinning rate set to 10, retaining 15,000 posterior samples for all parameters monitored. We evaluated chain convergence based on a potential scale reduction factor value less than 1.2 (Gelman and Rubin, 1992; Brooks and Gelman, 1998).
Distributional Areas and Modeling Changes in Sage-Grouse Distribution
Using standardized kernel point density models (Doherty and others, 2016), we created a probability density function across the Bi-State DPS using lek locations. Kernel estimators are appropriate for this type of analysis because they are non-parametric analyses that allow probabilistic inference of animal utilization based on density of geographic point locations. Although location data from individual animals are typically used to approximate probability density functions, point locations of breeding sites, such as leks, can provide an index of population-level density and distribution when abundance data are associated with each lek (Coates and others, 2016a; Doherty and others, 2016). Because we were interested in broad-scale population distributions, we estimated two probability density functions using separate bandwidths (Gitzen and others, 2006), specifically 6.4 and 10.6 kilometers (km), which represented estimates of breeding season and year-round population-level distribution patterns of radio-marked sage-grouse relative to lek sites within the Bi-State DPS (Coates and others, 2013). We compiled median for each lek in each year using estimates from the SSM described above (Coates and others, 2024b). We weighted each lek location by its corresponding median for each year, allowing for variation in spatial distribution based on variation in abundance among leks over time. The two probability density surfaces were then standardized and averaged for each pixel to account for both breeding areas and other seasonally used areas.
To estimate boundaries of core and overall sage-grouse distribution, we calculated 50-percent and 99-percent isopleths of the probability density function, respectively. We then removed non-habitat areas within these isopleths by intersecting them with areas of sage-grouse habitat defined in the “Objective 1” section to minimize inclusion of landcover types rarely used by sage-grouse (for example, salt flats, open water). We used the annual composite selection layer to represent habitat and considered non-habitat to be anything below the 5th percentile of values at all used locations (see the “Objective 1” section for full details). We calculated separate habitat layers for each population nadir estimated using the SSM to capture changes in habitat across time back to 1995, which was the earliest nadir for which time-varying remotely sensed layers were available. The resulting distributional layers created by intersecting the isopleths with the habitat layer represent occupied sage-grouse habitat during each time period and were scaled from 0 to 1. We then calculated two metrics of spatial distribution, the total area and proportional volume of the distributional raster, within each subpopulation, as well as the Bi-State DPS as a whole, in each year. We calculated area as the number of pixels in the resulting raster layers multiplied by the size of the pixel, and we calculated proportional volume for each year by dividing the raster representing area by the sum of all the pixels in that same raster, which incorporates the height of the distributional layer in addition to the spatial area. To validate the distributional areas, we calculated the proportion of modeled leks (94.8 percent) that fell within the 95-percent isopleth, indicating that sage-grouse space use patterns in this region continued to meet the assumptions used to generate distributional areas.
To evaluate changes in sage-grouse distribution across the study period (1995–2019) for each subpopulation and the Bi-State DPS as a whole, we used linear mixed models in a Bayesian framework. We analyzed subpopulation-specific trends in total occupied area on a common scale by standardizing the annual area for each subpopulation around its mean and standard deviation for the series. This allowed trend estimates to be comparable across subpopulations. Proportional volume did not require standardization because it was bounded between zero and one. By including both random intercepts, , and slopes, , for each subpopulation i, we allowed trends over time to vary across subpopulations. We estimated the trend using the marginal distribution of the time effect for each subpopulation, where a negative or positive sign would indicate a declining or increasing trend, respectively. We assumed random intercepts and slopes arose from Normal distributions with means of and and variances of and corresponding to subpopulation intercept and slope, respectively. For inferences at the Bi-State DPS-wide scale, we used the hyperparameters of the random slopes and we used differences in distribution between the initial and final years to estimate net gain and loss for each subpopulation and the Bi-State DPS as a whole. We report estimates of net gain/loss (km2), rate of gain/loss (), and probability (P) of for each subpopulation and the Bi-State DPS in each time period based on predicted posterior distributions. To evaluate if distributional trends deviated from abundance trends, we compared trends in both area and proportional volume for each subpopulation to trends in abundance based on estimates from the SSM (see the “Methods: Population Modeling and Estimated Abundance” section).
Results–Objective 2
Results: Population Modeling and Estimated Abundance
We compiled 1,720 counts from 58 leks across the Bi-State DPS to inform the observation processes of the SSM and IPM. To inform the state process of the IPM, we compiled individual-based life history data from 698 marked sage-grouse. Sample sizes varied for the estimation of specific vital rates (survival: n=698, nest survival: n=715, clutch size: n=494, hatchability: n=361, second nest propensity: n=283, chick survival: n=349).
Using an SSM, we evaluated changes in population abundance over six different temporal scales encompassing six complete population cycles: long-term (1969–2019), medium/long-term (1978–2019), medium-term (1983–2019), short/medium-term (1995–2019), short-term (2002–19), and recent-term (2008–19). Based on estimates from the state-space model the Bi-State DPS demonstrated evidence of declines of ~1.2–2.5 percent annually over the long-term (=0.979, 95-percent CRI: 0.968, 0.987), medium/long-term (=0.977, 95-percent CRI: 0.963, 0.987), medium-term (=0.985, 95-percent CRI: 0.969, 0.995), short/medium-term (=0.987, 95-percent CRI: 0.970, 0.999), short-term (=0.975, 95-percent CRI: 0.963, 0.985), and recent-term (=0.988, 95-percent CRI: 0.973, 1.001), which translated to estimated declines of 65.9 percent since the nadir in 1969, 62.0 percent since the nadir in 1978, 42.7 percent since the nadir in 1983, 26.7 percent since the nadir in 1995, 34.5 percent since the nadir in 2002, and 12.9 percent since the nadir in 2008 (table 6). Estimated trends over the short-term (=0.980, 95-percent CRI: 0.971, 0.990) and recent-term (=0.985, 95-percent CRI: 0.973, 0.997) from the integrated population model were similar to estimates from the SSM above and previous studies (fig. 10; Coates and others, 2021), although there were slight differences in the estimated nadir years and credible intervals were smaller due to the inclusion of demographic data. After accounting for variation in male lek attendance, sightability, and sex ratios, estimated population abundance across the Bi-State DPS from the SSM was 7,480 (95-percent CRI: 4707,13168) in 1969, 6,707 (95-percent CRI: 4214,12502) in 1978, 4,456 (95-percent CRI: 2841,8258) in 1983, 3,482 (95-percent CRI: 2414,5427) in 1995, 3,898 (95-percent CRI: 2955,5232) in 2002, 2,929 (95-percent CRI: 2261,3742) in 2008, and 2,551 (95-percent CRI: 1984,3194) in 2019.
Table 6.
Model-derived estimates of population rates of change () from the state-space model with 95-percent credible intervals for each subpopulation over the long-term (1969–2018), medium/long-term (1978–2018), medium-term (1983–2018), short/medium-term (1995–2018), short-term (2002–18), and recent-term (2008–18) for greater sage-grouse in the Bi-State Distinct Population Segment.[PMU, population management units; %, percent; CRI, credible intervals]
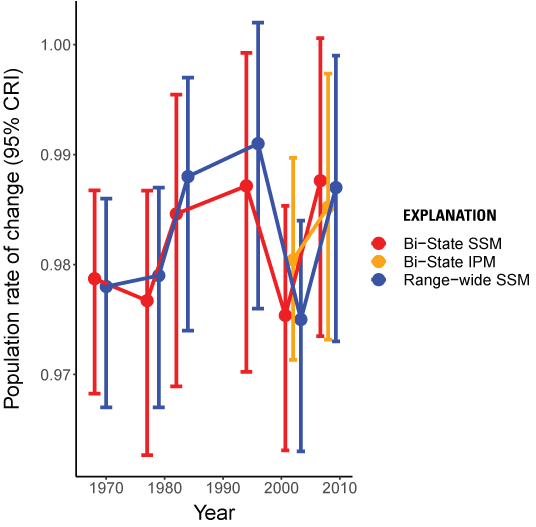
Estimated population rate of change with 95-percent (%) credible intervals (CRI) estimated for each population cycle from the Bi-State state-space model (SSM) and Bi-State integrated population model (IPM) presented here and the previously published range-wide SSM (Coates and others, 2023). Years represent the start of a population cycle, with the population rate of change for each cycle measured through 2018 for the SSMs and 2020 for the IPM based on the nadirs estimated by each model. Collection of demographic data began in 2003, so estimates for the IPM are only presented for the two most recent population cycles.
Most of the Bi-State DPS was concentrated in three subpopulations (A-003, A-004, and A-007), considered core subpopulations, and the remaining subpopulations were considered peripheral subpopulations (fig. 1B). Trends in abundance based on the SSM varied by subpopulation (table 6). However, only one core (A-003) and one peripheral subpopulation (A-002) had positive population growth over the medium- and short/medium-term, and one core (A-004) and one peripheral population (A-005) had positive population growth over the recent-term (table 6). All other subpopulations demonstrated evidence of negative population growth across all time periods, although credible intervals did overlap one for some subpopulations in some time periods (table 6). However, some subpopulations, particularly the subpopulation in the White Mountains (A-001), likely contain unknown leks and are difficult to access during the lekking season, which could affect estimates if trends at unknown leks or at leks lacking sufficient data due to access differ from trends at modeled leks. Declines were substantial in some subpopulations, with 5 subpopulations declining more than 50 percent and 3 peripheral subpopulations (A-001, A-006, and A-011) declining more than 75 percent since 1995.
Distributional Areas and Changes in Sage-Grouse Distribution
The distribution of occupied sage-grouse habitat declined in the Bi-State DPS (fig. 11) and shifted among subpopulations over 25 years and 3 population cycles. Whereas the area of two core subpopulations (A-003 and A-004) and one peripheral subpopulation (A-002) and the volume of one core subpopulation (A-003) increased, the area and volume of six and eight subpopulations, respectively, decreased, with the evidence for increasing or decreasing area and volume strong across most subpopulations (tables 7, 8; figs. 12, 13). Annual rates of loss of area and volume were most substantial in one core subpopulation (A-007; figs. 12, 13; tables 7, 8) and three peripheral subpopulations (A-005, A-006, and A-009). Gains in three subpopulations (fig. 12) helped offset losses in the other subpopulations over time, but population-level trends for the Bi-State DPS were negative for both area (β= −0.02; 95-percent CRI: −0.08, 0.04) and volume (β= −0.03; 95-percent CRI: −0.06, 0.002) with 77- and 97-percent probabilities of contraction in area and volume, respectively. Overall, even though there was not strong evidence for a decline in area due to variation in trends among subpopulations, we estimated the Bi-State DPS lost ~156 km2 of occupied area between 1995 and 2019.
Table 7.
Model-derived estimates of the rate of gain in overall area (β), the probability that the rate of change is different from zero, estimated net change (km2), and the proportion of the overall area occupied in 1995 that changed for subpopulations of greater sage-grouse in the Bi-State Distinct Population Segment from 1995 to 2019.[Negative values represent a net loss for a subpopulation. Abbreviations: %, percent; CRI, credible intervals; >, greater than; km2, square kilometers]
Table 8.
Model-derived estimates of the rate of change in volume (β), the probability that the rate of change is different from zero, and the proportion of the volume occupied in 1995 that changed for subpopulations of greater sage-grouse in the Bi-State Distinct Population Segment from 1995 to 2019.[Negative values represent a net loss for a subpopulation. Abbreviations: %, percent; CRI, credible intervals; > greater than]
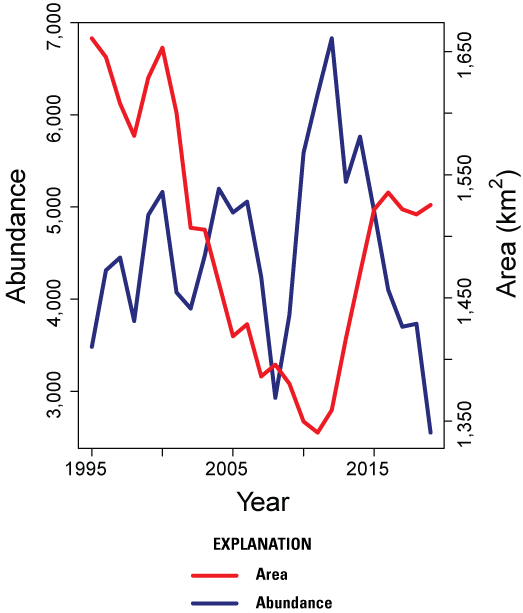
Trends in abundance (blue, left axis) and distribution (red, right axis) for greater sage-grouse in the Bi-State Distinct Population Segment from 1995 to 2019. Estimated abundance was based on a state-space model and credible intervals are omitted for clarity. Area shown in square kilometers (km2).
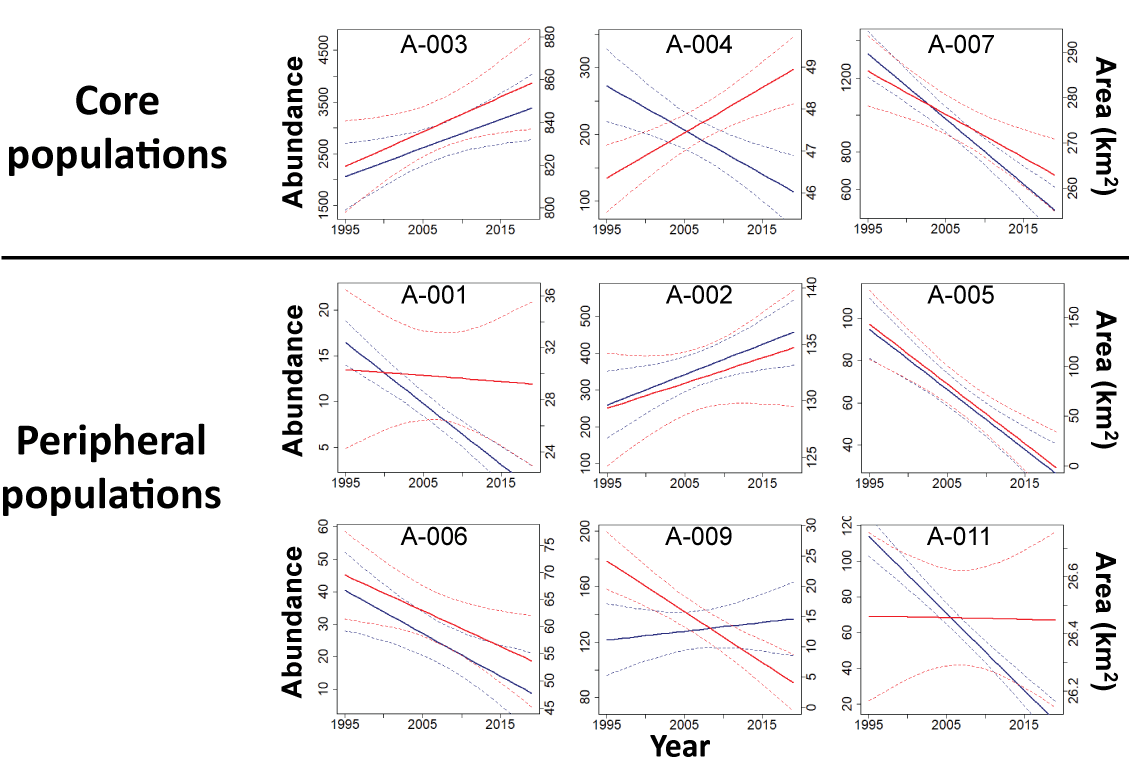
Changes in abundance (blue, left axis) and overall area (red, right axis) for three core subpopulations (top) and six peripheral subpopulations (bottom) of greater sage-grouse in the Bi-State Distinct Population Segment from 1995 to 2019. Estimated abundance was based on a state-space model and area was calculated as the 99-percent isopleth. Dashed lines represent 95-percent credible intervals.
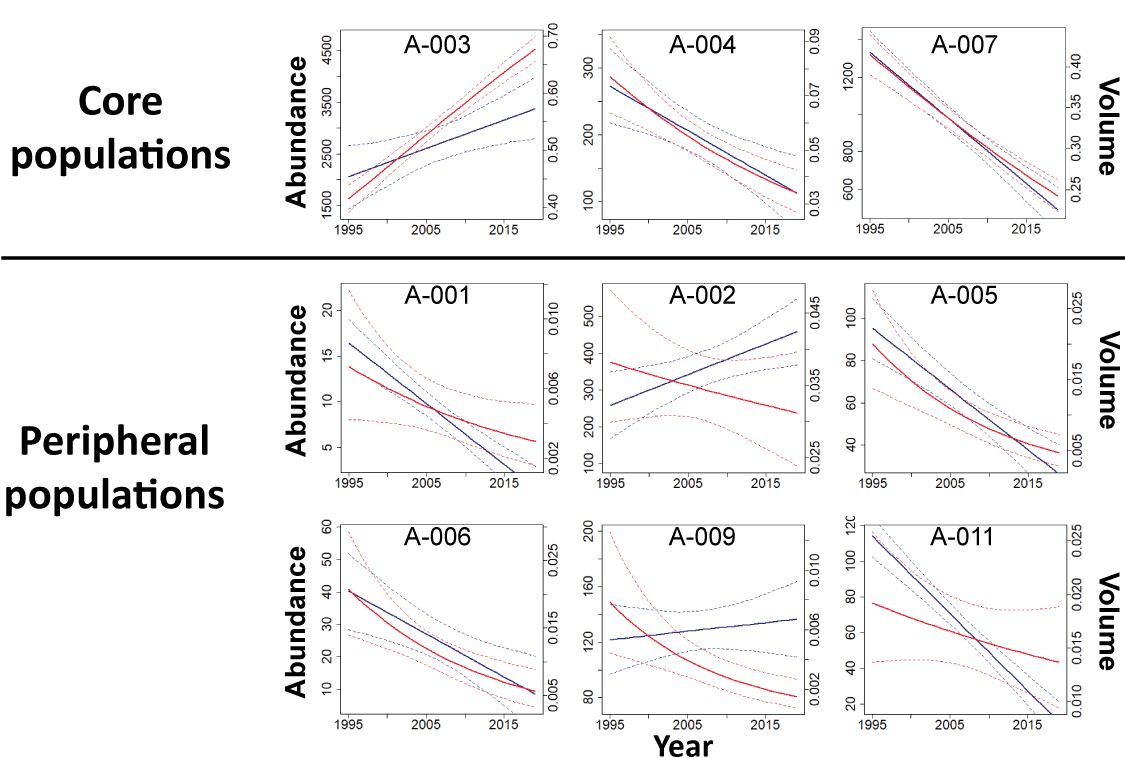
Changes in abundance (blue, left axis) and volume (red, right axis) for three core subpopulations (top) and six peripheral subpopulations (bottom) of greater sage-grouse in the Bi-State Distinct Population Segment from 1995 to 2019. Estimated abundance was based on a state-space model and volume was calculated by dividing the raster representing area by the sum of all the pixels in that same raster. Dashed lines represent 95-percent credible intervals.
The core distributional area (that is, the 50-percent isopleth) was comprised of three subpopulations (A-003, A-004, and A-007) over the 25-year period. During that time, two subpopulations (A-004 and A-007) contracted whereas the third (A-003) expanded (table 9; fig. 14). There was no significant change in the overall core distribution (β= −0.02; 95-percent CRI: −0.91, 0.96, P(|β|>0)=0.57), although there was high uncertainty.
Table 9.
Model-derived estimates of the rate of change in core area based on the 50-percent isopleth (β), the probability that the rate of change is different from zero, estimated net change (square kilometers), and the proportion of the core area occupied in 1995 that changed for subpopulations of greater sage-grouse in the Bi-State Distinct Population Segment from 1995 to 2019.[Negative values represent a net loss for a subpopulation and two subpopulations did not have any core area estimated in 1995. Abbreviations: %, percent; CRI, credible intervals; >, greater than; km2, square kilometers]
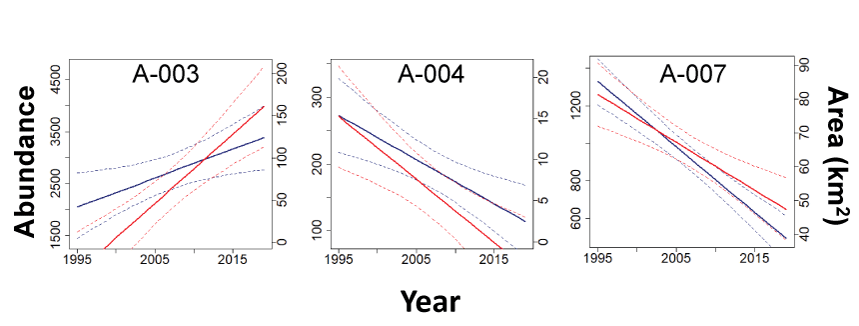
Changes in abundance (blue, left axis) and area (red, right axis) for three core subpopulations of greater sage-grouse in the Bi-State Distinct Population Segment from 1995 to 2019. Estimated abundance was based on a state-space model and area for core subpopulations was calculated as the 50-percent isopleth. Dashed lines represent 95-percent credible intervals.
The direction of trends in distribution and abundance were consistent for five subpopulations but diverged for four subpopulations (fig. 12). Increases in area and volume were typically greater in populations that were also experiencing increases in abundances, whereas decreases in population abundance were greater or similar in populations experiencing decreases in area and volume. Overall, this indicates important variation among subpopulations, with increases in both population abundance and distribution in expanding subpopulations generally being insufficient to offset decreases in subpopulations declining in both abundance and distribution.
Objective 3. Evaluate Efficacy of Ongoing Conservation Actions Targeting Sage-Grouse within the Bi-State Distinct Population Segment
Purpose
Evaluation of conservation efforts requires effective monitoring and analytical frameworks to assess those actions in relation to population viability. However, quantifying conservation efficacy is often difficult because ecosystem processes are complex and dynamic, and there are often practical limitations to assessing target species’ population dynamics. To improve sage-grouse population growth within the Bi-State DPS and prevent a potential ESA listing, a team of stakeholders including state and federal agencies, academic researchers, nongovernmental organizations, local tribes, private landowners, and the public collectively developed a comprehensive conservation plan for the Bi-State region (Bi-State Technical Advisory Committee, 2012). The action plan identified a suite of conservation actions to be taken, targeting improved population performance of the Bi-State DPS, and mandated long-term monitoring and empirical evaluation of management action effectiveness. We developed a monitoring approach that allows for broad-scale, long-term quantification of the effects of conservation actions on sage-grouse population dynamics and trends within the Bi-State region. We used information from a newly developed Conservation Efforts Database (CED v4.0.0 https://conservationefforts.org/sgce/home/; U.S. Geological Survey, 2023), which compiles and maintains records of conservation actions taking place within sagebrush ecosystems, with lek count data (O’Donnell and others, 2021) and population trend estimation (Coates and others, 2021) of sage-grouse. We used a before-after-control-impact (BACI) analytical framework to evaluate how each conservation action implemented within the Bi-State region has affected sage-grouse population trends since 1990.
Methods
Conservation Efforts Database
The USFWS, USGS, and the Great Northern Landscape Conservation Cooperative (GNLCC) developed the CED (U.S. Geological Survey, 2023) to track conservation efforts for sage-grouse across broad regions managed by multiple entities (public and private). To that end, the CED includes conservation efforts carried out from 1960 to present (2024) across the range of sage-grouse and is continuously updated as additional conservation efforts are implemented. Advantageously, the CED facilitates various agencies or organizations to enter data records of conservation actions, as well as supporting documents or reports, to create a centralized, comprehensive database of conservation efforts undertaken within the sagebrush ecosystem.
We queried the CED and generated summaries of all conservation actions and treatments completed on public and private lands within the Bi-State region between 2012 and 2019. These years were chosen because they aligned with years of robust lek count data collection, which included periods before and after efforts were implemented. We recorded information about the type of conservation effort, the year the effort was implemented, the size of the treatment area, and method of treatment if applicable (for example, mechanical versus chemical removal of invasive weeds). We considered the following conservation efforts in the Bi-State region and recorded in the CED: sagebrush restoration, fence modification (fence removal, fence letdown, or fence marking), road closure, conifer removal, invasive weed reduction, and wet meadow restoration (fig. 1C; table 10). We excluded some conservation efforts (CEs) that are included in the CED (for example, energy, fire, and herd management), due to inadequate sample size or lack of details about the specific treatment performed.
Table 10.
The average size and standard deviation (in parentheses) of areas formed from conservation effort (CE) polygons and 5-kilometer lek (traditional breeding grounds) buffers within the Bi-State Distinct Population Segment of greater sage-grouse.[All CEs took place between 2012 and 2020 and were associated with at least three or more active leks. Abbreviation: ha, hectares]
Modeling Rates of Population Change in Abundance
We obtained Bi-State DPS lek count data spanning 1990–2021 following the same procedures described for the “Objective 2” section. We retained observations that were recorded during the season of peak lek attendance (March 1 to May 31; Emmons and Braun, 1984; Wann and others, 2019), carried out within 30 minutes before and 90 minutes after local sunrise (Jenni and Hartzler, 1978) and were collected using one of the following standardized survey methods: ground-based lek counts, aerial helicopter-based counts, aerial fixed-wing aircraft-based counts, aerial helicopter-mounted HD/IR camera counts, aerial fixed-wing aircraft-mounted HD/IR camera counts, unknown aerial based counts, ground-based lek count routes, and unknown ground-based counts. We assumed counts with missing date, time, or survey methods were done within daily and seasonal windows and followed an approved survey method. We only included the maximum count for each year when multiple, within-year counts were done. Finally, we did not include leks that were considered active (greater than or equal to two males present/visit) in fewer than 5 years during the inferential period (1990–2021), based on findings from Coates and others (2020) that sporadic time series data can generate biased estimates of the intrinsic rate of population change ().
We used the software package R (R Core Team, 2022) with the JAGS library utilizing the “rjags” package (Plummer, 2016) to fit a SSM on the filtered lek count data. State-space models produce estimates of that represent trends in the sampled population when count data are collected with imperfect, but relatively constant detection rates across time (Kéry and Schaub, 2012; Monroe and others, 2019). Additionally, SSMs consist of a set of linked likelihoods, which allows for parameters to be fit according to their effect on process (that is, environmental) variance or observation error (Kéry and Schaub, 2012). The process component of our model (eq. 53) incorporated spatiotemporal relationships that linked population dynamics across fine (individual lek), intermediate (neighborhood; a cluster of leks), and coarse (Bi-State DPS) spatial scales (O’Donnell and others, 2019; Coates and others, 202130):
whereis the estimated population size at lek l in time t, and
is the estimated intrinsic rate of change at lek l in time t.
Here represents the latent state (that is, population size) of lek l in year t, and the intrinsic rate of change in abundance (), describes changes in log-population size from one year to the next (that is, from t to t+1). We used an exponential growth model, which uses a log-scale for abundance and rate of change parameters (Lande and others, 2003) because of the stochasticity of sage-grouse population dynamics (Moynahan and others, 2007; Fedy and Aldridge, 2011; Fedy and Doherty, 2011; Dinkins and others, 2016). We modeled as realizations of a normally distributed random process where population dynamics were propagated across progressively larger spatial extents:
whereis the estimated intrinsic rate of change at lek l in time t,
is the estimated intrinsic rate of change for intermediate population level n,
is the standard deviation for lek l,
is the estimated intrinsic rate of change for the Bi-State DPS,
is the standard deviation for intermediate population level n,
is the mean hyperparameter for the Bi-State DPS, and
is the standard deviation hyperparameter for the Bi-State DPS.
The mean () represents the annual rate of change within intermediate population levels (neighborhood n) within which each lek (l) is spatially nested. The standard deviation () quantifies inter-annual, environmental variability affecting lek-level rates of change. We chose a weakly informative prior to represent (eq. 55). Intrinsic growth rates within intermediate population levels () were modeled as normally distributed realizations with mean () and standard deviation () parameters representing central tendencies of the Bi-State DPS (c) and inter-annual, environmental variability at the intermediate population level, respectively. Bi-State DPS rates of change were described using a similar specification, but with the mean () parameter representing the long-term average intrinsic rate of population change of the Bi-State DPS. We assigned a vague, uniform prior for (eq. 60), which allows for percentage changes ranging from approximately −10 to 10 percent, which is beyond estimates reported for long-term sage-grouse population performance range-wide (Garton and others, 2011), and within the Bi-State DPS (Coates and others, 2018).The final parameter within our model () represented a measure of inter-annual, environmental variability affecting population rates of change within the distributional extent of the species’ range within the United States (Coates and others, 2021).
The observation process of our model mapped the latent state of abundance onto the observed data (), which were maximum counts of male sage-grouse (y) attending leks (l) in each year (t; eq. 61).
whereis the observed count data at lek l in time t, and
is the estimated population size at lek l in time t.
Counts were modeled using a Poisson distribution, which assumes increasing variance with increasing individuals counted. We specified a vague prior with lower and upper bounds of 2 and 500, respectively, to describe initial populations for each lek (l) during year one (t=1) (eq. 62).
We ran the model on three chains of 100,000 iterations each, discarded the first 50,000 iterations from each chain as burn-in, and used a thinning rate set to 50, retaining 3,000 posterior samples for all parameters monitored. We evaluated chain convergence based on a potential scale reduction factor value less than 1.2 (Gelman and Rubin, 1992; Brooks and Gelman, 1998).
Evaluating Conservation Action Effectiveness
We used cross-scale comparisons of sage-grouse population performance before and after treatments were applied (BACI study design) to evaluate the effectiveness of CEs. Using the posterior distributions of abundance from the SSM as a performance metric, we partitioned that index into one of two treatment (control or impact) and period (before or after) categories. We split treatment periods at the year in which the CE occurred. Leks were assigned to the impact group if they were within 5 km (Coates and others, 2013) of at least one CE and were considered active (recording greater than two males during at least one count) during at least 1 year during the post-treatment period. By requiring that leks remained active for at least 1 post-treatment year, we ensured that CEs analyzed here had the opportunity to affect population performance. Lek count data were collected during the spring, so we assigned abundance estimates from the same year as the CE to the period before implementation because it was likely that CE implementation occurred after those counts. We lacked a conventional control and so used all leks within the DPS that were not within 5 km of the CE being assessed as the spatial reference. Finally, we only considered CEs that were implemented within a 5-km radius of greater than or equal to three leks. The number of leks used to evaluate the effectiveness of specific CEs are provided in table 10.
We incorporated a paired-series component (BACIPS) into the traditional BACI methodology that accounted for time-dependent changes in our response variable. Both traditional BACI and BACIPS analyses assume that the system being measured responds in an immediate and constant manner. This assumption is observed during steps that reduce the temporal variation of the time series (Thiault and others, 2017) into four categories (before-control, before-impact, after-control, after-impact). Although this may be appropriate in some cases, the effect of CEs on sage-grouse population dynamics is expected to be more complex, with lagged and potentially non-linear abundance responses. The PC BACIPS framework (Thiault and others, 2017) uses multi-model inference to evaluate alternative functional relationships for the relativized BACI effect. We used PC BACIPS methods to investigate whether CEs implemented within the Bi-State region had effects on sage-grouse populations that were either (1) immediate and constant (step change; classic BACIPS model), (2) linear (the difference between control and affected area changes at a constant rate through time), (3) asymptotic (the rate of change monotonically decreases to zero as time passes), or (4) sigmoidal (the rate of change initially increases over time but then decreases to zero). Conservation efforts were implemented at different times for different leks, but our interest was in the general effectiveness of CEs rather than on any one population’s (that is, lek) response. Therefore, we standardized lek-level time series such that CE effectiveness was measured in years since CE implementation. We first used a standard BACIPS analysis applied to posterior parameter distributions (Conner and others, 2016) for each lek but did not average over the post-treatment intervals. We calculated R-ratios of abundance during the pre-treatment period that represented long-term (9-year) averages from immediately before CE implementation (eq. 63):
whereis the R-ratio of abundance during the pre-treatment period,
is the estimated population size during the pre-treatment period at leks where conservation efforts were implemented, and
is the estimated population size during the pre-treatment period at control leks.
However, we calculated R-ratios of abundance during the post-treatment period using an annual form (eq. 64):
whereis the R-ratio of abundance during the post-treatment period in a given year,
is the estimated population size during the post-treatment period at leks where conservation efforts were implemented in a given year, and
is the estimated population size during the post-treatment period at control leks in a given year.
is the BACIPS ratio,
is the R-ratio of abundance during the post-treatment period in a given year, and
is the R-ratio of abundance during the pre-treatment period.
We then standardized the temporal indices of the BACIPS ratios by subtracting the year of CE implementation, which allowed us to aggregate lek-level BACIPS ratios using intra-interval averages. The PC BACIPS analysis requires a complete time series including pre- and post-treatment periods for each treatment (impact and control), so we decomposed the time-standardized BACIPS ratios into the individual time series components. For the control group, we set the time series (9 years before and 9 years after) to a fixed value equaling one for simplicity and we assigned the same value to the before period for the impact time series. As a result, we could use the time-standardized BACIPS ratios as the after-impact time series because all other denominators had been cancelled out. To include the uncertainty in the SSM outputs (that is, posterior draws of abundance parameters), we iteratively applied the PC BACIPS method to a single set of BACIPS, recording the top-performing model and corresponding parameter estimates during each iteration. We then summed the number of times each model (that is, step, linear, sigmoid, or asymptotic) was scored as the top model and chose the model with the greatest number of overall selections for inference and line fitting. We based inference and line fit on the mean estimate of model parameters from the iterations corresponding to the overall, top-performing model. We also calculated traditional BACI ratios in addition to the PC BACIPS analysis to identify instances where the traditional BACI method underestimated current (2021) and future effects following CE implementation. Finally, to use as much information from the CED as possible, we implemented the same steps as described above but summarized each unique CE independently. This part of the analysis could be used by land managers to prioritize specific types of CEs that could be implemented in the future based on their relative effects on sage-grouse population performance.
Results–Objective 3
We analyzed data from 90 leks monitored within the Bi-State DPS between 1990 and 2021. Of those, 57 (63.3 percent) met all criteria for inclusion in population rate of change analyses, 46 (51.1 percent) had at least one conservation effort occur within 5 km, and 35 (38.9 percent) were active during at least 1 year of the post-treatment period and met all other criteria for inclusion. The number of conservation efforts associated with each lek ranged from 1 to 6 and averaged 2.4 (standard deviation [SD]=1.4), which resulted in a total of 85 unique conservation effort-by-lek data points. The most common conservation effort was removal of conifers, which varied by the type of treatment (hand crew, n=19, mechanical, n=11; table 10). Road closure efforts overlapped with 5-km lek buffers slightly less often (n=28) than conifer efforts with most being classified as seasonal closures (n=22). Invasive weed reduction and sagebrush restoration projects represented nine and eight samples, respectively. Wet meadow restoration and fence modification projects were slightly less common with five instances each. The average size of conservation efforts, in terms of area overlapping 5-km lek buffers, varied among types. Conifer removal projects were the largest (572.8 ha; SD=542.4). However, there was considerable variation in treatment size by conifer removal method (mechanical=57.4 ha, SD=217.3; hand crew=813.3 ha, SD=530.4). Among sagebrush restoration efforts, seeding actions (137.3 ha; SD=207.9) were larger than plantings (12.9 ha; SD=24.2). Wet meadow restoration projects averaged 237.2 ha (SD=70.0), and all other projects were smaller by comparison (table 10).
We determined that sage-grouse population responses over the initial 9 years post-implementation were best described by a linear increase in the BACIPS ratio (fig. 15) when we applied the framework across all CEs (table 11), whereas the step change model (BACIPS ratio=1.68; 95-percent CRI=1.39–2.22) oversimplified sage-grouse response following CEs (fig. 15). We estimated the average annual improvement in the BACIPS ratio to be 13 percent for any CE, based on the line fit from the most parsimonious model (table 12). After 9 years, populations exposed to any combination of CEs had average abundance estimates 121 percent greater than controls (BACIPS ratio=2.21; 95-percent CRI=1.83–2.99). Despite an initially weaker response predicted by the linear model, 9-year post-treatment values were nearly twice the step change estimate (fig. 15). Accounting for population-specific CE-exposure times, we calculated an average annual increase in abundance, relative to leks that did not receive conservation actions, of 4.4 percent, and a cumulative increase of 37.4 percent since 2012.
Table 11.
Model results following iterative application of the Progressive-Change before-after-control-impact paired-series (BACIPS) framework to posterior draws of abundance using control and impact (received conservation effort) populations of greater sage-grouse located within the Bi-State Distinct Population Segment.[Larger proportions correspond to more parsimonious models, which in turn describe the shape of the temporal change in the BACIPS ratio]
Table 12.
Parameter estimates corresponding to the most parsimonious model from application of the Progressive-Change before-after-control-impact paired-series (BACIPS) framework to populations of greater sage-grouse receiving various conservation efforts (CE) within the Bi-State Distinct Population Segment.[The long-term effect of CE implementation is represented by the parameter M for step, asymptotic, and sigmoid models. The parameter B represents the intercept and applies to the asymptotic and sigmoid models. The time required to achieve half of the long-term effect is represented by the parameter L. The scaling parameter K applies to the sigmoid model and affects the steepness of the curve. The parameter I applies to the linear model and is interpreted as the deviation from a BACIPS ratio of one. For linear models, the parameter R represents the constant rate of change. Abbreviation: —, parameter did not apply]
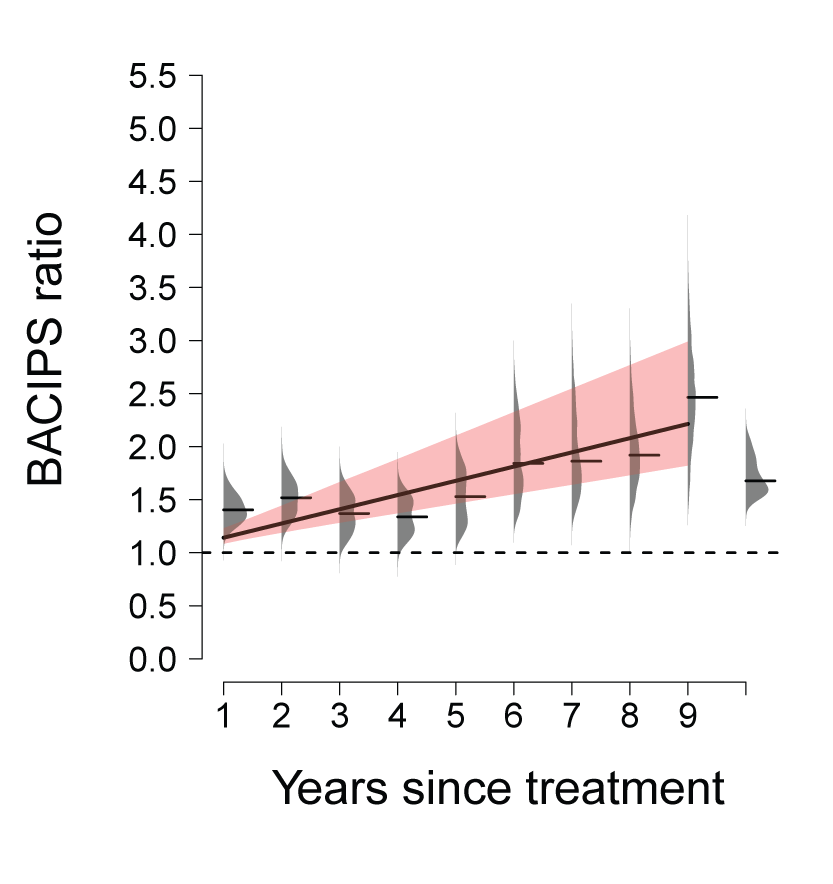
Before-After-Control-Impact Paired-Series (BACIPS) ratios representing changes in greater sage-grouse population abundance () by each lag year and averaged (mean) following application of all conservation efforts (CE) executed within the Bi-State Distinct Population Segment during 2012–21. Posterior distributions (gray) and median ratios (short black lines) were calculated from outputs of Bayesian state-space models based on lek count data. Horizontal dashed line references a BACIPS ratio of one (no difference between control and CE treatment). The solid black line (median) and red polygon (95-percent credible interval) represent predictions from the best fit Progressive Change BACIPS model (linear).
We detected substantial variation in effectiveness among different CEs targeting habitat improvement for sage-grouse in the Bi-State DPS, with effects most commonly providing evidence of a step change. However, several CEs investigated did provide evidence of progressive linear and non-linear effects across time lags since CE implementation (table 8). We determined that support for progressive versus step change models for some CEs varied by application method alone. A step change model that indicated an instantaneous and constant improvement in population abundance of 118 percent (=2.18; 95-percent CRI=1.48–2.79; fig. 16A) best described effects of conifer removal using hand cutting techniques, whereas the effects of mechanical conifer removal provided evidence of a linear increase in population abundance (tables 7, 8). We detected significantly greater relative improvements from mechanical removal of conifers, compared to removal by hand crews, by the ninth post-treatment year (=4.30; 95-percent CRI=2.51–5.97; fig. 16B). For sagebrush restoration CEs, we determined that neither planting nor seeding supported a traditional step-change assumption (table 8). Rather, we documented evidence for a linear response to seeding with predicted maximum annual increases in the BACIPS ratio equal to 12 percent (fig. 16C) and a sigmoidal response to planting with predicted maximum annual increases in BACIPS of 35 percent (fig. 16D). Cumulative responses from planting efforts =3.49; 95-percent CRI=2.23–4.79) yielded a threefold increase over seeding efforts (=1.74; 95-percent CRI=1.29–2.79). Thus, we documented stronger evidence of improvements in population abundance following planting efforts compared to seeding techniques. Based on our analysis, populations adjacent to invasive weed reduction efforts marginally increased, relative to controls, but only when the treatment method was chemical (fig. 16E), whereas mechanical treatments provided strong evidence of a negative step change (tables 7, 8). However, alternative model structures (for example, sigmoid) and positive long-term effects were plausible based on visual inspection of annual BACIPS ratios (fig. 16F) and if the pattern observed during years 4–6 following mechanical treatments continued into the future (for example, during years 7–9). There was strong support for an immediate and constant population response after wet meadow restoration efforts (fig. 16G), with the step change model (table 8) indicating a 110 percent increase (=2.10, 95-percent CRI=1.28–3.17) in population abundance.
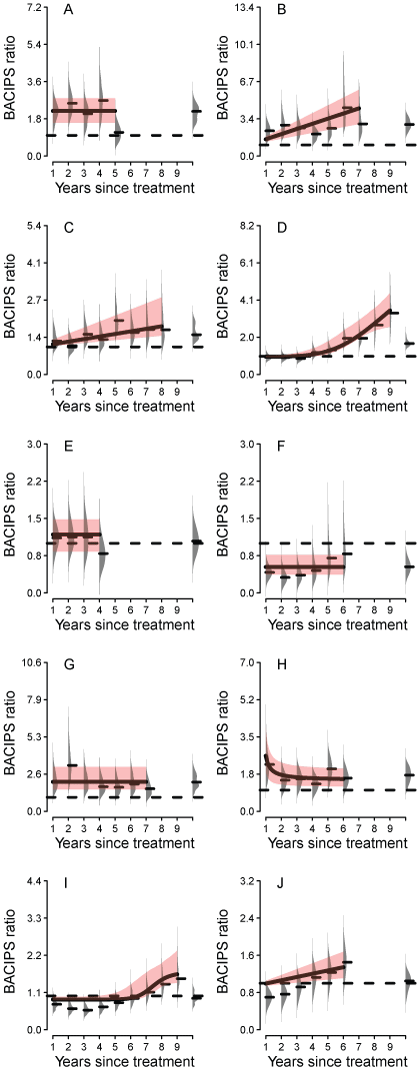
Before-After-Control-Impact Paired-Series (BACIPS) ratios representing changes in greater sage-grouse population abundance () by each lag year and averaged (mean) following application of A, hand cutting and B, mastication techniques for conifer removal; C, seeding and D, planting sagebrush; E, chemical and F, mechanical removal of invasive weeds; G, restoration of wet meadows; H, fence modifications; and I, seasonal and J, permanent road closures within the Bi-State Distinct Population Segment during 2012–21. Posterior distributions (gray) and median ratios (short black lines) were calculated from outputs of Bayesian state-space models based on lek count data. Horizontal dashed line references a BACIPS ratio of 1 (no difference between control and treatment). The solid black lines (median) and red polygons (95-percent credible interval) represent predictions from the best fit Progressive Change BACIPS models.
We also detected variation in the time lag effects from CEs that focused on modifying anthropogenic features on the landscape. We detected a non-linear positive response in population abundance, to fence modifications, that declined after the first year (fig. 16H). Populations that were adjacent to fence modifications consistently outperformed their controls, both immediately (=2.57, 95-percent CRI=1.59–3.95) and 6 years post treatment (=1.52, 95-percent CRI=1.08–2.02), despite a negative trend in the asymptotic curve. We also detected support for positive population effects of road closures, with seasonal and permanent closures having sigmoidal and linear time lag effects (table 8; fig. 16I, J), respectively. We estimated maximum annual increases in the BACIPS ratio for seasonal and permanent road closures as 27 percent and 7 percent, respectively. Seasonal closures resulted in cumulative population increases of 60 percent (=1.60, 95-percent CRI=1.28–2.37), which represented a 25 percent improvement over permanent closures (=1.35, 95-percent CRI=1.06–1.68), despite underperforming controls during the initial years of the post-treatment period. However, only 6 years of post-implementation monitoring were available for permanent road closures compared to 9 years for seasonal closures, the cumulative effect of permanent closures outperformed the observed effect of seasonal closures after 6 years, and unlike the sigmoidal population response to seasonal closures, the linear response to permanent closures showed no sign of yet approaching an asymptote.
The traditional BACIPS approach significantly underestimated CE effectiveness during the later parts of the observed post-treatment period (for example, figs. 15, 16D) and the magnitude of expected population responses during future years (table 8), when temporal changes in the BACIPS ratios did not meet traditional BACIPS assumptions (that is, step change). Linear responses in sage-grouse performance following treatment were associated with conifer removal (mechanical; fig. 16B), sagebrush restoration (seeding; fig. 16C), and road closure (permanent; fig. 16J) projects, whereas sigmoidal or asymptotic responses were associated with sagebrush restoration (planting; fig. 16D), fence modification (fig. 16H), and road closure (seasonal; fig. 16I) projects. When the top model of a PC BACIPS analysis was the step model, we directly report the magnitude of the effect (M; table 8). When the top model of a PC BACIPS analysis was the asymptotic or sigmoidal model and L (half-life time required to reach the full effect) was greater than twice the post-treatment observation period (table 8), we report the predicted magnitude of the treatment effect (M). For linear models, the annual increase demonstrates constancy over the observed period, but linear population increases are unlikely to continue indefinitely, for example as the population approaches carrying capacity. The response of sage-grouse populations to sagebrush restoration efforts is expected to shift from linear to sigmoidal as seeds and seedlings, of limited value as sage-grouse food or cover, mature into larger shrubs that represent resources for sage-grouse. Linear population responses to CEs (for example, mechanical conifer removal) may be artifacts of too few years of post-treatment monitoring, whereas additional years of monitoring or larger sample sizes would likely indicate asymptotic or sigmoidal responses. Nevertheless, compared to a step change model, the linear models provided evidence of greater long-term improvements in abundance. We calculated the difference between the cumulative estimate (that is, PC BACIPS framework) and the step change estimate (traditional approach) for CEs associated with linear, asymptotic, or sigmoidal progressive change response curves to demonstrate scenarios where population effects indicated by the traditional approach were biased high or low. In most cases, the traditional approach resulted in an estimate that was biased low. However, with fence modifications the estimate produced by the traditional approach was biased high. All other CEs produced cumulative effects estimates that exceeded the step effect. Available data allowed us to compare the magnitude of cumulative effects to the estimated step effect at different post-treatment time lags. We estimated increases relative to the step change model (that is, the magnitude of bias relative to the step change model) for conifer removal (mechanical) at 7 years post-treatment (146 percent), sagebrush restoration (seeding) at 8 years post-treatment (30 percent), sagebrush restoration (planting) at 9 years post-treatment (179 percent), road closure (seasonal) at 9 years post-treatment (67 percent), and road closure (permanent) at 6 years post-treatment (30 percent).
Interpretation and Synthesis
We presented timely science on the population trends, distribution, habitat selection, and efficacy of implemented conservation actions in the Bi-State DPS and provided an update to previous assessments of the Bi-State DPS (Coates and others, 2019) using the most up-to-date data and methodology. First, we mapped sage-grouse habitat within the Bi-State region across seasons and reproductive life stages to provide a more complete understanding of habitat relationships and inform improved prioritization of key areas for conservation or restoration. Second, we evaluated the metapopulation status of sage-grouse within the Bi-State DPS by examining trends in both abundance and distribution. Third, we assessed the efficacy of conservation actions that have been implemented within the Bi-State region to improve sage-grouse population performance and prevent further regulatory actions and ESA listing by the USFWS. In the following paragraphs, we interpret and discuss the implications of our results including important caveats and limitations.
Objective 1—Map Sage-Grouse Habitat Selection and Demographic Performance Within the Bi-State Distinct Population Segment
Although resource selection analyses and associated maps are often used by managers to inform conservation actions (Crawford and others, 2020; Pratt and Beck, 2021; Saher and others, 2022), incorporating data on demographic performance provides more robust inferences regarding spatial patterns of habitat quality. Integrated analyses of space use and demographic data, such as survival or reproductive success, can improve inferences when ranking habitat for management priority, particularly when multiple life stages across large spatial extents are considered (Stephens and others, 2015; Gibson and others, 2016; Pratt and Beck, 2021). We identified important habitat covariates underlying sage-grouse habitat selection patterns across three seasons and reproductive life stages and, for reproductive life stages, provided a quantitative approach to differentiate productive habitat supporting both high selection and demographic performance (for example, nest success and brood success) from areas of maladaptive selection (that is, where selection and demographic performance are misaligned). From a management perspective, productive habitat that supports both high selection and high reproductive success can provide critical value for long-term conservation (Crawford and others, 2020; O’Neil and others, 2020; Saher and others, 2022). Areas with maladaptive habitat selection (that is, those with high selection but corresponding low reproductive success), however, could represent sink habitat for a specific life stage (for example, nesting sinks). Sinks and ecological traps may be important targets for further investigations assessing the mechanisms leading to poor reproductive performance and options for habitat improvement or restoration (Holbrook and others, 2017). In particular, the variation in amount of sink habitat across life stages could have implications for where habitat improvement would be best targeted. Considering only habitat occupancy and selection patterns could inadvertently lead to the conservation of selected yet poor-quality habitat wherein individuals experience reduced survival or poor demographic performance. However, understanding selection patterns and cues is still critical, as all individuals contribute to population performance. Maps that integrate ranked indices for habitat selection with demographic performance during reproductive life stages can be combined with information on species occupancy (for example, the abundance and space use index used to delineate example habitat management categories; Coates and others, 2016a) to further inform the targeting of management practices, such as reintroduction, in areas with high potential but minimal current occupancy.
Our results generally aligned with previous research evaluating habitat selection by sage-grouse and were consistent across life stages and seasons, with a few exceptions. Shrub cover and elevation consistently had positive effects on selection, but pinyon-juniper cover and burned area had negative effects, aligning with previous research (Coates and others, 2017; O’Neil and others, 2020). Selection for hydrologic variables, including wet meadows, was more variable, with selection for these features generally being strongest during late brood-rearing, summer, and winter, corroborating previous research (Saher and others, 2022).
By simultaneously evaluating both habitat selection and demographic performance, we found evidence for two examples of mismatches (with burned areas and with conifer encroachment) during reproductive life stages. First, wildfire is drastically changing ecosystems across western North America (Flannigan and others, 2009), and our results indicated mismatches between selection patterns and survival consequences within burned areas. Sage-grouse avoided burned habitat during the brood-rearing life stage despite experiencing higher brood survival in those areas. However, only a small proportion (2 percent) of nests were within fire perimeters, so the apparent avoidance during brood-rearing may be at least partially due to a lack of burned areas accessible to sage-grouse broods. Previous studies have documented negative effects of wildfire on sage-grouse across multiple life stages (O’Neil and others, 2020; Anthony and others, 2022; Tyrrell and others, 2023143), but these studies have largely examined effects of recent megafires (Dudley and others, 2021; Anthony and others, 2022) and positive feedback between fire and annual grass invasion, which is particularly prevalent in other parts of the Great Basin (Coates and others, 2016b; Germino and others, 2016; Brussee and others, 2022). In contrast, megafires combined with invasive annual grasses are relatively rare in the Bi-State region with only 4.5 percent of burned areas having greater than 10 percent annual grass cover. Consistent with our findings, previous studies have also documented positive short-term effects of wildfire on brood survival in the Great Basin where cheatgrass is not prevalent, presumably due to increased herbaceous cover and forb production (Brussee and others, 2022). Identifying productive habitats that support both high selection and high survival across multiple life stages, especially relative to burned areas, will be important to guide habitat management under changing conditions, particularly given the large-scale threat posed by wildfire in sagebrush ecosystems.
Increased conifer cover has also been shown to have negative effects on population performance of sage-grouse (Olsen and others, 2021), and our results indicated strong avoidance of pinyon-juniper cover across all seasons, but that avoidance decreased during early and late brood-rearing. Previous research documented decreased adult survival in areas with more conifer cover (Coates and others, 2017), whereas brood success was improved by conifer removal (Sandford and others, 2017). Areas experiencing conifer encroachment may represent an ecological trap, particularly at low levels of encroachment when shrubs are still dominant. During the initial stages of conifer encroachment, other resources may still appear attractive and be selected despite the increased presence of predators such as perching raptors (Coates and others, 2017). However, due to strong avoidance across all seasons and life stages, we detected little to no evidence of an effect of conifer cover on survival during reproductive life stages, although uncertainty around the effect on early brood survival was high. Decreased avoidance during brood-rearing may reflect a trade-off, where broods move up in elevation to access mesic areas that provide necessary cover and forage resources (Aldridge and Boyce, 2007; Donnelly and others, 2018), but encounter increased pinyon-juniper cover potentially with negative survival consequences, although our results did not support effects on survival. Access to forage resources associated with restricted mesic areas, may be most important during the late brood-rearing period, which is typically characterized by low precipitation and desiccation of lowland vegetation. Furthermore, chicks are larger and more mobile during the late brood-rearing stage, rendering them less susceptible to predators. Identification of such trade-offs provides a means for managers to prioritize management actions, for example by focusing restoration on conifer removal in areas that support high selection but reduced survival or where there is a need for better connectivity among seasonal habitats.
Our study did have several limitations. We only evaluated the relative intensity of habitat selection across different life stages and seasons, and we did not incorporate movement dynamics of birds within or among seasons. Nevertheless, areas where individuals can fulfill the needs of their annual cycle while minimizing movements among seasonal habitats should support higher demographic success. Our analysis identified priority areas that benefit multiple life stages, that is, while minimizing the need for risky movements (Gibson and others, 2017; Prochazka and others, 2017). In addition, we did not have sufficient data to model individual chick survival and instead evaluated overall brood survival as a binary variable resulting in lower resolution information. Marking and tracking individual chicks is challenging, but modeling chick survival would provide more detailed inferences about overall reproductive success and productivity (Dahlgren and others, 2010). Further, some covariates affecting habitat quality, such as precipitation, vary temporally but are difficult to sufficiently capture in a habitat selection analysis. However, our study spanned a 17-year period, which should provide useful relative indices of temporally dynamic factors like climate variability, which is a key extrinsic driver of population dynamics in the Bi-State DPS (Coates and others, 2018). Finally, by evaluating survival only during reproductive life stages, we identified areas that might represent sinks for individual life stages, but we were unable to identify population level sinks, that is, where habitat selection is high but population growth rates negative. Nevertheless, we would expect areas supporting lower reproductive success to have corresponding lower population growth rates given the large effect that reproduction has on sage-grouse population dynamics (Taylor and others, 2012).
Evaluating habitat selection across seasons and reproductive life stages, and survival across key reproductive life stages, provided a more complete understanding of life history requirements and constraints for sage-grouse. Our results, combined with widely available remotely sensed data, allow managers to anticipate patterns of sage-grouse selection and survival across large spatial extents and to target conservation and restoration efforts where they will have the largest effect. For example, conservation efforts could focus on productive areas that support high habitat selection and high survival. Alternatively, areas of maladaptive habitat selection, that have high selection but low survival, may be better prioritized for restoration efforts to improve habitat quality and subsequent demographic performance. By spatially identifying areas of misalignment between selection and survival and linking them to underlying habitat covariates, managers can begin to identify the mechanisms underlying ecological traps and implement management actions to offset them. Measures of current occupancy can also be paired with maps of habitat selection and survival to guide management actions such as reintroductions (Picardi and others, 2022), which might be more successful in currently unoccupied areas of high habitat quality. Overall, ranking habitat based on metrics of both the intensity of selection and demographic performance across seasons or life stages can be used to tailor management recommendations to specific areas.
Objective 2—Evaluate Population Abundance, Trends, and Distribution of Sage-Grouse Within the Bi-State Distinct Population Segment
The Bi-State DPS declined slightly, ~1.2–2.5 percent per year, over six population cycles. However, the rate of decline was lowest over the most recent population cycle, when the conservation actions discussed in the “Objective 3” section were implemented. Trends in distribution varied among subpopulations, with an estimated decline of 156 km2 in occupied area across the Bi-State DPS from 1995 to 2019. Declines in abundance and spatial distribution were not distributed evenly across subpopulations, with both abundance and area increasing or remaining stable in one core and one peripheral subpopulation but declining or diverging in all other subpopulations. Important variation among subpopulations resulted in increases in both population abundance and distribution in expanding populations generally being insufficient to offset decreases in declining populations with the distribution of the Bi-State DPS becoming more concentrated in a smaller area. However, reductions in the distribution, and implications regarding changing habitat suitability, may have gone unnoticed if we had focused only on overall metapopulation abundance. Furthermore, if the spatial distribution of sage-grouse in the Bi-State DPS as a whole and of individual subpopulations are contracting more quickly than abundance is declining, the remaining populations may be subject to intensified density dependence and may experience further declines before reverting toward a lower carrying capacity.
Previous population trends for the Bi-State DPS, estimated using the IPM approach, were largely stable to slightly negative during the 1995–2018 (λ=1.02), 2001–18 (λ=0.99), and 2008–18 (λ=0.99) periods (Coates and others, 2019). A separate analysis, using SSMs, estimated a population rate change of =0.991 from 1995 to 2018 and =0.987 from 2008 to 2018 (Coates and others, 2023). Our population rate of change estimates, extending to the most-recent population nadir in 2019, indicate that population rates of change remain slightly negative across all temporal scales considered (for example, =0.987 from 1995 to 2018), although population rates of change did improve over the most recent population cycle (=0.988 from 2008 to 2018). Hence, population rates of change appear to have remained steady or increased slightly since the 2013 listing proposal (U.S. Fish and Wildlife Service, 2013). Furthermore, 95-percent credible intervals (which did not overlap with λ=1 in all population cycles except the most recent) provide strong evidence for a continuing population decline across the Bi-State DPS as a whole, although the rate of decline was lower in recent years.
The SSM implemented here did have some differences from the model used to estimate previously published trends (Coates and others, 2023), which used range-wide lek data and included a nested random effects structure that was not possible for a single regional population. In the range-wide model, the regional precision parameter was informed by data from across the range of sage-grouse and so could encompass greater inter-annual, environmental variability (Coates and others, 2023), whereas the model presented here was limited to data from the Bi-State DPS. Nevertheless, trend estimates for all population cycles were generally consistent between models. In addition, whereas the IPM allowed for greater precision by incorporating demographic data, the SSM allowed us to estimate trends in population abundance over a longer time frame, thus providing greater context for current (2024) population trends in the Bi-State DPS.
Population declines across the Bi-State DPS were not as severe as those seen in other portions of the sage-grouse range (Coates and others, 2021). However, trends in the Bi-State DPS were uneven, with peripheral subpopulations, in particular, suffering declines in both abundance and distribution, which is consistent with patterns seen across the species range (Coates and others, 2021). As a result of these trends, the Bi-State DPS appeared to be contracting, although important variation existed among subpopulations, and multiple smaller peripheral subpopulations may be at risk of extirpation. With the loss of peripheral subpopulations, the Bi-State DPS could be more vulnerable to catastrophes, including severe wildfires, because the overall population has become more concentrated in a smaller area (Den Boer, 1968; Quinn and Hastings, 1987; White and others, 2021). More isolated populations may also be subject to decreasing rates of heterozygosity and the risk of genetic bottlenecks that threaten long-term population viability (Bush and others, 2011). Conservation strategies have traditionally targeted large contiguous areas of habitat, but several small patches of habitat can increase the probability of population persistence by distributing extinction risk over multiple sites (Den Boer, 1968; Hammill and Clements, 2020). In a metapopulation like the Bi-State DPS, multiple small subpopulations can act as an insurance policy, particularly in the face of disturbance, by increasing redundancy, improving the resiliency of subpopulations connected by emigration processes, and buffering the metapopulation from genetic bottlenecks (Den Boer, 1968; Kallimanis and others, 2005; White and others, 2021). Collectively, this indicates that the probability of long-term persistence of the Bi-State DPS would be improved with the retention of peripheral subpopulations. The Bi-State DPS would have greater redundancy with more subpopulations spread over a larger area and thus be more resilient and allow for any given subpopulation to be affected by disturbance with fewer consequences to the DPS as a whole (Den Boer, 1968; Caughley, 1994; Fox and others, 2017).
Changes in habitat may explain changes in population abundance and distribution in the Bi-State DPS over the last 25 years as habitat fragmentation at fine scales can reduce carrying capacity (O’Neil and others, 2020). Abundance in one of the largest core subpopulations (A-007) declined by 59 percent between 1995 and 2019, whereas abundance of another core subpopulation (A-003) increased by 17 percent since 1995 and accounted for 63 percent of all birds in the Bi-State DPS in 2019. The main difference is that the growing core subpopulation (A-003) has maintained relatively intact and contiguous habitat (Coates and others, 2018), whereas the declining core subpopulation (A-007) suffered habitat degradation due to the interacting effects of drought and water diversions, with resulting losses of key mesic habitats that are crucial during the brood-rearing phase (Severson and others, 2022).
Habitat loss due to both wildfire and conifer encroachment has also occurred in peripheral subpopulations in the Bi-State DPS (Oyler-McCance and others, 2014; Coates and others, 2016b33; 2017). Large wildfires can contribute to both acute effects on sage-grouse demographics (Anthony and others, 2022; Tyrrell and others, 2023) and long-term declines in sagebrush habitat availability and integrity (Coates and others, 2016b), but have been relatively rare in the Bi-State DPS, historically. However, wildfires have particularly affected the northern subpopulations, which have experienced some of the most precipitous declines among subpopulations in the Bi-State DPS. Wildfire in sagebrush ecosystems also initiates a feedback loop with increased invasive annual grasses (Coates and others, 2016b; Germino and others, 2016; Brussee and others, 2022), and the cumulative effects can negatively affect sage-grouse across multiple life stages (O’Neil and others, 2020; Anthony and others, 20225). Sage-grouse populations can also be negatively affected by conifer expansion (Olsen and others, 2021), which, given avoidance, can reduce usable space for nest sites, and can affect adult survival and brood success, in part due to the increased presence of predators such as perching raptors (Coates and others, 2017; Sandford and others, 2017; Severson and others, 2017). Particularly in the northern subpopulations, conifer expansion into sagebrush has reduced available habitat and resulted in small, isolated patches of sagebrush (Oyler-McCance and others, 2014), which combined with increased wildfire effects, has likely affected the declines in both abundance and distribution of peripheral subpopulations.
Although habitat loss and degradation likely affected observed trends in the Bi-State DPS, sage-grouse populations are also driven strongly by climatic cycles (Coates and others, 2018). The Bi-State DPS experienced several especially wet years, starting in 2010, followed by a severe drought (Coates and others, 2018) and subsequent precipitous drop in sage-grouse population abundance. Because productivity in sagebrush and other cold-desert ecosystems is closely tied to precipitation and temperature (Noy-Meir, 1973), sage-grouse population cycles are partially driven by pulses of primary productivity associated with above-average precipitation that increases resources available for reproduction and survival (Fedy and Doherty, 2011; Blomberg and others, 2013a; Gibson and others, 2017; Coates and others, 2018). Trends in population abundance in the Bi-State DPS appeared to track climate cycles, but the pattern was less apparent in trends in distribution which may have longer lag times, although that merits further investigation. The area occupied briefly expanded following years of above-average precipitation, but the increase was dampened and occurred several years after the sharp upward increase in abundance. This disconnect could occur if increases in abundance occurred at large leks such that density increased without occupying more area. However, if increases in area do not follow those in abundance during favorable years, this could represent a potential decoupling, with implications for population persistence, because it reinforces contraction to a single large core population if peripheral populations do not expand or are not recolonized in favorable years.
We estimated changes in both population abundance and spatial distribution over three population cycles to assess the combined effects of declining abundance and loss of habitat on the distribution of sage-grouse in the Bi-State DPS by combining multiple sources of data, including population counts, demographic data, and information on space use from marked individuals. In addition, we were able to leverage population counts to evaluate trends in abundance from the 1960s to provide a larger context of trends in the Bi-State DPS. By evaluating changes in both abundance and distribution, we were able to evaluate potential misalignments that could have implications for population persistence and would be missed if both were not evaluated together. For example, by evaluating variation in trends among subpopulations, we observed that increases in expanding populations were insufficient to offset decreases in declining populations. The net result is that the overall distribution of the Bi-State DPS is becoming more concentrated in a smaller area. Preventing the extirpation of peripheral populations while conserving one to two core populations can improve population resiliency, increase gene flow, and improve metapopulation functionality (Den Boer, 1968; Hammill and Clements, 2020; Ancrenaz and others, 2021). Thus, conservation planning for the Bi-State DPS may benefit from considering actions that prevent extirpation of small satellite populations that may not appear significant with regards to population numbers but may have implications for long-term population persistence.
Objective 3—Evaluate Efficacy of Ongoing Conservation Actions Targeting Sage-Grouse Within the Bi-State Distinct Population Segment
Based on our results, there was clear empirical evidence that a suite of conservation actions carried out at broad spatial and temporal scales within the Bi-State DPS benefited sage-grouse populations, increasing average annual population abundance by ~4.4 percent, relative to controls, with cumulative increases of 37.4 percent since 2012. Conservation actions represented cooperative conservation efforts among public, non-profit, private, academic, state, and federal agencies and organizations based on an inter-agency action plan (Bi-State Technical Advisory Committee, 2012) that had the stated goal of improving sage-grouse population growth. Long-term population monitoring and empirical assessment of management action effectiveness were mandated to evaluate the success of the conservation plan.
There was considerable variation in effectiveness among different types of conservation actions and their time-lagged effects, with some actions having immediate effects, whereas others required longer time periods to affect sage-grouse populations. Conservation actions also varied with respect to the duration of their effects. For example, road closures resulted in lagged improvements to population growth rates, with BACIPS ratios only exceeding one (that is, treated populations outperformed controls) several years after treatment. The positive effects of road closures then continued to increase in magnitude across years, though the effect of seasonal road closures took a sigmoidal shape that indicated tapering over time, whereas the effect of permanent road closures continued to increase in a linear fashion. In contrast, fence modifications were associated with an immediate positive effect in the first year after implementation, but that effect tapered off in subsequent years, whereas restoration of wet meadows showed an immediate and relatively constant effect. In addition, different approaches to sagebrush restoration (that is, seeding versus planting) had different magnitudes of improvement in growth rates, with planting improving population growth more significantly than seeding. These different temporal dynamics indicate that long-term monitoring is essential to ensuring detection and accurate characterization of the effects of conservation actions and efficacy.
We documented strong evidence that hand cutting and mechanically removing conifers had immediate and positive effects relative to controls, which corroborates a study in Oregon that concluded that population growth of sage-grouse increased by 25 percent following conifer treatments, relative to controls (Severson and others, 2017). Similarly, another study demonstrated increases in nest and brood survival following conifer treatment (Sandford and others, 2017). Conifer cover negatively affects lek persistence (Baruch-Mordo and others, 2013) and survival (Coates and others, 2017) and can also increase movement rates of sage-grouse, thus exposing them to a higher risk of predation (Prochazka and others, 2017). Because trees provide elevated nesting and perching substrate for ravens and raptors, which are important predators of sage-grouse eggs (Coates and Delehanty, 2010; Lockyer and others, 2013) and adults (Blomberg and others, 2013b), we hypothesize that tree removal reduces predation risk, resulting in increased survival rates across multiple life stages and improved population performance relative to control populations. Although we earlier reported a slight tendency toward higher early brood survival in proximity to conifer cover (see the “Objective 1” section), this effect likely reflects the co-occurrence of early seral conifer encroachment and forage resources favored by broods, and conifer removal in such areas would likely further enhance brood survival.
In this analysis, many of the sagebrush restoration actions were addressing postfire rehabilitation by seeding or planting container-grown sagebrush seedlings into burned areas. Although wildfire is a natural process in sagebrush ecosystems (Bukowski and Baker, 2013), both the frequency and size of wildfires have increased significantly in recent decades (Brooks and others, 2015; Pilliod and others, 2017). In addition, invasive annual grasses (for example, Bromus tectorum) can reshape fire regimes through positive feedback loops where invasive grasses increase fuel loads and fine fuel continuity, increasing the probability of subsequent fires that remove sagebrush cover and favor the establishment of additional annual grasses (Ellsworth and others, 2020). This grass-wildfire feedback loop is well-documented in sagebrush ecosystems and negatively affects sage-grouse population growth (Coates and others, 2016b; Dudley and others, 2021) and multiple vital rates, including nest (Lockyer and others, 2013; Dudley and others, 2022) and female (Foster and others, 2019; Tyrrell and others, 2023) survival, which have major effects on population growth (Taylor and others, 2012). Importantly, despite large-scale efforts to restore sagebrush, few studies have quantitatively assessed effects of restoration on sage-grouse. Our results clearly indicate that sagebrush restoration actions positively affect sage-grouse populations, which is consistent with a recent study that concluded that sage-grouse in Idaho and Oregon selected for areas containing recovered sagebrush 3 years post-fire and avoided annual grasslands (Poessel and others, 2022).
In the Bi-State region, burned areas may naturally recover to their original state relatively quickly because sagebrush ecosystems there have greater resilience (that is, ability for vegetation to recover after disturbance) and resistance to invasives (hereinafter, R&R; Chambers and others, 2014) relative to most of the western portion of the sage-grouse range. In the absence of invasive annual grass, small scale fires may even provide short-term survival benefits during the brood-rearing stage, as suggested by both the results presented in the “Objective 1” section and previous research (Brussee and others, 2022). Nevertheless, sagebrush habitat loss is likely outpacing natural sagebrush re-establishment even in high R&R regions, particularly given contemporary wildfire regimes across the sagebrush biome (Coates and others, 2016b). Therefore, to facilitate recovery of sage-grouse habitat in a manner that keeps pace with typical rates of sage-grouse recruitment, targeted postfire restoration and rehabilitation actions are likely necessary (Pyke, 2011; Chambers and others, 2014; Ricca and Coates, 2020).
We documented that planting container-grown sagebrush seedlings within 5 km of leks significantly improved sage-grouse population growth relative to controls following a 5-year lag, which may indicate the recovery of nesting habitat because greater than 95-percent of sage-grouse nesting locations are within 5 km of leks (Coates and others, 2013). In addition, the lag effect was sigmoidal, that coincides with recent findings that planted sagebrush typically requires at least 4 years to realize the minimum recommended canopy cover for nesting sage-grouse (15 percent; Connelly and others, 2003) under average climatic conditions (Pyke and others, 2020). Therefore, planting high-density patches of sagebrush seedlings strategically within burned areas that encompass historical nesting areas will likely benefit sage-grouse populations, particularly where large wildfires have removed most large sagebrush plants. Our results were also largely consistent with previous findings that suitable nesting habitat for sage-grouse takes longer to establish from seeding methods, and seedling recruitment is far less reliable, compared to container-plantings (Pyke and others, 2020). Thus, planting seedlings may help promote reestablishment of larger sagebrush in sage-grouse nesting habitat and provide a jumpstart in the critical years post-fire (Monroe and others, 2019; Pyke and others, 2020; Ricca and Coates, 2020). Due to data limitations, we did not evaluate the effectiveness of removing or thinning existing sagebrush using chemical, mechanical, or prescribed burning treatments, which was historically done to improve sage-grouse habitats, but a recent study indicated mostly neutral or negative effects on sage-grouse population abundance lasting at least 11 years after the onset of such treatments to Wyoming big sagebrush (Artemisia tridentata wyomingensis; Smith and Beck, 2018). Collectively, conclusions from both our study and Smith and Beck (2018) highlight the importance of conserving relatively large stands of big sagebrush and actively restoring sagebrush after disturbance, particularly by planting container-grown seedlings. Effective restoration targeting nesting habitat after large-scale disturbance can be further guided by recent advances in quantitative decision-support tools (Ricca and others, 2018; Roth and others, 2022).
Interestingly, efforts to reduce invasive weeds did not significantly (chemical treatment) or positively (mechanical treatment) affect changes in sage-grouse abundance, which may be because invasive weeds provide some concealment for sage-grouse and the loss of such cover contributes to exposure of bare ground, particularly in the absence of a healthy perennial grass component. Regardless, reducing invasive grasses and other annual weeds is critically important to prevent their expansion, dampen the annual-wildfire cycle, and reduce the probability of landscapes transitioning from shrublands to annual grasslands. Our findings also emphasize the importance of recovering sagebrush and native perennial grasses immediately following reduction of invasive weeds. If data from more post-treatment years were available, it is possible that the step change models would be less appropriate and reveal a more complex recovery process, but based on the available post-treatment data, we detected modest anecdotal evidence for a non-linear response to mechanical weed removal.
We also detected evidence that sage-grouse population performance improved in relation to modification of anthropogenic infrastructure in sagebrush environments. Anthropogenic infrastructure is a well-documented threat to sage-grouse populations in remote sagebrush environments (Walker and others, 2007; Harju and others, 2010; Holloran and others, 2010; Green and others, 2017). Specifically, we hypothesize that road closures near lek sites may increase nest and adult survival probabilities through multiple mechanisms. First, roads can negatively affect the distribution of sage-grouse (Lyon and Anderson, 2003; Holloran and Anderson, 2005), primarily through noise pollution (Blickley and Patricelli, 2012). Roads can also accelerate the dispersal of exotic plant communities and lead to habitat loss (Gelbard and Belnap, 2003). Finally, adult survival is often lower near roads (Lyon and Anderson, 2003; Aldridge and Boyce, 2007), possibly due to direct collisions with vehicles. Predation rates may also be elevated as roads provide travel corridors for terrestrial predators (Dickie and others, 2020; Hill and others, 2021), and roadkill can attract large avian predators and corvids, which are effective predators of sage-grouse eggs (Coates and Delehanty, 2010; Lockyer and others, 2013). Although an extended period of time (7–8 years) was required for seasonal road closures to produce a BACIPS ratio above 1.0, our results indicate long-term cumulative benefits to sage-grouse populations from these types of conservation actions alone. With permanent road closures, positive effects were achieved relatively quickly and increased linearly with time. Similarly, we hypothesize that fence modification positively affected sage-grouse population growth through increases in juvenile and adult survival. Collisions with fences and related fatalities have been reported elsewhere (Christiansen, 2009; Stevens and others, 2012; Van Lanen and others, 2017), and our results corroborate previous findings that fence modifications, including placing reflective markers on fences, can positively affect adult survival (Stevens and others, 2012; Van Lanen and others, 2017).
There were multiple advantages to using PC BACIPS over the traditional BACIPS approach. For example, when temporal changes in the BACIPS ratios did not meet traditional BACIPS assumptions (that is, step change), then the traditional approach would have significantly underestimated CE effectiveness during the latter parts of the observed post-treatment period as well as expected population responses during future years. With linear models, yearly increases demonstrate ongoing population responses, which are unlikely to continue indefinitely due to limitations in carrying capacity. Actions, such as conifer removal (mechanical), may have an apparent linear response due to the post-treatment observation period being too narrow to reveal possible asymptotic or sigmoid response curves. Nevertheless, the linear models provided evidence of stronger population responses than would have been evident from the step-change model, inherent in the BACIPS approach. With sagebrush restoration, we would expect the response to shift from linear to sigmoidal as seedlings mature into plants that provide resources for grouse and growth rates eventually slow within mature sagebrush stands. Regardless, a traditional BACIPS approach would have severely underestimated the expected sage-grouse population response to sagebrush planting compared to our PC BACIPS approach, which estimated the strongest effects 7–9 years after planting.
Our study represents a novel and rigorous assessment of the effectiveness of multiple types of conservation actions in a distinct population segment of sage-grouse, but these outcomes may not reflect range-wide patterns, and more research would be necessary to elucidate how conservation actions can affect population abundance at range-wide scales. In addition, limitations in either data availability or quality (for example, incomplete or missing records of conservation actions) prevented us from differentiating how different scales or intensities of conservation actions affect population abundance. For example, we did not always have detailed data on the method used for a specific action (for example, mechanical versus hand tree removal), the spatiotemporal scale of the action, or whether local ecological conditions affected the implementation of actions. We still included incomplete data records in the analysis as a separate category (that is, indicated as CE type “unknown”) from complete data records, but the “unknown” category is composed of several different types of actions and should be treated as a generalized conservation action. Similarly, with our analytical approach, we were not able to differentiate potential effects of conservation actions based on the proportion of the lek buffer that intersected the conservation action, or the spatial extent of treatments, which likely affected the potential effects on the buffered lek. However, we could only evaluate presence/absence of a treatment within a given lek buffer. Higher levels of overlap between CE treatment areas and lek buffers could be associated with greater positive population responses, but further research is necessary to explore this relationship.
Overall, our results indicate that conservation actions have reduced the average annual rate of population decline of sage-grouse in the Bi-State DPS relative to those trends experienced in the absence of conservation actions. Further, the conservation efficacy we report may explain why sage-grouse in the Bi-State DPS are not declining as steeply as other nearby populations (Coates and others, 2021). According to a recent study on spatiotemporal trends in sage-grouse populations, there was an ~1.0 percent difference between sage-grouse population growth rates in the remainder of the Great Basin (λ=0.986) compared to in the Bi-State DPS (λ=0.995) since the mid-1990s (Coates and others, 2021). This difference is only slightly less than the percentage increase in rate of change attributed to conservation actions within the Bi-State DPS over the same time frame. Furthermore, sage-grouse experienced much greater annual declines (λ=0.956) in another geographically isolated population, in the northwestern portion of their range in Washington state (Coates and others, 2021). Although management and conservation actions also occurred outside the Bi-State region, both the frequency and intensity of conservation actions are likely the greatest in the Bi-State compared to elsewhere in their western range, with greater than 45 million dollars allocated to cooperative conservation in the past decade (Bi-State Technical Advisory Committee, 2012, 2022). Further, the Bi-State DPS occupies an area with relatively high resistance and resilience which should respond more readily to restoration activities. Expanding these analyses range-wide and investigating variation among regions in effectiveness of conservation actions would help inform national land-use plans and conservation design strategies currently (2024) underway. We demonstrated the potential positive effects of multiple conservation actions on sage-grouse growth rates, but populations are ultimately limited by environmental carrying capacity. In recent decades, sagebrush ecosystems have experienced habitat degradation and loss, including due to increased invasive annual grasses, conifer expansion, and anthropogenic disturbance (Wisdom and others, 2005), which has likely decreased the carrying capacity for sage-grouse and led to decreasing growth rates and population decline. Our results indicated that actions that helped restore habitat quality and extent (for example, conifer removal and sagebrush restoration) were some of the most effective at improving sage-grouse population growth rates over long time frames, likely because they increased the carrying capacity of previously degraded habitat. Thus, future conservation actions implemented as part of a strategic conservation plan may help reverse negative trends in sage-grouse abundance by restoring both the quality and quantity of habitat to support historic population carrying capacities.
References Cited
Aeberhard, W.H., Flemming, J.M., and Nielsen, A., 2018, Review of state-space models for fisheries science: Annual Review of Statistics and Its Application, v. 5, no. 1, p. 215–235. [Available at https://doi.org/10.1146/annurev-statistics-031017-100427.]
Aldridge, C.L., and Boyce, M.S., 2007, Linking occurrence and fitness to persistence—Habitat based approach for endangered greater sage-grouse: Ecological Applications, v. 17, no. 2, p. 508–526. [Available at https://doi.org/10.1890/05-1871.]
Ammann, G.A., 1944, Determining the age of pinnated and sharp-tailed grouses: The Journal of Wildlife Management, v. 8, no. 2, p. 170–171. [Available at https://doi.org/10.2307/3796451.]
Ancrenaz, M., Oram, F., Nardiyono, N., Silmi, M., Jopony, M.E.M., Voigt, M., Seaman, D.J.I., Sherman, J., Lackman, I., Traeholt, C., Wich, S.A., Santika, T., Struebig, M.J., and Meijaard, E., 2021, Importance of small forest fragments in agricultural landscapes for maintaining orangutan metapopulations: Frontiers in Forests and Global Change, v. 4, 7 p. [Available at https://doi.org/10.3389/ffgc.2021.560944.]
Anthony, C.R., Foster, L.J., Hagen, C.A., and Dugger, K.M., 2022, Acute and lagged fitness consequences for a sagebrush obligate in a post mega‐wildfire landscape: Ecology and Evolution, v. 12, no. 1, 12 p. [Available at https://doi.org/10.1002/ece3.8488.]
Avgar, T., Lele, S.R., Keim, J.L., and Boyce, M.S., 2017, Relative selection strength—Quantifying effect size in habitat- and step-selection inference: Ecology and Evolution, v. 7, no. 14, p. 5322–5330. [Available at https://doi.org/10.1002/ece3.3122.]
Baruch-Mordo, S., Evans, J.S., Severson, J.P., Naugle, D.E., Maestas, J.D., Kiesecker, J.M., Falkowski, M.J., Hagen, C.A., and Reese, K.P., 2013, Saving sage-grouse from the trees—A proactive solution to reducing a key threat to a candidate species: Biological Conservation, v. 167, p. 233–241. [Available at https://doi.org/10.1016/j.biocon.2013.08.017.]
Bi-State Technical Advisory Committee, 2012, Bi-state action plan—Past, present, and future actions for conservation of the greater sage-grouse Bi-state distinct population segment: Prepared by the Bi-State Technical Advisory Committee for the Bi-State Executive Oversight Committee for the Conservation of Greater Sage-Grouse, 158 p., accessed August 17, 2023, at https://bistatesagegrouse.com/sites/default/files/fileattachments/general/page/301/bi-stateactionplan2012.pdf.
Bi-State Technical Advisory Committee, 2022, Bi-State Sage-Grouse 10-year accomplishment report 2012–2021: Bi-State Sage-Grouse, California–Nevada, 58 p., accessed December 8, 2023, at https://bistatesagegrouse.com/sites/default/files/fileattachments/general/page/950/bi_state_tenyearreport_final-min.pdf.
Blickley, J.L., and Patricelli, G.L., 2012, Potential acoustic masking of Greater Sage-Grouse (Centrocercus urophasianus) display components by chronic industrial noise: Ornithological Monographs, chap. 3, no. 74, p. 23–35. [Available at https://doi.org/10.1525/om.2012.74.1.23.]
Blomberg, E.J., Poulson, S.R., Sedinger, J.S., and Gibson, D., 2013a, Prefledging diet is correlated with individual growth in Greater Sage-Grouse (Centrocercus urophasianus): The Auk, v. 130, no. 4, p. 715–724. [Available at https://doi.org/10.1525/auk.2013.12188.]
Blomberg, E.J., Sedinger, J.S., Gibson, D., Coates, P.S., and Casazza, M.L., 2014, Carryover effects and climatic conditions influence the postfledging survival of greater sage‐grouse: Ecology and Evolution, v. 4, no. 23, p. 4488–4499. [Available at https://doi.org/10.1002/ece3.1139.]
Blomberg, E.J., Sedinger, J.S., Nonne, D.V., and Atamian, M.T., 2013b, Seasonal reproductive costs contribute to reduced survival of female greater sage-grouse: Journal of Avian Biology, v. 44, p. 149–158. [Available at https://doi.org/10.1111/j.1600-048X.2012.00013.x.]
Boyce, M.S., 2006, Scale for resource selection functions: Diversity and Distributions, v. 12, no. 3, p. 269–276. [Available at https://doi.org/10.1111/j.1366-9516.2006.00243.x.]
Boyte, S.P., and Wylie, B.K., 2016, Near-real-time cheatgrass percent cover in the Northern Great Basin, USA, 2015: Rangelands, v. 38, no. 5, p. 278–284. [Available at https://doi.org/10.1016/j.rala.2016.08.002.]
Braun, C.E., Budeau, D.A., and Schroeder, M.A., 2015, Fall population structure of sage-grouse in Colorado and Oregon: Oregon Department of Fish and Wildlife, Wildlife Technical Report 005–2015, 72 p., accessed November 9, 2022, at https://www.dfw.state.or.us/wildlife/research/docs/Fall_Popn_Structure_Sage-grouse_v3182015.pdf.
Brooks, S.P., and Gelman, A., 1998, General methods for monitoring convergence of iterative simulations: Journal of Computational and Graphical Statistics, v. 7, no. 4, p. 434–455. [Available at https://doi.org/10.1080/10618600.1998.10474787.]
Brooks, M.L., Matchett, J.R., Shinneman, D.J., and Coates, P.S., 2015, Fire patterns in the range of the greater sage-grouse, 1984–2013—Implications for conservation and management: U.S. Geological Survey Open-File Report 2015–1167, 66 p., accessed December 8, 2023, at https://doi.org/10.3133/ofr20151167.
Brussee, B.E., Coates, P.S., O’Neil, S.T., Casazza, M.L., Espinosa, S.P., Boone, J.D., Ammon, E.M., Gardner, S.C., and Delehanty, D.J., 2022, Invasion of annual grasses following wildfire corresponds to maladaptive habitat selection by a sagebrush ecosystem indicator species: Global Ecology and Conservation, v. 37, 19 p. [Available at https://doi.org/10.1016/j.gecco.2022.e02147.]
Bukowski, B.E., and Baker, W.L., 2013, Historical fire regimes, reconstructed from land‐survey data, led to complexity and fluctuation in sagebrush landscapes: Ecological Applications, v. 23, no. 3, p. 546–564. [Available at https://doi.org/10.1890/12-0844.1.]
Bush, K.L., Dyte, C.K., Moynahan, B.J., Aldridge, C.L., Sauls, H.S., Battazzo, A.M., Walker, B.L., Doherty, K.E., Tack, J., Carlson, J., Eslinger, D., Nicholson, J., Boyce, M.S., Naugle, D.E., Paszkowski, C.A., and Coltman, D.W., 2011, Population structure and genetic diversity of greater sage-grouse (Centrocercus urophasianus) in fragmented landscapes at the northern edge of their range: Conservation Genetics, v. 12, p. 527–542. [Available at https://doi.org/10.1007/s10592-010-0159-8.]
Caughley, G., 1994, Directions in conservation biology: Journal of Animal Ecology, v. 63, no. 2, p. 215–244. [Available at https://doi.org/10.2307/5542.]
Chambers, J.C., Miller, R.F., Board, D.I., Pyke, D.A., Roundy, B.A., Grace, J.B., Schupp, E.W., and Tausch, R.J., 2014, Resilience and resistance of sagebrush ecosystems—Implications for state and transition models and management treatments: Rangeland Ecology and Management, v. 67, no. 5, p. 440–454. [Available at https://doi.org/10.2111/REM-D-13-00074.1.]
Christiansen, T., 2009, Fence marking to reduce Greater Sage-grouse (Centrocercus urophasianus) collisions and mortality near Farson, Wyoming—Summary of interim results: Cheyenne, Wyo., Wyoming Game and Fish Department, 3 p., accessed December 8, 2023, at https://www.nrc.gov/docs/ML1108/ML110830116.pdf.
Coates, P.S., Brussee, B.E., Ricca, M.A., Severson, J.P., Casazza, M.L., Gustafson, K.B., Espinosa, S.P., Gardner, S.C., and Delehanty, D.J., 2020, Spatially explicit models of seasonal habitat for greater sage‐grouse at broad spatial scales—Informing areas for management in Nevada and northeastern California: Ecology and Evolution, v. 10, no. 1, p. 104–118. [Available at https://doi.org/10.1002/ece3.5842.]
Coates, P.S., Casazza, M.L., Blomberg, E.J., Gardner, S.C., Espinosa, S.P., Yee, J.L., Wiechman, L., and Halstead, B.J., 2013, Evaluating greater sage‐grouse seasonal space use relative to leks—Implications for surface use designations in sagebrush ecosystems: The Journal of Wildlife Management, v. 77, no. 8, p. 1598–1609. [Available at https://doi.org/10.1002/jwmg.618.]
Coates, P.S., Casazza, M.L., Ricca, M.A., Brussee, B.E., Blomberg, E.J., Gustafson, K.B., Overton, C.T., Davis, D.M., Niell, L.E., Espinosa, S.P., Gardner, S.C., and Delehanty, D.J., 2016a, Integrating spatially explicit indices of abundance and habitat quality—An applied example for greater sage-grouse management: Journal of Applied Ecology, v. 53, no. 1, p. 83–95. [Available at https://doi.org/10.1111/1365-2664.12558.]
Coates, P.S., and Delehanty, D.J., 2010, Nest predation of greater sage-grouse in relation to microhabitat factors and predators: The Journal of Wildlife Management, v. 74, no. 2, p. 240–248. [Available at https://doi.org/10.2193/2009-047.]
Coates, P.S., Prochazka, B.G., Aldridge, C.L., O’Donnell, M.S., Edmunds, D.R., Monroe, A.P., Hanser, S.E., Wiechman, L.A., and Chenaille, M.P., 2023, Range-wide population trend analysis for greater sage-grouse (Centrocercus urophasianus)—Updated 1960–2022: U.S. Geological Survey Data Report 1175, 17 p., accessed December 7, 2023, at https://doi.org/10.3133/dr1175.
Coates, P.S., Prochazka, B.G., O’Donnell, M.S., Aldridge, C.L., Edmunds, D.R., Monroe, A., Ricca, M.A., Wann, G.T., Hanser, S.E., and Wiechman, L.A., 2021, Range-wide greater sage-grouse hierarchical monitoring framework—Implications for defining population boundaries, trend estimation, and a targeted annual warning system: U.S. Geological Survey Open-File Report 2020–1154, 243 p., accessed September 23, 2022, at https://doi.org/10.3133/ofr20201154.
Coates, P.S., Prochazka, B.G., Ricca, M.A., Gustafson, K.B., Ziegler, P., and Casazza, M.L., 2017, Pinyon and juniper encroachment into sagebrush ecosystems impacts distribution and survival of greater sage-grouse: Rangeland Ecology and Management, v. 70, no. 1, p. 25–38. [Available at https://doi.org/10.1016/j.rama.2016.09.001.]
Coates, P.S., Prochazka, B.G., Ricca, M.A., Halstead, B.J., Casazza, M.L., Blomberg, E.J., Brussee, B.E., Wiechman, L., Tebbenkamp, J., Gardner, S.C., and Reese, K.P., 2018, The relative importance of intrinsic and extrinsic drivers to population growth vary among local populations of Greater Sage-Grouse—An integrated population modeling approach: The Auk, v. 135, no. 2, p. 240–261. [Available at https://doi.org/10.1642/AUK-17-137.1.]
Coates, P.S., Ricca, M.A., Prochazka, B.G., Brooks, M.L., Doherty, K.E., Kroger, T., Blomberg, E.J., Hagen, C.A., and Casazza, M.L., 2016b, Wildfire, climate, and invasive grass interactions negatively impact an indicator species by reshaping sagebrush ecosystems: Proceedings of the National Academy of Sciences of the United States of America, v. 113, no. 45, p. 12745–12750. [Available at https://doi.org/10.1073/pnas.1606898113.]
Coates, P.S., Ricca, M.A., Prochazka, B.G., O’Neil, S.T., Severson, J.P., Mathews, S.R., Espinosa, S., Gardner, S., Lisius, S., and Delehanty, D.J., 2019, Population and habitat analyses for greater sage-grouse (Centrocercus urophasianus) in the Bi-State Distinct Population Segment—2018 update: U.S. Geological Survey Open-File Report 2019–1149, 122 p. [Available at https://doi.org/10.3133/ofr20191149.]
Coates, P.S., Milligan, M.C., Brussee, B.E., O’Neil, S.T., and Chenaille, M.P., 2024a, Rasters and tables for selection and survival of Greater Sage-grouse nests and broods in the Bi-State Distinct Population Segment of California and Nevada: U.S. Geological Survey data release. [Available at https://doi.org/10.5066/P95HTJG8.]
Coates, P.S., Milligan, M.C., Brussee, B.E., O’Neil, S.T., and Chenaille, M.P., 2024b, Greater sage-grouse habitat selection, survival, abundance, and space-use in the Bi-State Distinct Population Segment of California and Nevada: U.S. Geological Survey data release. [Available at https://doi.org/10.5066/P1AATW9D.]
Connelly, J.W., Reese, K.P., and Schroeder, M.A., 2003, Monitoring of greater sage-grouse habitats and populations: Moscow, Idaho, University of Idaho, College of Natural Resources Experiment Station Bulletin 80, accessed December 8, 2023, at https://doi.org/10.5962/bhl.title.153828.
Conner, M.M., Saunders, W.C., Bouwes, N., and Jordan, C., 2016, Evaluating impacts using a BACI design, ratios, and a Bayesian approach with a focus on restoration: Environmental Monitoring and Assessment, v. 188, article no. 555, 14 p. [Available at https://doi.org/10.1007/s10661-016-5526-6.]
Crawford, B.A., Maerz, J.C., and Moore, C.T., 2020, Expert-informed habitat suitability analysis for at-risk species assessment and conservation planning: Journal of Fish and Wildlife Management, v. 11, no. 1, p. 130–150. [Available at https://doi.org/10.3996/092019-JFWM-075.]
Dahlgren, D.K., Messmer, T.A., and Koons, D.N., 2010, Achieving better estimates of greater sage-grouse chick survival in Utah: The Journal of Wildlife Management, v. 74, no. 6, p. 1286–1294. [Available at https://doi.org/10.1111/j.1937-2817.2010.tb01249.x.]
Den Boer, P.J., 1968, Spreading of risk and stabilization of animal numbers: Acta Biotheoretica, v. 18, p. 165–194. [Available at https://doi.org/10.1007/BF01556726.]
Dickie, M., McNay, S.R., Sutherland, G.D., Cody, M., and Avgar, T., 2020, Corridors or risk? Movement along, and use of, linear features varies predictably among large mammal predator and prey species: Journal of Animal Ecology, v. 89, no. 2, p. 623–634. [Available at https://doi.org/10.1111/1365-2656.13130.]
Dinkins, J.B., Conover, M.R., Kirol, C.P., Beck, J.L., and Frey, S.N., 2016, Effects of common raven and coyote removal and temporal variation in climate on greater sage-grouse nesting success: Biological Conservation, v. 202, p. 50–58. [Available at https://doi.org/10.1016/j.biocon.2016.08.011.]
Doherty, K.E., Evans, J.S., Coates, P.S., Juliusson, L.M., and Fedy, B.C., 2016, Importance of regional variation in conservation planning—A rangewide example of the Greater Sage-Grouse: Ecosphere, v. 7, no. 10, 27 p. [Available at https://doi.org/10.1002/ecs2.1462.]
Doherty, K., Theobald, D.M., Bradford, J.B., Wiechman, L.A., Bedrosian, G., Boyd, C.S., Cahill, M., Coates, P.S., Creutzburg, M.K., Crist, M.R., Finn, S.P., Kumar, A.V., Littlefield, C.E., Maestas, J.D., Prentice, K.L., Prochazka, B.G., Remington, T.E., Sparklin, W.D., Tull, J.C., Wurtzebach, Z., and Zeller, K.A., 2022, A sagebrush conservation design to proactively restore America’s sagebrush biome: U.S. Geological Survey Open-File Report 2022–1081, 38 p., accessed December 6, 2023, at https://doi.org/10.3133/ofr20221081.
Donnelly, J.P., Allred, B.W., Perret, D., Silverman, N.L., Tack, J.D., Dreitz, V.J., Maestas, J.D., and Naugle, D.E., 2018, Seasonal drought in North America’s sagebrush biome structures dynamic mesic resources for sage-grouse: Ecology and Evolution, v. 8, no. 24, p. 12492–12505. [Available at https://doi.org/10.1002/ece3.4614.]
Dudko, J.E., Coates, P.S., and Delehanty, D.J., 2019, Movements of female Sage Grouse Centrocercus urophasianus during incubation recess: The Ibis, v. 161, no. 1, p. 222–229. [Available at https://doi.org/10.1111/ibi.12670.]
Dudley, I.F., Coates, P.S., Prochazka, B.G., Davis, D.M., Gardner, S.C., and Delehanty, D.J., 2022, Maladaptive nest‐site selection and reduced nest survival in female sage‐grouse following wildfire: Ecosphere, v. 13, no. 12, 13 p. [Available at https://doi.org/10.1002/ecs2.4282.]
Dudley, I.F., Coates, P.S., Prochazka, B.G., O’Neil, S.T., Gardner, S., and Delehanty, D.J., 2021, Large-scale wildfire reduces population growth in a peripheral population of sage-grouse: Fire Ecology, v. 17, article no. 15. [Available at https://doi.org/10.1186/s42408-021-00099-z.]
Duvall, A.L., Metcalf, A.L., and Coates, P.S., 2017, Conserving the greater sage-grouse—A social-ecological systems case study from the California–Nevada region: Rangeland Ecology and Management, v. 70, no. 1, p. 129–140. [Available at https://doi.org/10.1016/j.rama.2016.08.001.]
Edmunds, D.R., Aldridge, C.L., O’Donnell, M.S., and Monroe, A.P., 2018, Greater sage‐grouse population trends across Wyoming: The Journal of Wildlife Management, v. 82, no. 2, p. 397–412. [Available at https://doi.org/10.1002/jwmg.21386.]
Ehrlén, J., and Morris, W.F., 2015, Predicting changes in the distribution and abundance of species under environmental change: Ecology Letters, v. 18, no. 3, p. 303–314. [Available at https://doi.org/10.1111/ele.12410.]
Ellsworth, L.M., Kauffman, J.B., Reis, S.A., Sapsis, D., and Moseley, K., 2020, Repeated fire altered succession and increased fire behavior in basin big sagebrush–native perennial grasslands: Ecosphere, v. 11, no. 5. [Available at https://doi.org/10.1002/ecs2.3124.]
Emmons, S.R., and Braun, C.E., 1984, Lek attendance of male sage grouse: The Journal of Wildlife Management, v. 48, no. 3, p. 1023–1028. [Available at https://doi.org/10.2307/3801461.]
Evans, J.S., Oakleaf, J., Cushman, S.A., and Theobald, D., 2014, An ArcGIS toolbox for surface gradient and geomorphometric modeling, version 2.0-0: accessed January 5, 2021, at https://evansmurphy.wix.com/evansspatial.
Fedy, B.C., and Aldridge, C.L., 2011, The importance of within‐year repeated counts and the influence of scale on long‐term monitoring of sage‐grouse: The Journal of Wildlife Management, v. 75, no. 5, p. 1022–1033. [Available at https://doi.org/10.1002/jwmg.155.]
Fedy, B.C., and Doherty, K.E., 2011, Population cycles are highly correlated over long time series and large spatial scales in two unrelated species—Greater sage-grouse and cottontail rabbits: Oecologia, v. 165, no. 4, p. 915–924. [Available at https://doi.org/10.1007/s00442-010-1768-0.]
Fieberg, J.R., Forester, J.D., Street, G.M., Johnson, D.H., ArchMiller, A.A., and Matthiopoulos, J., 2018, Used‐habitat calibration plots—A new procedure for validating species distribution, resource selection, and step‐selection models: Ecography, v. 41, p. 737–752. [Available at https://doi.org/10.1111/ecog.03123.]
Flannigan, M.D., Krawchuk, M.A., de Groot, W.J., Wotton, B.M., and Gowman, L.M., 2009, Implications of changing climate for global wildland fire: International Journal of Wildland Fire, v. 18, no. 5, p. 483–507. [Available at https://doi.org/10.1071/WF08187.]
Foster, L.J., Dugger, K.M., Hagen, C.A., and Budeau, D.A., 2019, Greater sage-grouse vital rates after wildfire: The Journal of Wildlife Management, v. 83, no. 1, p. 121–134. [Available at https://doi.org/10.1002/jwmg.21573.]
Fox, J.W., Vasseur, D., Cotroneo, M., Guan, L., and Simon, F., 2017, Population extinctions can increase metapopulation persistence: Nature Ecology & Evolution, v. 1, no. 9, p. 1271–1278. [Available at https://doi.org/10.1038/s41559-017-0271-y.]
Garton, E.O., Connelly, J.W., Horne, J.S., Hagen, C.A., Moser, A., and Schroeder, M.A., 2011, Greater sage-grouse population dynamics and probability of persistence, chap. 15 in Knick, S.T., and Connelly, J.W., eds., Greater sage grouse—Ecology and conservation of a landscape species and its habitats: Berkeley, Calif., University of California Press, Studies in Avian Biology, v. 38, p. 293–381. [Available at https://doi.org/10.1525/california/9780520267114.003.0016.]
Gelbard, J.L., and Belnap, J., 2003, Roads as conduits for exotic plant invasions in a semiarid landscape: Conservation Biology, v. 17, no. 2, p. 420–432. [Available at https://doi.org/10.1046/j.1523-1739.2003.01408.x.]
Gelman, A., and Hill, J., 2006, Data analysis using regression and multilevel/hierarchical models: Cambridge University Press, 648 p. [Available at https://doi.org/10.1017/CBO9780511790942.]
Gelman, A., and Rubin, D.B., 1992, Inference from iterative simulation using multiple sequences: Statistical Science, v. 7, no. 4, p. 457–472. [Available at https://doi.org/10.1214/ss/1177011136.]
Gelman, A., Carlin, J.B., Stern, H.S., Dunson, D.B., Vehtari, A., and Rubin, D.B., 2013, Bayesian data analysis (3d ed.): New York, N.Y., Chapman and Hall/CRC, 675 p. [Available at https://doi.org/10.1201/b16018.]
Germino, M.J., Belnap, J., Stark, J.M., Allen, E.B., and Rau, B.M., 2016, Ecosystem impacts of exotic annual invaders in the genus Bromus, in Germino, M., Chambers, J.C., and Brown, C., eds., Exotic brome-grasses in arid and semiarid ecosystems of the Western US: Springer, Series on Environmental Management, p. 61–95, accessed March 25, 2022, at https://doi.org/10.1007/978-3-319-24930-8_3.
Gesch, D., Evans, G., Mauck, J., Hutchinson, J., and Carswell, W.J., Jr., 2009, The national map—Elevation: U.S. Geological Survey Fact Sheet 2009–3053, 4 p., accessed June 8, 2017, at https://doi.org/10.3133/fs20093053.
Gibson, D., Blomberg, E.J., Atamian, M.T., and Sedinger, J.S., 2016, Nesting habitat selection influences nest and early offspring survival in Greater Sage-Grouse: The Condor, v. 118, no. 4, p. 689–702. [Available at https://doi.org/10.1650/CONDOR-16-62.1.]
Gibson, D., Blomberg, E.J., Atamian, M.T., and Sedinger, J.S., 2017, Weather, habitat composition, and female behavior interact to modify offspring survival in Greater Sage-Grouse: Ecological Applications, v. 27, no. 1, p. 168–181. [Available at https://doi.org/10.1002/eap.1427.]
Gitzen, R.A., Millspaugh, J.J., and Kernohan, B.J., 2006, Bandwidth selection for fixed-kernel analysis of animal utilization distributions: The Journal of Wildlife Management, v. 70, no. 5, p. 1334–1344. [Available at https://doi.org/10.2193/0022-541X(2006)70[1334:BSFFAO]2.0.CO;2.]
Green, A.W., Aldridge, C.L., and O’Donnell, M.S., 2017, Investigating impacts of oil and gas development on greater sage‐grouse: The Journal of Wildlife Management, v. 81, no. 1, p. 46–57. [Available at https://doi.org/10.1002/jwmg.21179.]
Gustafson, K.B., Coates, P.S., Roth, C.L., Chenaille, M.P., Ricca, M.A., Sanchez-Chopitea, E., and Casazza, M.L., 2018, Using object-based image analysis to conduct high-resolution conifer extraction at regional spatial scales: International Journal of Applied Earth Observation and Geoinformation, v. 73, p. 148–155. [Available at https://doi.org/10.1016/j.jag.2018.06.002.]
Halstead, B.J., Wylie, G.D., Coates, P.S., Valcarcel, P., and Casazza, M.L., 2012, Bayesian shared frailty models for regional inference about wildlife survival: Animal Conservation, v. 15, no. 2, p. 117–124. [Available at https://doi.org/10.1111/j.1469-1795.2011.00495.x.]
Hammill, E., and Clements, C.F., 2020, Imperfect detection alters the outcome of management strategies for protected areas: Ecology Letters, v. 23, no. 4, p. 682–691. [Available at https://doi.org/10.1111/ele.13475.]
Hanser, S.E., and Knick, S.T., 2011, Greater sage-grouse as an umbrella species for shrubland passerine birds—A multiscale assessment, chap. 19 in Knick, S.T., eds., Greater sage grouse—Ecology and conservation of a landscape species and its habitats: University of California Press, p. 474–487. [Available at https://doi.org/10.1525/california/9780520267114.003.0020.]
Harju, S.M., Dzialak, M.R., Taylor, R.C., Hayden‐Wing, L.D., and Winstead, J.B., 2010, Thresholds and time lags in effects of energy development on greater sage‐grouse populations: The Journal of Wildlife Management, v. 74, no. 3, p. 437–448. [Available at https://doi.org/10.2193/2008-289.]
Hill, J.E., DeVault, T.L., and Belant, J.L., 2021, A review of ecological factors promoting road use by mammals: Mammal Review, v. 51, no. 2, p. 214–227. [Available at https://doi.org/10.1111/mam.12222.]
Holbrook, J.D., Squires, J.R., Olson, L.E., DeCesare, N.J., and Lawrence, R.L., 2017, Understanding and predicting habitat for wildlife conservation—The case of Canada lynx at the range periphery: Ecosphere, v. 8, no. 9, 25 p. [Available at https://doi.org/10.1002/ecs2.1939.]
Holloran, M.J., and Anderson, S.H., 2005, Spatial distribution of greater sage-grouse nests in relatively contiguous sagebrush habitats: The Condor, v. 107, no. 4, p. 742–752. [Available at https://doi.org/10.1093/condor/107.4.742.]
Holloran, M.J., Kaiser, R.C., and Hubert, W.A., 2010, Yearling greater sage‐grouse response to energy development in Wyoming: The Journal of Wildlife Management, v. 74, no. 1, p. 65–72. [Available at https://doi.org/10.2193/2008-291.]
Hooten, M.B., and Hobbs, N.T., 2015, A guide to Bayesian model selection for ecologists: Ecological Monographs, v. 85, no. 1, p. 3–28. [Available at https://doi.org/10.1890/14-0661.1.]
Jenni, D.A., and Hartzler, J.E., 1978, Attendance at a sage grouse lek—Implications for spring censuses: The Journal of Wildlife Management, v. 42, no. 1, p. 46–52. [Available at https://doi.org/10.2307/3800688.]
Johnson, C.J., Nielsen, S.E., Merrill, E.H., McDonald, T.L., and Boyce, M.S., 2006, Resource selection functions based on use–availability data—Theoretical motivation and evaluation methods: The Journal of Wildlife Management, v. 70, no. 2, p. 347–357. [Available at https://doi.org/10.2193/0022-541X(2006)70[347:RSFBOU]2.0.CO;2.]
Kallimanis, A.S., Kunin, W.E., Halley, J.M., and Sgardelis, S.P., 2005, Metapopulation extinction risk under spatially autocorrelated disturbance: Conservation Biology, v. 19, no. 2, p. 534–546. [Available at https://doi.org/10.1111/j.1523-1739.2005.00418.x.]
Kéry, M., 2010, Introduction to WinBUGS for ecologists—A Bayesian approach to regression, ANOVA, mixed models, and related analyses: Academic Press, 302 p. [Available at https://doi.org/10.1016/C2009-0-30639-X.]
Kéry, M., and Schaub, M., 2012, Bayesian population analysis using WinBUGS—A hierarchical perspective: Academic Press, 537 p. [Available at https://doi.org/10.1016/C2010-0-68368-4.]
Lande, R., Engen, S., and Saether, B.E., 2003, Stochastic population dynamics in ecology and conservation: Oxford University Press. [Available at https://doi.org/10.1093/acprof:oso/9780198525257.001.0001.]
Lockyer, Z.B., Coates, P.S., Casazza, M.L., Espinosa, S., and Delehanty, D.J., 2013, Greater Sage-Grouse nest predators in the Virginia Mountains of northwestern Nevada: Journal of Fish and Wildlife Management, v. 4, no. 2, p. 242–255. [Available at https://doi.org/10.3996/122012-JFWM-110R1.]
Makowski, D., Ben-Shachar, M.S., Chen, S.H.A., and Lüdecke, D., 2019, Indices of effect existence and significance in the Bayesian framework: Frontiers in Psychology, v. 10, 14 p. [Available at https://doi.org/10.3389/fpsyg.2019.02767.]
Manly, B.F.J., McDonald, L.L., Thomas, D.L., McDonald, T.L., and Erickson, W.P., 2002, Resource selection by animals—Statistical analysis and design for field studies: Springer Dordrecht, 222 p. [Available at https://doi.org/10.1007/0-306-48151-0.]
McDonald, T.L., 2013, The point process use‐availability or presence‐only likelihood and comments on analysis: Journal of Animal Ecology, v. 82, no. 6, p. 1174–1182. [Available at https://doi.org/10.1111/1365-2656.12132.]
Monroe, A.P., Wann, G.T., Aldridge, C.L., and Coates, P.S., 2019, The importance of simulation assumptions when evaluating detectability in population models: Ecosphere, v. 10, no. 7. [Available at https://doi.org/10.1002/ecs2.2791.]
Moynahan, B.J., Lindberg, M.S., Rotella, J.J., and Thomas, J.W., 2007, Factors affecting nest survival of greater sage-grouse in northcentral Montana: The Journal of Wildlife Management, v. 71, no. 6, p. 1773–1783. [Available at https://doi.org/10.2193/2005-386.]
Nevada Governor’s Sage-Grouse Conservation Team, 2004, Greater sage-grouse conservation plan for Nevada and eastern California: Nevada Department of Wildlife, California Department of Fish and Game, 108 p., accessed March 1, 2019, at https://water.nv.gov/hearings/past/Spring%20Valley%202006/exhibits/USFWS/FWS-2060/FWS-2060.pdf.
Northrup, J.M., Hooten, M.B., Anderson, C.R., Jr., and Wittemyer, G., 2013, Practical guidance on characterizing availability in resource selection functions under a use-availability design: Ecology, v. 94, no. 7, p. 1456–1463. [Available at https://doi.org/10.1890/12-1688.1.]
Noy-Meir, I., 1973, Desert ecosystems—Environment and producers: Annual Review of Ecology and Systematics, v. 4, no. 1, p. 25–51. [Available at https://doi.org/10.1146/annurev.es.04.110173.000325.]
O’Donnell, M.S., Edmunds, D.R., Aldridge, C.L., Heinrichs, J.A., Coates, P.S., Prochazka, B.G., and Hanser, S.E., 2019, Designing multi‐scale hierarchical monitoring frameworks for wildlife to support management—A sage‐grouse case study: Ecosphere, v. 10, no. 9, 34 p. [Available at https://doi.org/10.1002/ecs2.2872.
O’Donnell, M.S., Edmunds, D.R., Aldridge, C.L., Heinrichs, J.A., Monroe, A.P., Coates, P.S., Prochazka, B.G., Hanser, S.E., and Wiechman, L.A., 2022, Defining biologically relevant and hierarchically nested population units to inform wildlife management: Ecology and Evolution, v. 12, no. 12, 22 p. [Available at https://doi.org/10.1002/ece3.9565.]
O’Donnell, M.S., Edmunds, D.R., Aldridge, C.L., Heinrichs, J.A., Monroe, A.P., Coates, P.S., Prochazka, B.G., Hanser, S.E., Wiechman, L.A., Christiansen, T.J., Cook, A.A., Espinosa, S.P., Foster, L.J., Griffin, K.A., Kolar, J.L., Miller, K.S., Moser, A.M., Remington, T.E., Runia, T.J., Schreiber, L.A., Schroeder, M.A., Stiver, S.J., Whitford, N.I., and Wightman, C.S., 2021, Synthesizing and analyzing long-term monitoring data—A greater sage-grouse case study: Ecological Informatics, v. 63, 16 p. [Available at https://doi.org/10.1016/j.ecoinf.2021.101327.]
Olsen, A.C., Severson, J.P., Maestas, J.D., Naugle, D.E., Smith, J.T., Tack, J.D., Yates, K.H., and Hagen, C.A., 2021, Reversing tree expansion in sagebrush steppe yields population‐level benefit for imperiled grouse: Ecosphere, v. 12, no. 6, 17 p. [Available at https://doi.org/10.1002/ecs2.3551.]
O’Neil, S.T., Coates, P.S., Brussee, B.E., Ricca, M.A., Espinosa, S.P., Gardner, S., and Delehanty, D.J., 2020, Wildfire and the ecological niche—Diminishing habitat suitability for an indicator species within semi-arid ecosystems: Global Change Biology, v. 26, no. 11, p. 6296–6312. [Available at https://doi.org/10.1111/gcb.15300.]
Oyler-McCance, S.J., Casazza, M.L., Fike, J.A., and Coates, P.S., 2014, Hierarchical spatial genetic structure in a distinct population segment of greater sage-grouse: Conservation Genetics, v. 15, no. 6, p. 1299–1311. [Available at https://doi.org/10.1007/s10592-014-0618-8.]
Oyler-McCance, S.J., Cornman, R.S., Jones, K.L., and Fike, J.A., 2015, Genomic single-nucleotide polymorphisms confirm that Gunnison and Greater sage-grouse are genetically well differentiated and that the Bi-State population is distinct: The Condor, v. 117, no. 2, p. 217–227. [Available at https://doi.org/10.1650/CONDOR-14-174.1.]
Park, T., and Casella, G., 2008, The Bayesian lasso: Journal of the American Statistical Association, v. 103, no. 482, p. 681–686. [Available at https://doi.org/10.1198/016214508000000337.]
Picardi, S., Coates, P.S., Kolar, J., O’Neil, S.T., Mathews, S., and Dahlgren, D., 2022, Behavioural state-dependent habitat selection and implications for animal translocations: Journal of Applied Ecology, v. 59, no. 2, p. 624–635. [Available at https://doi.org/10.1111/1365-2664.14080.]
Pilliod, D.S., Welty, J.L., and Arkle, R.S., 2017, Refining the cheatgrass-fire cycle in the Great Basin—Precipitation timing and fine fuel composition predict wildfire trends: Ecology and Evolution, v. 7, no. 19, p. 8126–8151. [Available at https://doi.org/10.1002/ece3.3414.]
Plummer, M., 2016, JAGS version 4.3: Source Forge, accessed January 12, 2021, at https://mcmc-jags.sourceforge.io/.
Poessel, S.A., Barnard, D.M., Applestein, C., Germino, M.J., Ellsworth, E.A., Major, D., Moser, A., and Katzner, T.E., 2022, Greater sage‐grouse respond positively to intensive post‐fire restoration treatments: Ecology and Evolution, v. 12, no. 3, 13 p. [Available at https://doi.org/10.1002/ece3.8671.]
Pratt, A.C., and Beck, J.L., 2021, Do greater sage‐grouse exhibit maladaptive habitat selection?: Ecosphere, v. 12, no. 3, 22 p. [Available at https://doi.org/10.1002/ecs2.3354.]
Prochazka, B.G., Coates, P.S., O’Donnell, M.S., Edmunds, D.R., Monroe, A.P., Ricca, M.A., Wann, G.T., Hanser, S.E., Wiechman, L.A., Doherty, K.E., Chenaille, M.P., and Aldridge, C.L., 2023, A targeted annual warning system developed for the conservation of a sagebrush indicator species: Ecological Indicators, v. 148, 13 p. [Available at https://doi.org/10.1016/j.ecolind.2023.110097.]
Prochazka, B.G., Coates, P.S., Ricca, M.A., Casazza, M.L., Gustafson, K.B., and Hull, J.M., 2017, Encounters with pinyon-juniper influence riskier movements in greater sage-grouse across the Great Basin: Rangeland Ecology and Management, v. 70, no. 1, p. 39–49. [Available at https://doi.org/10.1016/j.rama.2016.07.004.]
Pyke, D.A., Shriver, R.K., Arkle, R.S., Pilliod, D.S., Aldridge, C.L., Coates, P.S., Germino, M.J., Heinrichs, J.A., Ricca, M.A., and Shaff, S.E., 2020, Postfire growth of seeded and planted big sagebrush—Strategic designs for restoring greater sage‐grouse nesting habitat: Restoration Ecology, v. 28, no. 6, p. 1495–1504. [Available at https://doi.org/10.1111/rec.13264.]
Quinn, J.F., and Hastings, A., 1987, Extinction in subdivided habitats: Conservation Biology, v. 1, no. 3, p. 198–209. [Available at https://doi.org/10.1111/j.1523-1739.1987.tb00033.x.]
R Core Team, 2022, R—A language and environment for statistical computing: Vienna, Austria, R Foundation for Statistical Computing, accessed March 3, 2022, at http://www.r-project.org/.
Ricca, M.A., and Coates, P.S., 2020, Integrating ecosystem resilience and resistance into decision support tools for multi-scale population management of a sagebrush indicator species: Frontiers in Ecology and Evolution, v. 7, 22 p. [Available at https://doi.org/10.3389/fevo.2019.00493.]
Ricca, M.A., Coates, P.S., Gustafson, K.B., Brussee, B.E., Chambers, J.C., Espinosa, S.P., Gardner, S.C., Lisius, S., Ziegler, P., Delehanty, D.J., and Casazza, M.L., 2018, A conservation planning tool for Greater Sage‐grouse using indices of species distribution, resilience, and resistance: Ecological Applications, v. 28, no. 4, p. 878–896. [Available at https://doi.org/10.1002/eap.1690.]
Rigge, M., Homer, C., Cleeves, L., Meyer, D.K., Bunde, B., Shi, H., Xian, G., Schell, S., and Bobo, M., 2020, Quantifying western U.S. rangelands as fractional components with multi-resolution remote sensing and in situ data: Remote Sensing (Basel), v. 12, no. 3, 26 p. [Available at https://doi.org/10.3390/rs12030412.]
Rigge, M., Homer, C., Shi, H., Meyer, D., Bunde, B., Granneman, B., Postma, K., Danielson, P., Case, A., and Xian, G., 2021, Rangeland fractional components across the western United States from 1985 to 2018: Remote Sensing (Basel), v. 13, no. 4, p. 813, 24 p. [Available at https://doi.org/10.3390/rs13040813.]
Roth, C.L., O’Neil, S.T., Coates, P.S., Ricca, M.A., Pyke, D.A., Aldridge, C.L., Heinrichs, J.A., Espinosa, S.P., and Delehanty, D.J., 2022, Targeting sagebrush (Artemisia Spp.) restoration following wildfire with Greater Sage-Grouse (Centrocercus Urophasianus): Environmental Management, v. 70, no. 2, p. 288–306. [Available at https://doi.org/10.1007/s00267-022-01649-0.]
Royle, J.A., and Dorazio, R.M., 2009, Hierarchical modeling and inference in ecology—The analysis of data from populations, metapopulations, and communities: San Diego, Calif., Academic Press, 444 p. [Available at https://doi.org/10.1016/B978-0-12-374097-7.50001-5.]
Saher, D.J., O’Donnell, M.S., Aldridge, C.L., and Heinrichs, J.A., 2022, Balancing model generality and specificity in management-focused habitat selection models for Gunnison sage-grouse: Global Ecology and Conservation, v. 35, 21 p. [Available at https://doi.org/10.1016/j.gecco.2021.e01935.]
Sandford, C.P., Kohl, M.T., Messmer, T.A., Dahlgren, D.K., Cook, A., and Wing, B.R., 2017, Greater sage-grouse resource selection drives reproductive fitness under a conifer removal strategy: Rangeland Ecology and Management, v. 70, no. 1, p. 59–67. [Available at https://doi.org/10.1016/j.rama.2016.09.002.]
Schaub, M., and Abadi, F., 2011, Integrated population models—A novel analysis framework for deeper insights into population dynamics: Journal of Ornithology, v. 152, p. 227–237. [Available at https://doi.org/10.1007/s10336-010-0632-7.]
Schmidt, J.H., Walker, J.A., Lindberg, M.S., Johnson, D.S., and Stephens, S.E., 2010, A general Bayesian hierarchical model for estimating survival of nests and young: The Auk, v. 127, no. 2, p. 379–386. [Available at https://doi.org/10.1525/auk.2009.09015.]
Schroeder, M.A., Aldridge, C.L., Apa, A.D., Bohne, J.R., Braun, C.E., Bunnell, S.D., Connelly, J.W., Deibert, P.A., Gardner, S.C., Hilliard, M.A., Kobriger, G.D., McAdam, S.M., McCarthy, C.W., McCarthy, J.J., Mitchell, D.L., Rickerson, E.V., and Stiver, S.J., 2004, Distribution of sage-grouse in North America: The Condor, v. 106, no. 2, p. 363–376. [Available at https://doi.org/10.1093/condor/106.2.363.]
Severson, J.P., Coates, P.S., Milligan, M.C., O’Neil, S.T., Ricca, M.A., Abele, S.C., Boone, J.D., and Casazza, M.L., 2022, Moisture abundance and proximity mediate seasonal use of mesic areas and survival of greater sage‐grouse broods: Ecological Solutions and Evidence, v. 3, no. 4, 14 p. [Available at https://doi.org/10.1002/2688-8319.12194.]
Severson, J.P., Coates, P.S., Prochazka, B.G., Ricca, M.A., Casazza, M.L., and Delehanty, D.J., 2019, Global positioning system tracking devices can decrease Greater Sage-Grouse survival: The Condor, v. 121, no. 3, 15 p. [Available at https://doi.org/10.1093/condor/duz032.]
Severson, J.P., Hagen, C.A., Maestas, J.D., Naugle, D.E., Forbes, J.T., and Reese, K.P., 2017, Effects of conifer expansion on greater sage‐grouse nesting habitat selection: The Journal of Wildlife Management, v. 81, no. 1, p. 86–95. [Available at https://doi.org/10.1002/jwmg.21183.]
Shaffer, T.L., 2004, A unified approach to analyzing nest success: The Auk, v. 121, no. 2, p. 526–540. [Available at https://www.jstor.org/stable/4090416.]
Sinnott, E.A., Thompson, F.R., III, Weegman, M.D., and Thompson, T.R., 2022, Northern Bobwhite juvenile survival is greater in native grasslands managed with fire and grazing and lower in non-native field borders and strip crop fields: The Condor, v. 124, no. 1, 15 p. [Available at https://doi.org/10.1093/ornithapp/duab057.]
Smith, K.T., and Beck, J.L., 2018, Sagebrush treatments influence annual population change for greater sage‐grouse: Restoration Ecology, v. 26, no. 3, p. 497–505. [Available at https://doi.org/10.1111/rec.12589.]
Stephens, P.A., Pettorelli, N., Barlow, J., Whittingham, M.J., and Cadotte, M.W., 2015, Management by proxy? The use of indices in applied ecology: Journal of Applied Ecology, v. 52, no. 1, p. 1–6. [Available at https://doi.org/10.1111/1365-2664.12383.]
Stevens, B.S., Connelly, J.W., and Reese, K.P., 2012, Multi‐scale assessment of greater sage‐grouse fence collision as a function of site and broad scale factors: The Journal of Wildlife Management, v. 76, no. 7, p. 1370–1380. [Available at https://doi.org/10.1002/jwmg.397.]
Stuber, E.F., and Fontaine, J.J., 2019, How characteristic is the species characteristic selection scale?: Global Ecology and Biogeography, v. 28, no. 12, p. 1839–1854. [Available at https://doi.org/10.1111/geb.12998.]
Stuber, E.F., Gruber, L.F., and Fontaine, J.J., 2017, A Bayesian method for assessing multi-scale species-habitat relationships: Landscape Ecology, v. 32, no. 12, p. 2365–2381. [Available at https://doi.org/10.1007/s10980-017-0575-y.]
Su, Y.S., and Yajima, M., 2015, R2jags—Using R to run ‘JAGS’, version 0.6-1: The R Project, accessed January 12, 2021, at https://cran.r-project.org/web//packages/R2jags/.
Taylor, R.L., Walker, B.L., Naugle, D.E., and Mills, L.S., 2012, Managing multiple vital rates to maximize greater sage-grouse population growth: The Journal of Wildlife Management, v. 76, no. 2, p. 336–347. [Available at https://doi.org/10.1002/jwmg.267.]
Thiault, L., Kernaléguen, L., Osenberg, C.W., and Claudet, J., 2017, Progressive‐change BACIPS—A flexible approach for environmental impact assessment: Methods in Ecology and Evolution, v. 8, no. 3, p. 288–296. [Available at https://doi.org/10.1111/2041-210X.12655.]
Tyrrell, E.A., Coates, P.S., Prochazka, B.G., Brussee, B.E., Espinosa, S.P., and Hull, J.M., 2023, Wildfire immediately reduces nest and adult survival of greater sage-grouse: Scientific Reports, v. 13, article no. 10970, 12 p. [Available at https://doi.org/10.1038/s41598-023-32937-2.]
U.S. Fish and Wildlife Service, 2002, Endangered and threatened wildlife and plants; 90-day finding on a petition to list the Mono Basin area sage grouse as endangered: Federal Register, v. 67, no. 248, p. 78811–78815, accessed June 21, 2023, at https://www.govinfo.gov/content/pkg/FR-2002-12-26/pdf/FR-2002-12-26.pdf.
U.S. Fish and Wildlife Service, 2006, Endangered and threatened wildlife and plants; 90-day finding on petitions to list the Mono Basin area population of the greater sage-grouse as threatened or endangered: Federal Register, v. 71, no. 243, p. 76058–76078, accessed June 21, 2023, at https://www.govinfo.gov/content/pkg/FR-2006-12-19/pdf/E6-21135.pdf.
U.S. Fish and Wildlife Service, 2008, Endangered and threatened wildlife and plants; 90-day finding on petitions to list the Mono Basin area population of greater sage-grouse (Centrocercus urophasianus) as threatened or endangered: Federal Register, v. 73, no. 83, p. 23173–23175, accessed June 21, 2023, at https://www.govinfo.gov/content/pkg/FR-2008-04-29/pdf/E8-9185.pdf.
U.S. Fish and Wildlife Service, 2010, Endangered and threatened wildlife and plants; 12-month findings for petitions to list the greater sage-grouse (Centrocercus urophasianus) as threatened or endangered: Federal Register, v. 75, no. 55, p. 13910–14014, accessed June 21, 2023, at https://www.federalregister.gov/documents/2010/03/23/2010-5132/endangered-and-threatened-wildlife-and-plants-12-month-findings-for-petitions-to-list-t he-greater.
U.S. Fish and Wildlife Service, 2013, Endangered and threatened wildlife and plants; designation of critical habitat for the Bi-State Distinct Population Segment of greater sage-grouse: Federal Register, v. 78, no. 208, p. 64328–64355, accessed June 21, 2023, at https://www.govinfo.gov/content/pkg/FR-2013-10-28/pdf/2013-24305.pdf.
U.S. Fish and Wildlife Service, 2015, Endangered and threatened wildlife and plants; withdrawal of proposed rule to list the Bi-State Distinct Population Segment of greater sage-grouse and designate critical habitat: Federal Register, v. 80, no. 78, p. 22827–22866, accessed June 22, 2023, at https://www.govinfo.gov/content/pkg/FR-2015-04-23/pdf/2015-09417.pdf.
U.S. Fish and Wildlife Service, 2019, Endangered and threatened wildlife and plants; threatened status for the Bi-State Distinct Population Segment of greater sage-grouse with section 4(d) rule and designation of critical habitat: Federal Register, v. 84, no. 71, p. 14909–14910, accessed June 22, 2023, at https://www.govinfo.gov/content/pkg/FR-2019-04-12/pdf/2019-07252.pdf.
U.S. Fish and Wildlife Service, 2020, Endangered and threatened wildlife and plants; withdrawal of the proposed rules to list the Bi-State Distinct Population Segment of greater sage-grouse with section 4(d) rule and to designate critical habitat: Federal Register, v. 85, no. 62, p. 18054–18099, accessed June 22, 2023, at https://www.govinfo.gov/content/pkg/FR-2020-03-31/pdf/2020-06384.pdf.
U.S. Fish and Wildlife Service, 2022, National wetlands inventory: U.S. Fish and Wildlife Service web page, accessed January 13, 2021, at https://www.fws.gov/program/national-wetlands-inventory/.
U.S. Fish and Wildlife Service, 2023, Endangered and threatened wildlife and plants; threatened status for the Bi-State Distinct Population Segment of greater sage-grouse with section 4(d) rule and designation of critical habitat: Federal Register, v. 88, no. 81, p. 25613–25616, accessed June 20, 2023, at https://www.govinfo.gov/content/pkg/FR-2023-04-27/pdf/2023-08848.pdf.
U.S. Geological Survey, 2017, National hydrography dataset: U.S. Geological Survey web page, accessed December 23, 2019, at https://www.usgs.gov/national-hydrography/national-hydrography-dataset.
U.S. Geological Survey, 2023, Conservation efforts database: U.S. Geological Survey web page, accessed January 5, 2023, at https://conservationefforts.org/sgce/home/.
Van Lanen, N.J., Green, A.W., Gorman, T.R., Quattrini, L.A., and Pavlacky, D.C., Jr., 2017, Evaluating efficacy of fence markers in reducing greater sage-grouse collisions with fencing: Biological Conservation, v. 213, part A, p. 70–83. [Available at https://doi.org/10.1016/j.biocon.2017.06.030.]
Walker, B.L., Naugle, D.E., and Doherty, K.E., 2007, Greater sage‐grouse population response to energy development and habitat loss: The Journal of Wildlife Management, v. 71, no. 8, p. 2644–2654. [Available at https://doi.org/10.2193/2006-529.]
Wann, G.T., Coates, P.S., Prochazka, B.G., Severson, J.P., Monroe, A.P., and Aldridge, C.L., 2019, Assessing lek attendance of male greater sage‐grouse using fine‐resolution GPS data—Implications for population monitoring of lek mating grouse: Population Ecology, v. 61, no. 2, p. 183–197. [Available at https://doi.org/10.1002/1438-390X.1019.]
Western Association of Fish and Wildlife Agencies, 2015, Greater sage-grouse population trends: an analysis of lek count databases 1965–2015: Cheyenne, Wyo., Western Association of Fish and Wildlife Agencies, 55 p., accessed July 12, 2023, at https://ir.library.oregonstate.edu/concern/technical_reports/ng451p621.
White, E.R., Baskett, M.L., and Hastings, A., 2021, Catastrophes, connectivity and Allee effects in the design of marine reserve networks: Oikos, v. 130, no. 3, p. 366–376. [Available at https://doi.org/10.1111/oik.07770.]
Wisdom, M.J., Rowland, M.M., and Suring, L.H., eds., 2005, Habitat threats in the sagebrush ecosystem—Methods of regional assessment and applications in the Great Basin: Lawrence, Kans., Alliance Communications Group, 301 p., accessed July 13, 2023, at https://www.fs.usda.gov/pnw/pubs/Habitat-Threats-in-the-Sagebrush-Ecosystem.pdf.
Zimmerman, S.J., Aldridge, C.L., O’Donnell, M.S., Edmunds, D.R., Coates, P.S., Prochazka, B.G., Fike, J.A., Cross, T.B., Fedy, B.C., and Oyler-McCance, S.J., 2023, A genetic warning system for a hierarchically structured wildlife monitoring framework: Ecological Applications, v. 33, no. 3, 18 p. [Available at https://doi.org/10.1002/eap.2787.]
Appendix 1. Results of Variable Selection AnalysesAppendix 1
Table 1.1.
Candidate landscape covariates for habitat selection and survival models of greater sage-grouse in the Bi-State Distinct Population Segment from 2003 to 2019 for nest, early, and late brood-rearing periods, selected from a preliminary Bayesian latent indicator variable selection procedure, where covariates with greater proportion of the posterior distribution were selected over other grouped covariates.[%, percent; m, meters; —, not relevant; cm, centimeters; km2, square kilometers; km, kilometer]
Table 1.2.
Candidate landscape covariates for habitat selection models of greater sage-grouse in the Bi-State Distinct Population Segment from 2003 to 2019 for spring, summer, and winter, selected from a preliminary Bayesian latent indicator variable selection procedure, where covariates with greater proportion of the posterior distribution were selected over other grouped covariates.[%, percent; m, meters; cm, centimeters; km2, square kilometers; km, kilometers]
Appendix 2. Habitat Changes Over Time in Bi-State Distinct Population SegmentAppendix 2.
Table 2.1.
Percentage changes in area for each subpopulation and the Bi-State Distinct Population Segment overall from previous population nadirs (1995, 2001, and 2008) to the most recent nadir (2021) based on composite selection, survival, and habitat suitability layers.[Area was calculated based on categorized layers after excluding the lowest category (that is, non-habitat for selection layers and very low survival for survival layers)]
Table 2.2.
Percentage changes in median index value for each subpopulation and the Bi-State Distinct Population Segment overall from previous population nadirs (1995, 2001, and 2008) to the most recent nadir (2021) based on composite selection, survival, and habitat suitability layers.[The median index value was calculated based on continuous layers for selection, survival, or suitability]
Table 2.3.
Percentage changes in area for each subpopulation and the Bi-State Distinct Population Segment overall from previous population nadirs (1995, 2001, and 2008) to the most recent nadir (2021) based on selection layers for each life stage and season.[Area was calculated based on categorized layers after excluding the lowest category (that is, non-habitat)]
Table 2.4.
Percentage changes in median index value for each subpopulation and the Bi-State Distinct Population Segment overall from previous population nadirs (1995, 2001, and 2008) to the most recent nadir (2021) based on selection layers for each life stage and season.[The median index value was calculated based on continuous selection layers]
Table 2.5.
Percentage changes in area for each subpopulation and the Bi-State Distinct Population Segment overall from previous population nadirs (1995, 2001, and 2008) to the most recent nadir (2021) based on survival layers for each life stage.[Area was calculated based on categorized layers after excluding the lowest category (that is, very low survival)]
Table 2.6.
Percentage changes in median index value for each subpopulation and the Bi-State Distinct Population Segment overall from previous population nadirs (1995, 2001, and 2008) to the most recent nadir (2021) based on survival layers for each life stage.[The median index value was calculated based on continuous survival layers]
Table 2.7.
Percentage changes in distribution for each subpopulation and the Bi-State Distinct Population Segment overall from previous population nadirs (1995, 2001, and 2008) to the most recent nadir (2021).[Distribution was calculated as the intersection between the composite selection index and the abundance and space use index]
Abbreviations
~
approximately
λ
finite rate of population change
estimated rate of population change
estimated intrinsic rate of population change
N
abundance
estimated abundance
ASUI
abundance and space use index
BACI
before-after-control-impact
BACIPS
before-after-control-impact paired-series
BLISS
Bayesian latent indicator scale selection
BLM
Bureau of Land Management
BSTAC
Bi-State Technical Advisory Committee
CE
conservation effort
CED
Conservation Efforts Database
CH
cumulative hazard
CI
confidence interval
CL1
clutch size 1
CL2
clutch size 2
CRI
credible interval
CTI
compound topographic index
DEM
digital elevation model
DPS
Distinct Population Segment
DS
daily survival probability
ESA
Endangered Species Act
GNLCC
Great Northern Landscape Conservation Cooperative
GPS
Global positioning system
HATCH
hatchability
HD/IR
high-definition infrared
HLI
heat load index
HSI
habitat selection index
IPM
integrated population model
LAWG
Local Area Working Group
MCMC
Markov Chain Monte Carlo
NP1
nest propensity 1
NS1
nest survival 1
NS2
nest survival 2
PC BACIPS
Progressive-Change before-after-control-impact paired-series
PMU
population management unit
QA/QC
quality assurance/quality control
R&R
resilience and resistance
RAP
Rangeland Analysis Platform
RCMAP
Rangeland Condition Monitoring Assessment and Projection
RSF
resource selection function
RSS
relative selection strength
SD
standard deviation
SSM
state-space model
TAC
Technical Advisory Committee
TRASP
transformed aspect
UHC
used-habitat calibration
USFWS
U.S. Fish and Wildlife Service
USGS
U.S. Geological Survey
VHF
very high frequency
WERC
Western Ecological Research Center
For more information concerning the research in this report, contact the
Director, Western Ecological Research Center
U.S. Geological Survey
3020 State University Drive East
Sacramento, California 95819
https://www.usgs.gov/centers/werc
Publishing support provided by the Science Publishing Network,
Sacramento Publishing Service Center
Disclaimers
Any use of trade, firm, or product names is for descriptive purposes only and does not imply endorsement by the U.S. Government.
Although this information product, for the most part, is in the public domain, it also may contain copyrighted materials as noted in the text. Permission to reproduce copyrighted items must be secured from the copyright owner.
Suggested Citation
Coates, P.S., Milligan, M.C., Prochazka, B.G., Brussee, B.E., O’Neil, S.T., Lundblad, C.G., Webster, S.C., Weise, C.L., Mathews, S.R., Chenaille, M.P., Aldridge, C.L., O’Donnell, M.S., Espinosa, S.P., Sturgill, A.C., Doherty, K.E., Tull, J.C., Miller, K., Wiechman, L.A., Abele, S., Boone, J., Stone, H., and Casazza, M.L., 2024, Status of greater sage-grouse in the Bi-State Distinct Population Segment—An evaluation of population trends, habitat selection, and efficacy of conservation actions: U.S. Geological Survey Open-File Report 2024–1030, 74 p., https://doi.org/10.3133/ofr20241030.
ISSN: 2331-1258 (online)
Study Area
Publication type | Report |
---|---|
Publication Subtype | USGS Numbered Series |
Title | Status of greater sage-grouse in the Bi-State Distinct Population Segment—An evaluation of population trends, habitat selection, and efficacy of conservation actions |
Series title | Open-File Report |
Series number | 2024-1030 |
DOI | 10.3133/ofr20241030 |
Year Published | 2024 |
Language | English |
Publisher | U.S. Geological Survey |
Publisher location | Reston, VA |
Contributing office(s) | Western Ecological Research Center |
Description | Report: x, 74 p.; 2 Data Releases |
Country | United States |
State | California, Nevada |
Online Only (Y/N) | Y |
Google Analytic Metrics | Metrics page |