High-flow experimental outcomes to inform Everglades restoration, 2010–22
Links
- Document: Report (5.4 MB pdf) , HTML , XML
- Data Releases:
- USGS Data Release - Biophysical Data for Simulating Overland Flow in the Everglades
- USGS Data Release - High-Flow Field Experiments to Inform Everglades Restoration: Experimental Data 2010 to 2022 (ver. 2.0, October 2023)
- USGS Data Release - High-flow Experimental Outcomes for Everglades Hydraulics and Aquatic Metabolism
- Download citation as: RIS | Dublin Core
Acknowledgments
The research was supported by the U.S. Geological Survey (USGS) Greater Everglades Priority Ecosystems Studies (GEPES) Program and by funding from the U.S. Army Corps of Engineers (agreement USACE-USGS MIPR W32CS510826251) and funding from the South Florida Water Management District (SFWMD; agreements USGS_JFA0113-SFWMD_19MCJFA0113, and USGS_20HWCOLL0003-SFWMD_4600004249). We acknowledge the inspiration and great work of the many scientists from other agencies and academic institutions who conducted complementary projects at Decompartmentalization Physical Model, which informed this report. This report covers the parts of the project work at the Decompartmentalization Physical Model site that were led by the USGS, and the authors assume the responsibility for its content. The authors acknowledge assistance ranging from scientific advice to methods development to field assistance provided by USGS scientists Greg Noe, Karl Hasse, Brendan Buskirk, Jenny Lewis, Jordan Psaltakis, Alex Walker, Jay Sah, Geoff Sinclair, Lars Soderqvist, Travis Knight, Mark Zucker, Corey Whittaker, and Adam Hines. A big appreciation goes to the present and former staff of the USGS Caribbean-Florida Water Science Center Data Section, under leadership of Eduardo Patino and Mark Dickman, and the USGS Data Section scientists who provided outstanding airboat and logistical support: Jeff Woods, Eric Carlson, Corey Whittaker, Chaney Adrianza, Adam Hines, and Mike Oliver. We express additional appreciation to many colleagues across multiple agencies and academic institutions that freely discussed ideas about Everglades restoration: Nick Aumen and Barry Rosen (USGS); Fred Sklar, Colin Saunders, Sue Newman, Christa Zweig, Eric Tate-Boldt, Lisa Jackson, Carlos Coronado, Michael Manna, Fabiola Santamaria, and Eric Cline (SFWMD); David Ho (University of Hawai‘i); Aaron Hurst (University of California, Berkeley); and Joel Trexler, Mike Ross, Rudolf Jaffee, Peter Regier, Nate Dorn, Chris Hansen, Mike Bush, and Alex Ontkos (Florida International University). We also appreciate the helpful review comments provided by SFWMD scientists Colin Saunders, Sue Newman, Fabiola Santamaria, and Christa Zweig. Editorial assistance was provided by Kathryn Pauls.
Abstract
The Decompartmentalization Physical Model (DPM) was an experimental facility in the central Everglades operated between 2010 and 2022 to release high flows through a levee-enclosed area of degraded ridge and slough wetland that had been isolated from flow for sixty years. The purpose of DPM experimental program was to make measurements before, during, and after seasonal high-flow releases that could help guide the Congressionally authorized Everglades restoration project known as the Decompartmentalization and Sheet Flow Enhancement Project.
The DPM facility was operated by the South Florida Water Management District, with the U.S. Geological Survey (USGS) and several universities participating in experimental design and leading aspects of the research. The USGS research at DPM focused on measuring high-flow hydraulics and its sedimentary and ecological responses in downstream wetlands. USGS investigated interactions between flow and vegetation and microtopography that influenced flow velocity and water depth, bed shear stress, sediment entrainment, and the resulting downstream transport of suspended sediment and fate of particle-associated phosphorus. USGS also investigated high-flow changes in water-column mixing and gas exchange and resulting effects on metabolism of the aquatic ecosystem (primary productivity and respiration). USGS also investigated effects of built structures such as levee gaps that were constructed to reconnect levee-enclosed basins. This report describes the methods and results of the USGS-led data collection at DPM.
The USGS studies at DPM have identified factors that influence effectiveness of restoration, specifically how high-flow releases maximize sheet flow and affect sediment and nutrient dynamics while minimizing undesirable outcomes caused by past management that bypassed wetlands by conveying polluted water through canals to ecologically sensitive downstream areas. The DPM high-flow experiments reconnected the Water Conservation Area 3A and Water Conservation Area 3B basins, and it therefore has become a central feature of the restoration’s Decompartmentalization and Sheet Flow Enhancement Project. DPM’s scientific findings have already influenced the adaptive management of Everglades restoration in guiding elements of the final design and implementation of the Central Everglades Planning Project-South. In addition to serving Everglades restoration, the DPM has the potential to influence similar adaptive management programs throughout the nation’s network of federal and state-managed river corridors, floodplains, and riparian ecosystems.
Introduction
The Everglades was once an expansive subtropical ecosystem comprised of hydrologically interconnected cypress forests, sawgrass sloughs, marl prairies, and mangrove estuaries encompassing more than 3.6 million hectares (ha; Davis and others, 1994). These primary landscape features were physically defined by oligotrophic waters, low topographic relief, large spatial extent, seasonally dynamic rainfall and surface water patterns, hydrologic gradients and sheet flow, and an estuarine system (Ogden and others, 1999). The natural system was a floodplain with unconstrained sheet flow across well-connected deep-water sloughs between slightly higher and more densely vegetated sawgrass ridges. These features supported great populations of wading birds with foraging and nesting ranges that were tightly coupled with hydropatterns.
Over the last century, the Everglades ecosystem has been degraded by the unintended effects of water management and by spillover effects of agricultural and urban development. A vast network of canals, levees, control structures, and conservation areas was built to drain the Everglades and control floods and later the system was adjusted to store water for domestic supply and to meet agricultural and industrial needs. The largest of these projects was the Central and Southern Florida Project constructed during the 1950s–1970s (Light and Dineen, 1994) which sought to manage the hydrology of a wetland system in which the flow quantity, timing, and distribution had been substantially altered. Unintended side effects included degradation of functions and habitats for over more than 50 percent of the aerial extent of the Everglades, including substantial subsidence of peat in many areas, and the creation of a highly compartmentalized flow system with excessive drying in the northern areas and in the southern area comprised of Everglades National Park (Harvey and others, 2017) as well as eutrophication of wetlands at points of canal inflows (Hagerthey and others, 2008). An additional unintended side effect of water management has been an impairment of water quality leading to increased eutrophication in the St. Lucie and Caloosahatchee estuaries where stormwater has been routed from Lake Okeechobee rather than conveying it southward through the Everglades.
The 7 million people that inhabit southeast Florida are directly dependent on water management for flood control, water supply, irrigation, and economic values of recreation and tourism. Furthermore, Congress has supported preservation and restoration of the Everglades in ways that support human needs but also in ways that recognize the Everglades’ unique status as a wetland of international significance designated at the 1971 Ramsar convention on wetlands, and its status as a major National Park. Management of the Everglades and its resources therefore strikes a balance between the needs of the ecosystem with many societal interests; thus, an adaptive management approach is being implemented (Ogden and others, 2005). Key to the plan is the use of “best available science” and “adaptive assessment” which ensures plan flexibility to allow for modification as new information is obtained (Ogden and others, 2003). An “applied science strategy” has also been developed to incorporate science into the planning and implementation of water management (for example, Ogden and others, 2003, 2005).
Over the last four decades, a wealth of scientific knowledge has been gained about the Everglades and the south Florida natural system. This information has been synthesized into conceptual models that identify the major anthropogenic drivers and stressors, the ecological effect of these stressors, and the best biological attributes or indicators of these ecological responses for the primary landscape features of south Florida (see Ogden and others, 2005). The primary landscape types are the Everglades ridge and slough landscape (Ogden and others, 2005), southern marl prairies (Davis and others, 2005a), Everglades mangrove estuaries (Davis and others, 2005b), and Florida Bay (Rudnick and others, 2005). The Everglades ridge and slough conceptual model identifies loss of sheet flow and shortened hydroperiods in some areas and ponding in others. The underlying causes include the reduced water storage capacity of the Everglades and the increased compartmentalization with levees and canals. The disruption of sheet flow is the least understood stressor (Science Coordination Team, 2003) because there is no modern-day analog in which to easily evaluate how the higher levels of sheet flow, prevalent more than a century ago, influenced sediment and nutrient transport, or how flow created and maintained a bimodal landscape with subtle topographic differences that simultaneously support productive open water habitats and densely vegetated refugia. Prior to drainage (referred to as the pre-drainage Everglades) the system was well adapted for droughts by storing and slowly releasing water from deep-water sloughs while also retaining a capacity to convey episodic floods with only a modest rise in water levels that does no permanent harm to the landscape. The well-connected slough habitats promoted dispersal of animals and, during seasonal dry downs, concentrating fish and invertebrates in pools that supported wood storks and other consumers during the critical dry season. The managed areas of the Everglades have lost much of those functions of drought water storage and flood conveyance, as well as the high-quality habitats that support extraordinary wildlife resources. Loss of microtopography and homogenization of vegetation, for example, now threatens many ecosystem functions typified by the loss of the iconic super colonies of wading birds that the pre-drainage Everglades supported. Strategies to recover lost functions depend on strong environmental monitoring programs and scientifically based investigations that work hand in hand with Congressionally authorized adaptive management of the water and ecological resources.
Origins and Objectives of the DPM Project
The Decompartmentalization Physical Model (DPM) was an experimental facility in the heart of the Everglades where high-flow field releases were conducted for almost a decade in an area that has been isolated from flow for sixty years (fig. 1). The purpose of the DPM experiments was to provide scientific information to help guide the Congressionally authorized Everglades restoration project known as the Comprehensive Everglades Restoration Plan (CERP). CERP outlines an Everglades-wide restoration project to protect internationally valued wildlife resources and the potable water supply for southern Floridians while also increasing resilience to droughts and floods. The DPM high flow experiments specifically addressed one of CERP’s centerpiece projects, the Decompartmentalization and Sheet Flow Enhancement Project (DECOMP), through a process of scientifically based adaptive management. Because the DPM high-flow experiments measured the results of reconnecting the Water Conservation Area 3 basins (WCA 3A and WCA 3B), a central feature of DECOMP, even the preliminary results from DPM influenced the restoration’s final planning and initial implementation as part of the Central Everglades Planning Project (CEPP-S) in the southern Everglades.
DPM has identified factors that influence effectiveness of restoration, specifically how well high-flow releases maximize sheet flow while minimizing undesirable outcomes caused by past management that bypassed wetlands and conveyed polluted water through canals to sensitive downstream areas with highly valued ecological resources. In addition to serving Everglades restoration, DPM science is likely to influence similar adaptive management programs throughout the nation’s network of federal and state-managed river corridors, floodplains, and riparian ecosystems.
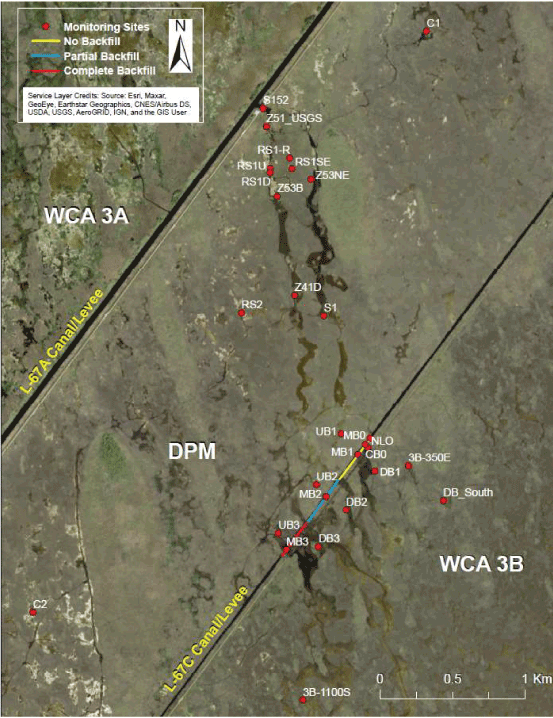
Map showing the Decompartmentalization Physical Model (DPM) experimental area near the L-67A and L-67C canal-levee system that impedes wetland sheet flow. High-flow releases through gated culverts at the S-152 structure moved southerly and easterly through the DPM wetland, eventually passing through the 914-meter gap in the L67-C levee with various levels of canal backfill (yellow, no backfill; blue, partial backfill; red, full backfill). Hydrologic, sedimentary, and biological response variables were measured at more than 20 wetland and canal sites between 2010 and 2022. km, kilometer; WCA, Water Conservation Area.
Purpose and Scope of this Report
Scientific research at DPM was conducted by multiple agencies and institutions. Here we focus on the work conducted by the U.S. Geological Survey (USGS) in measuring high-flow hydraulics; and interactions with vegetation and microtopography; as well as bed shear stress and sediment entrainment, phosphorous transport, and high-flow ecosystem effects measured as changes in aquatic metabolism. Also measured were hydrologic and water-quality effects of built structures, such as levee gaps with different levels of backfilling in adjacent canals. This report describes the USGS-led data collection and results, as well as measurement methods, instruments used, anticipated accuracy, and quality assurance approaches. The data are published in two data releases (Harvey and others, 2018, 2022a). The data collected for the entire twelve-year life of the project are available in the second of those data releases (Harvey and others, 2022a). Some data types that were collected early in the study and then were no longer collected by 2016 are available in Choi and others (2016). Model documentation and inputs and outputs are available in the present publication and three data releases (Harvey and others, 2022b; Harvey and Choi, 2022b; Choi and Harvey, 2023).
Overview of DPM Site Characteristics, Operation, and Data Collection
The DPM is an approximately 4-by-3-kilometer (km) area of degraded ridge and slough wetland within an area known as the “pocket” (fig. 1). It is referred to as such because it was enclosed by two levees, the L-67A levee that demarcates the upstream boundary with WCA 3A to the northwest and the L-67C levee that demarcates the downstream boundary with WCA 3B to the southeast. The DPM has been hydrologically isolated between those levees for approximately 60 years.
To examine high-flow impacts, new structures had to be built at the DPM. The infrastructure included ten gated culverts (S-152) emplaced in the L67-A levee. The culverts supply gravity drainage from WCA 3A into the DPM “pocket” area with a combined capacity up to 650 cubic feet per second (ft3/s) into the DPM wetlands with a desire to produce sheet flows in DPM wetlands with water velocities up to ten times greater than background velocities. At the southeastern edge of the “pocket,” a gap of 3,000 feet (ft), that is, 914-meter (m) wide, was created by removing a portion of the L-67C levee and backfilling the adjacent canal (L67-C) to test effects of complete, partial, and no backfilling of the canal, respectively (fig. 1).
The operational window of the S-152 culverts was subject to certain water level and phosphorus concentration triggers that initially limited operation to the months of November, December and January but later expanded to any time of year when targets were met to avoid high concentrations of phosphorus and excessive hydration of WCA 3B. On average the S-152 culverts were open and releasing water into the DPM on slightly more than a quarter of the time, accounting for 28 percent of the days between 2012 and 2022. The number of discrete openings of the culverts (excluding a few short-term openings for maintenance) was approximately sixteen, and openings lasted between 1 day and several months. The maximum combined discharge of the culverts was 637 ft3/s, and the average combined discharge was 216 ft3/s plus or minus a standard deviation of 109 ft3/s. The S-152 culvert operation schedule is summarized in table 1.
Table 1.
Starting and ending dates for the S-152 culvert high-flow releases into the Decompartmentalization Physical Model experimental wetland.[Dates shown as month/day/year. Times shown as HH:MM in Eastern daylight time or Eastern standard time depending on date. —, no data; NA, not applicable]
Flow releases were intended to spread radially into DPM wetlands and flow southeasterly, eventually passing through a 914-meter (m) gap in the downstream levee (L-67C) that would convey flow into WCA 3B (fig. 1). Three styles of backfilling were employed in the L-67C canal just upstream of the levee gap. The northeast section of the canal gap, comprising one-third of the 914-m gap, was not backfilled, whereas the center part of the canal gap was partially backfilled, and the southwest section was completely backfilled (fig. 1). The purpose was to test how backfilling of canals just upstream of levee gaps affects the source of water passing through the gap (for example, whether it is primarily from wetland sheet flow or primarily from flow in the canal toward the gap, as well as the transport of suspended sediment and phosphorus to wetlands downstream of the gap).
Data Collection Network
The DPM network of platforms and recording instruments was designed to test the hydraulic condition of restored sheet flow (e.g., laminar versus turbulent flow) and how it propagates through the wetlands and interacts with vegetation and bed sediment, how much suspended sediment is entrained and how far does it travel, and how is downstream phosphorus transport affected. We also investigated ecosystem processes by comparing low- and high-flow metabolism signatures that reveal changes in productivity and ecosystem respiration.
Measurements were made along a gradient of increased sheet flow velocities as they decreased in a radial pattern with distance away from the S-152 structure. Field monitoring of hydrologic and biological parameters at DPM were conducted under low-flow (baseline) and high-flow conditions at “impact” sites in the affected flow way. Those measurements were compared with data collected at “control” sites that were too far away to be affected by flow releases. Two control sites (C1 and C2) were established that were thought to be located outside of the influence of sheet flow enhancement, although site C1 proved to be somewhat affected by high flow.
“Sentinel” platform sites were established to accommodate infrastructure and instruments to continuously measure hydrologic and ecological responses, and to permit access to measure detailed hydraulic sedimentary, biogeochemical, and ecological responses to high flow while minimizing disturbance caused by frequent site access. Measurements were collected both by continuous self-operating instruments emplaced on the platforms for the wet season or by scientists who made discrete measurements during site visits.
In our early measurements, we observed that the most rapid responses and highest impact area were generally within 700 m of S-152. Farther downstream, the radial spreading of the flow had decreased the effects on water level and velocity. Those observations informed additional site selections. Eventually more than 20 fixed stations with sampling platforms were routinely visited throughout the wet season to make discrete measurements and to install, calibrate, and download data from continuously measuring instruments. Detailed naming and location information for the sites is given in table 2.
At each platform, we measured changes in water velocity, water depth, solute and suspended concentrations, sediment grain size and organic content, vegetation community type and stem density and diameter (which influences flow and particle dynamics), and microtopographic differences between slough and ridge. In addition, various environmental and introduced tracers were measured during the flow releases to capture local and larger scale surface water flow patterns. The detailed methods for all those measurements are provided in the “Methods” section of this report.
The complete hydrologic network of measurement sites extended from the S152-Head site in the L-67A canal on the upstream side of the DPM through the many sites in the pocket (S152-Tail, C1, C2, Z51-USGS, RS1U, RS1D, RS1-R, RS1SE, Z53B, Z53NE, Z41D, RS2, S1, UB1, UB2, and UB3), and through the gap in the L67-C levee into WCA 3B (fig. 1). In the L67-C canal adjacent to the levee gap were canal discharge measurement sites MB0, MB1, MB2, MB3, CB0, and S633-C (fig. 1.1. in appendix 1). The canal sites were paired with wetland sites upstream (UB1, UB2, and UB3) and downstream (DB1, DB2, DB3, 3B-350E, 3B-1100S, S633GNU-A, and S633GSU-B) of the L67-C levee gap to detect and sources of flow and amounts of flow passing through the levee gap into WCA 3B (fig. 1.1. in appendix 1).
Table 2.
Geographic coordinates of all sampling sites.[Some sites permitted sampling both a slough (S) and ridge (R), or both the upstream side (Head) and downstream side (Tail) of the S-152 structure, as reflected in detailed site name.]
Vegetation type was altered from ridge to slough after Active Marsh Improvement project initiation.
Site maintained by Everglades Depth Estimation Network (EDEN; 2020).
Locations and dates of autonomous measurements of water depths, water velocities, and water quality parameters are summarized in table 3. Data collection began in 2010 with collection of baseline low-flow data in the DPM before the construction of the culverts and levee gaps. Sampling occurred during the wet season (usually August through March). The 2010–11 wet season was very dry and ended with a severe fire in June 2011 that burned vegetation (but not peat) throughout the DPM experimental area. Measurements between 2010 and 2013 provided baseline low-flow data before the construction of the culverts and levee gaps that allowed high-flow to begin. The construction of levee gap and canal backfill treatments were completed in summer of 2012 and S-152 culverts were completed in late summer of 2013. The DPM high-flow experiment became operational on November 5, 2013, and has been operated for various periods each year through 2022. Although DPM experiments ended in 2022, the structures remain and will be operated as a part of Central Everglades Planning Project-South (CEPP-S). Recently, as a part of CEPP-S, a new levee gap (S-6333) was created in the L-67C levee in the path of the future S-6333 culvert structure that will be emplaced in the L-67A structure with measurements of flow being made at sites S633GNU-A and S633GSU-B (fig. 1.1. in appendix 1).
Table 3.
Summary of autonomous instrument deployments to measure water flow and water quality in the DPM.[Instrument type is designated by a code: water depth was measured using kilopound per square inch (KPSI) pressure transducers; water velocity was measured with acoustic doppler velocimeters (ADV); water quality was measured using sondes (QW); and vertical temperature profiles were measured (HOBO) in water column or peat. Specific instrument details and parameters measured are provided in appropriate sections of the text. Dates shown as month/day/year. NA, not applicable]
Active Marsh Improvement
Much of the hydraulic and sedimentary measurements were focused on detecting how sloughs responded to high flows. In fact, there were abundant indicators of flow-induced clearing of periphyton-clogged sloughs observed at DPM. However, there were no indications that emergent macrophytes that were in the process of invading sloughs had been affected, which could limit the long-term effectiveness of high-flow restoration of sloughs. In 2016, the South Florida Water Management District (SFWMD) began testing a program called Active Marsh Improvement (AMI) that involved application of herbicide in two primary historical sloughs along the southerly DPM flow path. Location of the AMI project is shown in figure 1.2. (appendix 1). Herbicide was applied on various days between September 26 and November 30 in 2016. During the winter of 2016–17, several trips were made by airboat to push over the standing dead vegetation in the herbicide impacted areas. Active efforts to eliminate emergent macrophytes in the selected historical sloughs were completed in March 2017. A May 2017 aerial image from Google Earth showed that sawgrass stems were dead and were pushed over but stems were still largely intact. In November 2017, the sawgrass stems were below the water surface with evidence of decay but also had dead stem bases projecting into the water column. In subsequent years, the AMI treatment area appeared slough-like.
Field and Laboratory Methods
DPM Experimental Flow Operations and Measurement Details
Background measurements of flow and sediment and phosphorus dynamics began in 2010. Construction of DPM structural features on the levees was initiated in June 2012 and completed in October 2013. Fifteen high-flow releases have been conducted (table 1) with measured discharges through the S-152 culverts listed in tables 2.1 through 2.8 in appendix 2. The combined discharge capacity of approximately 250 ft3/s through the S-152 structure generated water velocities as high as 6 centimeters per second (cm/s) at 450 m. The L-67C levee was degraded over a period of months and then breached on December 12, 2012, allowing water to flow from the pocket into WCA 3B unimpeded at moderate to high water levels.
Prior to the first of the S-152 flow release, the USGS installed instrumentation to compute continuous records of the headwater stage on the upstream side and the tail-water stage on the downstream side of the structure which would serve to calibrate measured flows through S-152 (Mark Dickman, [USGS], oral commun., 2015). An Argonaut-SW (shallow water) acoustic Doppler velocity meter (ADVM) was deployed inside of the downstream end of culvert pipe number 2, as counted from the north end of the structure, and a second SW ADVM was installed inside of the downstream end of pipe number 6. To develop discharge ratings, ten discharge measurements were made on the downstream end of each culvert using a SonTek FlowTracker according to the point velocity area method outlined in the SonTek FlowTracker Technical Manual (SonTek, 2007). The USGS also measured continuous water temperature and specific conductance on the headwater and tail water sides of S-152, EDEN 8, and 69 East, for the purpose of determining the source of water flowing through the S-152 structure.
Benchmark Elevation Surveying
Minor variations in topography are important drivers of sheet flow in the extremely flat terrain of the Everglades. Traditional ground-based surveying is impractical in the DPM research area because of the long distances involved, the challenging water-covered environment, and the soft-sediment conditions. A satellite-based survey was conducted to establish benchmark elevations at most of the DPM research sites to determine ground elevations and to relate the measured water levels across the project area to a common elevation datum.
Static elevation surveys were performed using Global Navigation Satellite Systems techniques following general guidance (Rydlund and Densmore, 2012) to achieve Level-1 precision, that is, the highest quality with the lowest achievable uncertainty, by requiring two occupations (observations on consecutive days at different times of the day) at least 4 hours long. Level-1 precision requires that the vertical peak-to-peak error should not exceed 0.06 m and the root mean squared error of the solution should not exceed 0.03 m as stated in Rydlund and Densmore (2012).
Elevation surveys using the North American Vertical Datum of 1988 (NAVD 88) were conducted five times between 2011 and 2021 to determine elevations of fixed benchmarks in NAVD 88 on platforms at DPM sites Z51_USGS-S, RS1D-S, RS2-S, S1-S, UB1-S, UB2-S, UB3-S, DB2-S, C1-S, C2-S, S633GNU-A-R, S633GSU-B-R, and at nearby National Geodetic Survey (NGS) benchmarks where elevation had previously been established (FCE_3888 in 2012, FCE_3889 in 2015, and AC4770 and AC4771 in 2021). Each survey consisted of two four-hour static deployments, one each day, with the first deployment in the morning of the first day and the second during the afternoon of the second day to collect data from a different set of satellites during each survey. Data files were submitted to NGS Online Positioning User Service program for processing. Uncertainties are provided with the elevation estimates in table 4. Those uncertainties were estimated using different methods that reflect the improving technology during the time span of the surveys. Our requirement for the DPM was for elevations with uncertainties less than 2 centimeters (cm). In all cases except one, the uncertainties were less than 1.5 cm. At one site, (Z51-USGS-S), the uncertainty was 4.4 cm, however, we accepted that result because of the good agreement between results on the two days of that survey and because the results otherwise met the requirements of a Level-1 survey.
Table 4.
Benchmark elevation survey data along with water depth measured on the survey day and derived ground elevation at each site.[Latitude and longitude in decimal degrees based on WGS 84 coordinate system. Date(s) of reference marker (RM) survey in the format of month/day/year. Elevation of the reference marker (RM) relative to North American Vertical Datum of 1988 (NAVD 88), in meters. Arithmetic mean of all peat elevation, in meters, relative to NAVD 88, from each visit, calculated as shown below. Standard deviation of all peat elevation, in meters, relative to NAVD 88, from each visit, calculated as shown below. Number of observations of all peat elevation collected at site visits.]
Vertical Peak to Peak Error: difference between maximum and minimum value of the coordinates obtained from the three baseline solutions (Rydlund and Densmore, 2012).
Root Mean Square Error: vertical accuracy at 95 percent confidence of the survey data using the high-accuracy independent dataset of trusted benchmarks (Rydlund and Densmore, 2012).
The static survey establishes the elevation of the platform reference marker, which were transferred as needed to estimate water levels and ground elevations by differential leveling techniques (Kenney, 2010). For example, we transferred the NAVD 88 elevation from the platform reference marker to the top of the kilopound per square inch (KPSI) standpipe, from which we made manual “tape-down” measurements to the water surface.
Upon arrival at a site the “down-to-water” (DTW) vertical distance was measured from the reference marker (RM) on the top of the KPSI standpipe down to the water surface, which when subtracted from the reference marker elevation determines the water-surface elevation for that day:
whereThe ground elevation of the peat surface was measured on each visit as:
where is the ground elevation as defined by the top of the peat surface determined on the day of the site visit. Water depth,and the standard deviation can be computed as:
where N denotes total number of visits.In the next section (“Surface-Water Elevation and Groundwater Hydraulic Head”) we discuss the use of discrete measurements of surface water level in combination with autonomously collected pressure transducer measurements to produce a time series of surface water elevations and water depths. In addition, the discrete water-surface elevation provided a basis to correct the KPSI pressure transducer measurements which often differed slightly because of drift and offset.
Surface Water Elevation and Groundwater Hydraulic Head
Surface water elevations were measured continuously using general guidance of the former USGS Office of Surface Water (Kenney, 2010). We used pressure transducers (KPSI 501 series) with an accuracy of ±0.3 cm to record water level at 15-minute intervals (TE Connectivity, 2017). The transducers were deployed by emplacement in screened 1.5-inch polyvinyl chloride (PVC) standpipes affixed with a through-bolt to a steel pipe previously driven into the bedrock. Pressure transducer sensors were suspended within standpipes at a pre-determined height where the hole on the side of each sensor was close to or below the ground surface such that surface water could always enter the hole, even at very low water levels.
The elevation of the KPSI pressure transducer sensor (ZKPSI) was determined by measuring the vertical distance between the surveyed reference marker and the KPSI sensor hole. The reference marker (RM) located at the top of the steel pipe was surveyed to determine absolute elevation (ZRM) by the GNSS technique described in detail in the “Benchmark Elevation Surveying” section. KPSI transducer-measured water levels, ZWL-KPSI, were then calculated by adding transducer hydrostatic pressure readings, HPKPSI, to the transducer reference elevation, ZKPSI:
Water depth was calculated by subtracting the mean ground elevation of peat (eq. 3) from a time series of the KPSI transducer-measured surface water elevations (eq. 5).Before finalizing estimates of the KPSI transducer-measured surface-water elevations, the results were checked and corrected for offset and drift based on discrete measurements of the reference surface water elevation, (ZWL_RM). That is, during each site visit, the surface-water elevations were collected by measuring from a reference mark on the top of the steel pipe supporting the PVC tube with the KPSI pressure transducer inside. The reference mark had been transferred from the surveyed reference marker (RM) that was on top of one of the pipes supporting the platform.
Data adjustments were made to account for offset and drift of transducers. On days that the site was visited, the transducer-measured water level was compared with ZWL_RM, that is, the hand-measured reference measurement of surface water elevation. Because a discrete measurement of reference surface-water elevation is precise and independent of pressure-transducer measurements, it can serve as a check on pressure-transducer measurements. Over time, the expected slope of ZWL-KPSI plotted versus ZWL-RM is expected to be 1 with an intercept of zero. A deviation in slope and intercept indicates an offset between transducer reading and the true surface-water elevation. No adjustment was made unless the difference of ZWL-KPSI and ZWL-RM was greater than 0.7 cm. If the difference was greater, an average of the offset measured during that visit and the offset measured during the previous visit was used to adjust transducer readings for the period between visits.
Pressure transducers were installed at sites C1-S, C2-S, DB2-S, Z51_USGS-S, RS1D-S, RS2-S, S1-S, UB1-S, UB2-S, UB3-S, and S633GNU-A-R. Additionally, three sites, Z51_USGS, RS1 and UB2, had groundwater transducers installed. The only difference between the surface-water and the groundwater transducers was that the PVC pipe for groundwater transducers was only screened near the bottom of the pipe approximately 1 m below the peat surface compared to surface-water transducers where the PVC pipe was fully screened. Transducer data were downloaded, and instrument diagnostics performed at approximately monthly intervals. Late in the study, telemetry systems using a SUTRON SatLink 3 Logger/Transmitter were set up at UB2 (July 2021) and S633GNU-A (October 2021). Telemetry provided remote access to monitor the status of the instrument and measurements. Data were accessed using AQUARIUS, a software platform developed by the Aquatic Informatics company (Aquatic Informatics, 2024), which the USGS uses for visualizing and processing downloaded hydrologic time-series data that later are made accessible in the USGS National Water Information System (NWIS; USGS, 2016). In the processing of water elevation data, a standard AQUARIUS threshold was implemented so that water-elevation values below 1.37 m and above 3.35 m were suppressed to filter out extremely erroneous data that can result from instrument connection failures. Water elevation data downloaded from AQUARIUS were further processed by accounting for offset and drift as described in the previous paragraph. Presently, the data available described above that were processed by AQUARIUS and are posted on NWIS are only internally accessible and would need to be updated with the drift-corrected data before being published.
Start and end dates of the monitoring periods of surface-water and groundwater transducers at the study sites are summarized in table 3. Multiple breaks in data collection are due to technical issues, such as instrument failure and other complications. Groundwater-surface water interactions were assessed by measuring the vertical hydraulic gradient (Harvey and others, 2004). Those measurements were done at Z51_USGS-S, RS1D-S, and UB2, occurring at times indicated in table 3. To calculate the vertical hydraulic gradient, the elevation of the groundwater surface within the piezometer (ZWL-RM,gw) was subtracted from the measured elevation of surface water (ZWL-RM,sw) and that difference was divided by the vertical distance (L) from the center of the screen of the piezometer emplaced in the peat to the peat surface (eq. 6).
As defined, the hydraulic gradient expresses groundwater recharge as a positive value and discharge as a negative value. At the time of site visits the groundwater hydraulic head was determined by measuring DTW inside the groundwater piezometer which was directly compared with the surface water elevation. Hydraulic gradients were also analyzed using transducer-measured water levels (ZWL-KPSI,sw and ZWL-KPSI,gw), with careful consideration given to correct transducer offset and drift to achieve the needed accuracy.Microtopography
Small-scale topography variations (referred to as microtopography) were measured at C1, C2, RS1D, RS2, S1, UB1, UB2, and UB3 starting in 2010; DB2 in 2011; RS1U in 2013; and Z51_USGS in 2015. The purpose of this study was to determine the surface elevations of the flocculent organic surface of the wetland and the underlying consolidated peat along ridge to slough transects. This was accomplished by repeatedly collecting measurements of the depth from the water surface to the surface of the floc and peat, and then relating the surface-water elevation to a locally surveyed ground elevation (which was described in the “Benchmark Elevation Surveying” section). The water depths to floc and peat, hfloc and hpeat, are measured by determining the vertical distance from the water surface to the floc and peat using a calibrated chlorinated polyvinyl chloride (CPVC) depth probe with an I-shaped foot, as described in Choi and Harvey (2013). The probe was gently placed on the floc to measure the depth to floc, and then pressure was applied to sink the probe through the floc in order to measure the depth to peat.
The elevations of the ground surfaces, with subscript floc indicating the flocculent organic-matter surface and peat indicating the consolidated peat surface, for example, Zfloc or Zpeat, respectively, were found by subtracting the measured water depth to the floc or peat (hfloc or hpeat) from the surface-water elevation recorded from KSPI pressure transducers (ZWL) at the site for a given day and time, for example:
At sites where surface water elevation was not available (that is, a KPSI pressure transducer was not installed), a surface-water level was applied from the next closest site if the site was within 30 m. At such a relatively short distance, water level differences are insignificant.
In 2010 and 2011, measurements were made at approximately 30 points, oriented in a large circle with a radius of roughly 100 m. At each of the 30 locations, 6 microtopography measurements were taken (6 floc-surface elevations and 6 corresponding peat-surface elevations). The position of the circle was chosen to include both ridge and slough topographies. Three measurements were collected within one square meter at each sampling point to calculate an average water depth to the floc and to the peat.
Additionally, from 2010 to 2019, water-depth-to-floc and water-depth-to-peat measurements were collected along ridge to slough transects at each site. Depending on length of the platform and coverage of ridge and slough, as many as 6 positions along the transect were selected for repeated depth measurements over time. At each transect position, triplicate measurements of depth to floc and depth to peat were made in a one square meter centered on that position. The triplicate measurements were averaged for water depth to floc and peat for each position on the transect.
During each field visit, independent of the transect measurements, one-point measurements of depth to floc and peat in the slough were recorded in an undisturbed area near each slough site. This ensured that microtopography measurements were made at all stations, including those without transect measurements, to help monitor changes in ground elevation over time. Calculations of water surface elevation, ground elevation, mean ground elevation, and standard deviation of ground elevations are provided in equations 1 through 4 (in section “Benchmark Elevation Surveying”).
Measuring Surface-Water Flow Velocity and Bed Shear Stress
Water flow velocity was estimated at fixed sites in wetlands with vegetation using continuously measuring SonTek down-looking acoustic Doppler velocimeters (Field ADV or Argonaut ADV) or with Nortek down-looking Vectrino ADVs. At additional sites away from measurement platforms, we used Sontek FlowTracker ADVs. We refer collectively to those instruments as ADVs. After March 2016, the Nortek instruments were no longer used, and by September 2019, the Sontek Field ADVs had been decommissioned or replaced by SonTek down-looking Argonaut ADVs. In the deeper and unobstructed surface waters of canals, we made measurements with acoustic Doppler current profilers (SonTek Argonaut XR or SonTek IQ) that estimated velocity profiles through the water column that are collectively referred to as ADCPs.
The measurement accuracy of ADVs and ADCPs was similar. Sontek Field ADVs and Sontek Argonaut ADVs are capable of measuring flow velocity to a resolution of 0.01 cm/s with an accuracy of 1 percent of the measured velocity, however the practical measurement resolution was more often 0.1 cm/s. The Nortek Vectrino ADV can measure velocity to a resolution of 0.1 cm/s with an accuracy of 0.5 percent (SonTek, 2015, 2021a, b; Nortek, 2020). The FlowTracker ADV can measure flow velocity to a resolution of 0.01 cm/s with an accuracy of 1 percent of measured velocity (SonTek, 2022). The ADCPs, Argonaut XR and IQ plus, both measure flow velocity to a resolution of 0.01 cm/s with an accuracy of 1 percent, respectively (SonTek, 2015, 2021a, b; Nortek, 2020).
Acoustic Doppler Velocimeter Measurements at Fixed Platforms
In the wetlands the ADV instruments were attached to custom cradles deployed on the upstream site of the fixed platforms at the location of the main monitoring sites referred to as sentinel sites (table 3) near where other hydrologic and biogeochemical measurements were being made (fig. 1). We followed the general operating procedures outlined in Harvey and others (2009) to measure flow velocity at a fixed depth located approximately at the midpoint of the water column. Flow velocity measurements were made continuously for months at a time in which velocities were recorded for one-minute bursts (600 samples) and collected every 15 minutes to save battery power. Quality control on velocity datasets were followed to maintain standards suggested by the instrument manufacturer (SonTek, 2001), as well as specific criteria developed and refined in a prior Everglades study (Riscassi and Schaffranek, 2004). Beam checks were performed on each visit as recommended by the manufacturer. To assess beam check results, the noise count was evaluated. If the noise level of any one beam is outside the range of 60–70 counts, this can be an indicator of an interference with the ADV operation problem by a noisy environment or a larger problem with the electronics. For this case, the system was moved away from any motors or electronics to see if the noise level changed. If no improvement was achieved, the instrument was sent for repair.
To further ensure data quality, ADV velocity data had to be filtered so that samples within a burst passed a 40-percent-minimum correlation filter of signal strength between the three receivers. Within a burst, at least 70 percent of samples need to pass the correlation filter to be retained (Martin and others, 2002). Data were also filtered using an acoustic signal-to-noise ratio (SNR) threshold to eliminate data with SNR values below 5 decibels (dB) and 3.5 dB for ADV and Argonaut ADV, respectively. A sound-speed correction was applied to ADV data using either data from an attached convention temperature device (CTD) or average temperature and salinity recorded by a nearby CTD during the deployment period. The Nortek Vectrino also applied a sound-speed correction using an embedded thermistor and measured salinity.
After filtering, a phase-space threshold “despiking” (that is, removing unrealistic data) and smoothing algorithm (Goring and Nikora, 2002) was applied to ADV, Argonaut ADV, and Vectrino data. Unrealistic data are identified by values that exceed 4 times the standard deviation of a 2-day window from the running median and then removed. Data points identified for smoothing are determined by values that exceed 3 times the standard deviation of a 4-hour window from the running median and then replaced with the running median. These quality-assured data were time averaged to produce hourly and daily values and statistics such as the standard deviation. Flow direction was determined using the Yamartino method (Yamartino, 1984).
We visited the monitoring sites for regular (quarterly) maintenance of the instruments during continuous deployments, during which data were downloaded, batteries were replaced, the compass was calibrated, and diagnostic beam checks were performed on the instrument. On each site visit, the height of probes was adjusted to keep the sampling volume approximately at or slightly below the mid-point of the water column anticipated for the deployment period. Instruments were continuously deployed on average for six months during the study period, between September and March, and we retrieved the instruments from the sites to avoid any potential damage owing to the probe’s exposure to air during the low water-level season. The velocity data collected during times when we visited the monitoring sites were removed to exclude the data from the disturbed system.
Late in the study (July 2021), telemetry systems using a SUTRON SatLink 3 Logger/Transmitter were set up at UB2, DB1, 3B-350E, 3B-1100S, S633GNU-A, and S633GSU-B). which the USGS uses for visualizing and processing downloaded hydrologic time-series data that later are made accessible in the NWIS (USGS, 2016). In the processing of ADV data that was accessed from telemetry using AQUARIUS (Aquatic Informatics, 2024), a threshold was implemented so that flow-speed values below −5 ft/s and above 5 ft/s were suppressed to filter out extremely erroneous data that can result from instrument connection failures. Unfortunately, due to the Covid-19 pandemic, field operations during the last year and a half of the study were negatively affected and the instrument data were never manually downloaded. As a result, flow speed data that were downloaded remotely and processed through AQUARIUS did not retain SNR values and additional quality control information, so processing of data from the last year of the study excluded the correlation and SNR filtering that had been performed on the data collected earlier. Ideally, in the future, ADV data should also be manually downloaded manually, and quality assurance and quality control (QA/QC) should be performed manually using the filtering and despiking steps described earlier in this section to satisfactorily assure the data. Downloading ADV data by SatLink will only be useful as a method for remotely screening data to identify potential problems, until a time when all quality control information can be remotely downloaded with the speed data.
Several methods were used for estimating the flow speed at RS1D-S after the USGS ADV was removed late in the study. For high flows after June 19, 2019, the ADV data from a SFWMD emplacement were used. For high-flow periods with no data, the velocities at RS1D-S were estimated using a linear regression between ADV data and S152 discharge (speed [in cm/s] = 0.0147*(S152 discharge [in ft3/s]) + 0.7893). After June 19, 2019, and when the S-152 culverts were closed, we estimated the resulting low-flow velocities as equal to the average of the measured low-flow velocities between 2010 and 2019 (0.5 cm/s).
Early in the study (2011–15), velocity profiles were measured vertically in the water column at selected sites. For vertical profiling, the instrument cradles were further modified for vertical movement that permitted measurements at multiple depths in the water column. At each depth increment, velocities were measured in 1-minute bursts yielding 600 samples with SNRs monitored continuously to determine if the sample volume was obstructed by vegetation and as an indicator of the vertical location of the top of the floc. Velocity profile data underwent the same corrections and filters as the continuous velocity data. Velocity profiles can be used to refine depth-averaged estimates based on continuously measured velocities measured at approximately the mid-depth. Essentially, point velocities at the mid-depth can be adjusted using velocity profile shape factors described in the procedures documented in Lightbody and Nepf (2006) and in Harvey and others (2009).
FlowTracker Measurements Away from Fixed Platforms
Discrete measurements of flow velocity were made using a SonTek ADV FlowTracker. Those measurements permitted data collection at sites where installation of fixed platforms with ADV cradles was impractical. FlowTracker data collection in the wetland was performed away from any artificial obstructions, such as the airboat. The FlowTracker was positioned in a location with approximately 30 cm of clear space around the probes; vegetation was clipped if necessary. Data were recorded for one minute at the midpoint of water column. Sometimes data were collected at 3 different depths: 5 cm above floc surface, in the middle of the water column, and 5 cm below water surface. Instrument operating procedure performed was followed according to the FlowTracker Handheld ADV Technical Manual (SonTek, 2007). Quality control of the measured data is continuously performed on all variables collected during a measurement. The SNR is the most important quality control data provided by the FlowTracker2, the default threshold of 10 dB is provided in FlowTracker2, User's Manual 1.6 (SonTek, 2019).
Bed Shear Stress Measurements
Bed shear stress measurements were taken pre-release and during the flow release at 6 discrete points along the RS1U ridge-to-slough transect using a Nortek Vectrino II Profiler ADV, manufactured by Nortek. To compute bed shear stress, the Vectrino Profiler was mounted on a vertically sliding rod and deployed to sample flow immediately above the bed. Each instrument reading consisted of instantaneous velocity collected over 18 points within a 2.5-cm vertical profile, at a spacing of 2.0 mm. The instrument was operated at high power to achieve the maximum SNR, with pinging set to “max interval.” Each reading was acquired over a period of 5 minutes. At the end of 5 minutes, the profiler was moved to its next location along the sliding rod (which overlapped with the first by about 0.5 cm) and again deployed. In this manner, four stacked 2.5-cm profiles were obtained near the bed. A fifth 2.5-cm profile was acquired in the middle of the water column at each site.
Raw velocity records were filtered to remove data points that did not meet standards for SNR (at least 5 dB), correlation (at least 40 percent), or consistency in redundant measurements of the z-direction velocity component. Instrument noise spikes were removed using Goring and Nikora’s (2002) threshold despiking algorithm. Following Biron and others (2004), profiles of total stress were calculated as the sum of the total kinetic energy derived stress and laminar stress, as follows:
where is a proportionality constant (0.19), and u’, v’, and w’ are the velocity fluctuations of the streamwise vertical and lateral components where < > denotes a temporal average, μ is dynamic viscosity (in pascal seconds, [Pa·s]), and U is the flow speed (in cm/s) at a height of z above the bed (in cm), where U is given as the resultant of the component-wise velocities,Canal Flow Velocity and Discharge
ADCPs were deployed at canal monitoring sites (table 3) to provide continuous records of flow velocity and discharge through the L-67C canal. The Argonaut XR and IQ plus ADCPs were deployed in an up-looking configuration and collected flow data in one-minute bursts every 30 minutes interval. The Argonaut XR provides a velocity profile as well as depth-averaged flow velocities in canals. The IQ plus measures velocity profiles and calculates the discharge through canal using velocity profiles and provided canal geometry information. For Argonaut XR quality control, the mean signal strength with each sample should be at least five counts above the noise level. The signal strength is reported in internal logarithmic units called counts (1 count = 0.43 dB). For IQ plus quality control, data with acoustic SNR of 3dB or less, as well as pitch or roll greater than 5 degrees, were discarded (SonTek, 2017).
Water discharge (flux) was measured at 4 cross sections in L-67C canal. The corresponding sites from northeast to southwest are MB0, CB0, MB2, MB3, and S633-C. SonTek IQ plus instruments were used to measure water flow speed with 4 beams oriented at different angles and estimated the mean flow speed by a power-law velocity profile model. We installed the IQ plus at MB2 and MB3 on February 6, 2019, at MB0 and CB0 on August 11, 2020, and at S633-C on April 14, 2021. Canal discharge measurements occurred at the time periods shown in table 3. The instruments were installed near the canal center and close to the bottom, facing upstream, as instructed by the instrument manufacturer (SonTek, 2017). Data were collected every 15 minutes at MB2 and MB3 and every 30 minutes at MB0, CB0, and S633-C.
Canal water flux (flowing parallel to the canal) was estimated by multiplying the mean flow speed by the canal cross-section area determined from user measurements of canal geometry and water depth measured by the onboard pressure sensor. An accurate channel geometry was determined by detailed surveying at MB2 and MB3 performed manually with transect tapes and meter stick. Because of the much deeper water depth, we used the ADCP to obtain the depths at specified cross-section locations to determine channel geometry at CB0, MB0, and S633-C. Data collected at site MB0 was found to have instrument difficulties, showing questionable values, so data were also collected with an IQ deployed near that site. A velocity mapping effort on September 1, 2020, also provided additional information for sites MB0 and CB0 (Knight and Soderqvist, 2021). To ensure data quality, data with SNR lower than 3 dB or pitch or roll larger than 5 degrees were discarded. Data were cleaned with a despiking algorithm, in which extreme spikes are removed before smaller spikes are replaced by a moving median. The resulting quality-assured data are used to produce daily values.
Suspended Sediment Sampling
During the first three DPM flow releases in 2013, 2014, and 2015, samples were collected for analysis of suspended sediment concentration (SSC), SSC load, and suspended particle size. Water column samples were collected by peristaltic pumping at a rate of 60 milliliters per minute (mL/min) through size 16 Masterflex tubing with a 500-micrometer (μm) Nitex screen fitted to a tubing inlet into acid-washed 1-liter (L) Nalgene sample bottles. Two 1-L samples were collected for a laboratory-based measurement of SSC and stored in the shade at near ambient temperature in a cooler with ice. Additional 250-mL samples were collected for the immediate analysis of particle size using the Laser In Situ Scattering and Transmissometry (LISST)-Portable-XR benchtop laser diffraction particle size analyzer (Sequoia Scientific, Inc., 2019). The LISST-Portable optically analyzes suspended sediment to provide a volumetrically based estimate of the particle size distribution in the water sample. Particle detections in a small sample aliquot, approximately 50 milliliters (mL), are divided into 44 logarithmically spaced size-class bins and reported across a range of 0.34–500 μm (Sequoia Scientific, Inc., 2019). Resulting measurements have a resolution of less than 1 mg/L and an accuracy of ±20 percent of measured sediment concentration (Sequoia Scientific, Inc., 2019). This instrument provides best results for samples with particle concentrations ranging between 30 and 1,900 milligrams per liter (mg/L), depending on the particle size distribution (Sequoia Scientific, Inc., 2019).
In November 2013, the water column samples were collected prior to the high flow release that began at 9:30 a.m. eastern standard time (EST) on November 5, 2013, during the high flow breakthrough on November 5 and 6, 2013, and during the steady high flow that followed (November 7, 8, and 10, 2013).
In November 2014, the water column samples were collected prior to the high flow release that began at 9:35 a.m. on November 4, 2014, during the flow breakthrough on November 4 and on November 5, 2014, and during the long period of steady high flow that followed, with sampling occurring on November 7, 2014, December 4, 2015, and January 21, 2015. After the closing of the S-152 on January 29, 2015, sampling was conducted on March 9 and 10, 2015.
In November 2016, the water column samplers were collected prior to the high-flow release that began at 9:30 a.m. on November 16, 2016, during the breakthrough of the first flow pulse (sampling between 9:30 a.m. on November 16, 2015, and 11:08 a.m. on November 17, 2015). The S-152 was closed briefly from 3:20 p.m. on November 17, 2015, to 9:00 a.m. on November 19, 2015, with sampling occurring at 8:57 a.m. on November 19. The S-152 was reopened for a second pulse at 9:00 a.m. on November 19, 2015, and sampling began at 9:41 a.m. on November 19, 2015, and on occasional days through 11:25 a.m. on January 21, 2016. The S-152 was closed on January 28, 2016, after which additional sampling occurred on March 1 and 2, 2016. The timeline and details of suspended sediment concentration sampling are shown in table 5.
Table 5.
Flow event, S-152 flow condition, sampling dates, and sampling sites for suspended sediment concentration (SSC) and particle size sampling.[Dates shown as month/day/year. Times shown as HH:MM in eastern daylight time or eastern standard time depending on date.]
Some of the suspended sediment sampling sites had paired sampling in nearby ridges and sloughs, and those sites have names beginning with “RS”. At other sites samples were collected from only sloughs. Samples at site RS1U were collected at 2-m-spaced intervals across the transition between ridge and slough (fig. 2). At the transect endpoints in the ridge and slough the samples were collected to represent the upper third, middle, and near-bed water (5 cm above floc surface) water column. Only near-bed samples were collected at locations between the transect endpoints at site RS1U. In 2014, sites RS1D, Z53B, RS1SE and RS2 were sampled from the low and middle water column in the ridge and slough and samples were omitted from the upper water column. At slough sites Z51_USGS, S1, UB1, UB2, UB3, DB1, DB2 and DB3, samples were collected from only the low and middle water column.
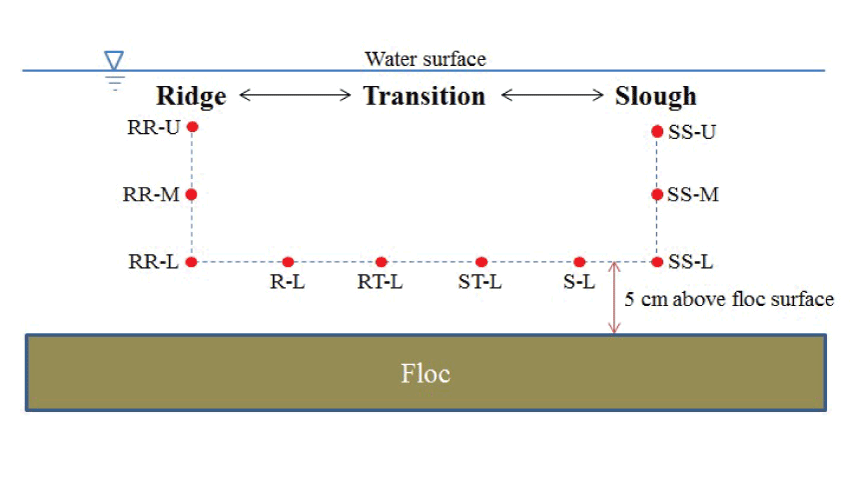
Diagram showing suspended sediment concentration sampling scheme along the RS1U transect. cm, centimeter.
In the laboratory, the volume of each suspended sediment sample was measured prior to filtration with a graduated cylinder. Each sample was then processed by vacuum filtration through a 0.7-μm glass fiber filter (Whatman GF/F) using the methods of Noe and others (2010). The filters were prepared by vacuum filtering 100 mL of deionized water through the filter and then heating at 500 degrees Celsius (°C) for one hour to remove any present organic material. The initial dry mass of the filter was measured on an analytical balance. The sediment-laden filters were oven dried at 60 °C and then weighed with an analytical balance. With this information, the SSC was calculated for each sampling time at each site by subtracting the mass of the dried filter from the mass of the sediment-laden filter and dividing the difference by the volume of the sample.
Duplicate SSC samples were collected on November 5, 2013, at two locations (sites RS1U_RR-M and RS1U_SS-M) and on November 8, 2013, at two locations (sites S1_L and UB1_L). They were also collected at sites UB2 and DB2 on March 9, 2015, and RS1U_RR-M and RS1U_SS-M on March 10, 2015. Duplicate samples were analyzed to determine the amount of error present within sampling and processing methods.
Depth-averaged values of SSC concentration were calculated at locations where vertical sampling was conducted in the water column. The total water depth was divided into sample water column intervals (WCIi), where subscript i is a counter for each vertical depth interval that represents a sample in the water column. The WCIi is determined for a given sample by adding half of the distance to the adjacent sample that is above and half of the distance to the adjacent sample that is below. For samples nearest the water surface or the bed, the whole distance is used instead of half the distance to the floc surface or the water surface. The depth-averaged concentration is calculated by
whereDepth-averaged SSC concentrations were analyzed spatially to determine distribution variation across the study area. Distances were calculated as a nominal distance along the predicted flow path. To simplify the analysis, the primary flow path direction was chosen as a linear path from the S-152 culverts to site MB2, another linear path segment from site MB2 through site DB2. Site locations were perpendicularly projected to the primary flow path to calculate the nominal distance from the S-152 culverts.
The flux of SSC per unit cross-sectional area of wetland was determined using velocity data collected by either a SonTek ADV or Nortek Vectrino at each sampling location and water depth where SSC was sampled. If multiple flow velocities had been measured within a water column interval, the depth-averaged velocity within that interval was applied. Load, mass flux per unit width, is calculated by:
where is the concentration (in milligrams per cubic centimeter [mg/cm3]),Particle Size Measurements
Particle-size measurements were made in-situ and on samples collected from the water column, as well as on samples of sediment attached to floating and emergent vegetation and sediment samples from the bed. For example, water-column samples were analyzed for particle size whenever a duplicate sample was collected for an SSC analysis. Particle sizes also were measured on aliquots from epiphyton and bed-floc sediment samples. Also, whenever possible, particle size was measured on water-column samples where a duplicate sample had been collected for the analysis of total phosphorus concentration.
Most of the particle size analyses were performed using a laser in situ scattering and transmissometry (LISST)-Portable benchtop laser diffraction particle size analyzer (Sequoia Scientific, Inc.). The instrument optically analyzes suspended sediment as described in the previous section in a small sample aliquot, approximately 50 milliliters (mL) to provide a volumetrically based estimate of the particle size distribution in the sample across a range of 0.34–500 μm. The instrument divides particle detections into 44 logarithmically spaced size-class bins (Sequoia Scientific, Inc., 2019). This instrument provides best results for samples with particle concentrations ranging between 30 and 1,900 milligrams per liter (mg/L), depending on the particle size distribution, with a resolution of less than 1 mg/L and an accuracy of ±20 percent of measured sediment concentration (Sequoia Scientific, Inc., 2019). Three to five sample runs were averaged to determine the average particle size distribution. The analyzer also computed metrics such as median volume weighted, D50 which is the grain diameter at which 50 percent of the sediment sample is finer. Sediment sizes D10 and D60 were also computed to quantify the 10 percent and 60 percent finer fractions, respectively. A particle size uniformity coefficient, D60/D10, was calculated with larger values of D60/D10 indicating a more poorly sorted suspended sediment sample. Depth-averaged values for D50 and D60/D10 were calculated using the same procedure to calculate depth-averaged SSC values. LISST-Portable analyses were performed the same day of sample collection, either on the airboat deck or that evening off site.
Continuous records for a day or more of suspended particle size distributions were obtained by measuring in situ using a laser-diffraction particle size analyzer (LISST-100X or LISST-FLOC, Sequoia Scientific) using methods described in Noe and others (2010) and Harvey and others (2011). LISST-100X and LISST-FLOC instruments were used from November 2010 to November 2015 to make in-situ measurements of suspended particle size distribution with the results provided in an earlier data release for this project (Choi and others, 2016). The LISST instruments work by emitting light and measuring laser diffraction to estimate the in-situ volume concentration and size distribution of particulates in suspension. The particle-size measurement range is 1.25–250 μm and 7.5–1,500 μm for the LISST-100X and LISST-FLOC, respectively, and the ranges are analyzed by dividing into 32 logarithmically spaced size-class bins (Sequoia Scientific, Inc., 2009a, b). The resolution of LISST-100X and LISST-FLOC is less than 1 mg/L (Sequoia Scientific, Inc., 2022). The instruments are deployed horizontally at the mid-point of the water column and programmed to collect data in 60-second bursts every 15 minutes. The instruments can be subject to biofouling, which limits deployment to periods of up to three days. It should be noted that the LISST-FLOC is an extended version of the LISST-100X with general operating procedures that are almost identical. Production of the LISST-FLOC instrument has been discontinued.
All of the LISST particle size analyzers provide volume weighted particle size information. To convert from volume-weighted to mass-weighted values, a particle density distribution was applied to the LISST output based on a settling column experiment using Stoke’s Law to compute particle density as it varies as a function of particle size (Larsen and others, 2009a; Harvey and others, 2011). Volume-weighted size distributions were converted into mass-weighted mean size for each sample based on:
where mED is the mass-weighted mean size of particles (in micrometers [µm]), N is the number of bins in the size distribution, s is the particle size (in µm) of each bin center, V is the volume concentration (in microliters per liter [µL/L]) of the particles in each bin, ρ is the density (in micrograms per microliters [µg/µL]) of the particles in each bin, which is determined by the settling column experiment, described in Larsen and others (2009a), and M is the total mass concentration (in micrograms per liter [µg/L]) of the distribution.Biogeochemical sampling and analysis
Biogeochemical sampling was performed between November 8, 2010, and March 28, 2022, to characterize the nutrient content in waters and in fine particles on plant stems and in the bed sediment. Physical characteristics, such as particle grain size, also were determined. Sediment particles attached to emergent macrophyte stems and floating periphyton were collectively referred to as “epiphyton,” and were sampled with and compared to results from sediment particles comprising the flocculent sediment on the bed. Briefly, we measured the total and particulate phosphorus concentration in the water column and the total phosphorus, nitrogen, and organic content of epiphyton and bed-floc sediments.
Water-Column Sampling
Water column samples were collected for analysis of total phosphorus concentration (TP) in surface water. During 2013, 2014, and 2015, the water column samples were collected concurrently with SSC samples (see “Suspended Sediment Sampling” section) by peristaltic pumping from the middle of the water column at a rate of 60 mL/min through size 16 Masterflex tubing into a 1-liter acid washed Nalgene sample bottle. To avoid inadvertently capturing periphyton in the sample, the pump tubing had been fitted with a 500-μm “coarse” Nitex screen over the tubing inlet. Samples were preserved by adding sulfuric acid (H2SO4) to the sample bottle to reach a pH of 2 and placed on ice before shipment to the SFWMD laboratory for analysis. Samples were acidified with H2SO4 to reach a pH of 2 and packed on fresh ice and shipped overnight for analysis at the Wetland Biological Laboratory (WBL), University of Florida, Gainesville, Florida.
Epiphyton and Bed Floc Particulate Sampling
Epiphyton samples were collected that represented fine particulates principally of an organic nature attached to macrophyte stems as well as to leaves of floating aquatic plants (also described as periphyton mats). Epiphyton samples were collected using a wet/dry vacuum operated at approximately the water column’s mid-depth within the canopy of vegetation stems and floating vegetation, as described in Larsen and others (2009a). Epiphyton was dislodged from vegetation by moving the vacuum hose though the canopy and pumping a slurry of epiphyton into a plastic tub. If needed to collect a representative sample the macrophyte stems and floating vegetation were cut and collected in the plastic tub and shaken with some Everglades water to dislodge epiphyton and to create a slurry. The tub was tipped to pour the slurry through a 500-μm Nitex “coarse” filter into a 1-liter Nalgene acid-washed bottle that was allowed to settle and decanted until the sample fit within a 250-mL acid-washed Nalgene bottle.
Bed floc samples were collected by coring the bed with a clear sharpened acrylic tube (1.9-inch inside diameter) to obtain all the top layer of loose, flocculent organic sediment known as “floc” that lies atop more consolidated peat. Floc is important because it represents recently settled material that is biogeochemically active and that often is phosphorus rich. After the core tube was removed it was held upright, allowing time for the floc to settle back onto the more consolidated peat that was also captured in the core tube. Then the clear liquid was gently poured off the top of the core, retaining the slurry of floc above the well consolidated peat. The thickness of floc was recorded by measuring its height with a tape held outside the core tube. Next the slurry of floc was poured off through a 500-μm Nitex “coarse” filter into a 1-liter acid-washed sample bottle that was allowed to settle and decanted until the sample fit within a 250-mL acid-washed Nalgene bottle. Note that after September 27, 2019, bed floc samples were collected by peristaltic pumping of the floc layer without disturbing the more consolidated peat layer through a 500-μm Nitex “coarse” filter into collection bottles. The area of peristaltic pumping (approximately 10×10 cm) was kept to a minimum to collect floc from above the underlying peat in that area without also collecting the peat. The area of collection was expanded as necessary to collect enough sample for analysis. Samples were stored in coolers containing wet ice to maintain a sample temperature of 4 °C. Samples were shipped either the same day or the next day with fresh ice using one-day service to the WBL.
Water-Column Biogeochemical Analysis
The SFWMD analyzed TP concentrations of water samples by first undertaking an acid-persulfate digestion to convert organic and inorganic phosphates into reactive phosphate. Total phosphorus analysis is based on the formation of a blue-colored antimony-phosphomolybdate complex from the reaction of ammonium molybdate, and antimony potassium tartrate with reactive phosphate in an acidic medium followed by reduction with ascorbic acid. The blue color complex was measured by photometric analysis at 880 nanometers (nm) on the Lachat Flow Injection Analyzer (FIA) with a detection limit of 0.002 mg/L and detection range of 0.002 to 0.4 mg/L. The total phosphorus concentration includes phosphorus associated with suspended sediment and dissolved phosphorus in the water column.
Beginning in September 2016, water column samples were analyzed for both TP and total dissolved phosphorus (TDP) by the WBL. Samples were first subjected to an acid-persulfate digestion to hydrolyze all condensed inorganic and oxidize organic phosphates and convert them into orthophosphate. The digestate was then analyzed on a Bran+Luebbe AutoAnalyzer 3 (AA3) instrument following the guidance of standard method (SM) 4500P-F for automated ascorbic acid reduction method for TP or TDP analysis (Lipps and others, 2023). This method is applicable to surface waters, ground waters, and porewater for either TP or TDP. The applicable range for low level TP analysis using AA3 is from 0.002 to 0.15 mg/L, or higher can be measured by using sample dilution. Detection limit for water TP is 0.002 mg TP/L using a standard curve that ranged from 0–0.15 mg/L. For analyses that passed the QA and QC, the particulate phosphorus concentration (TPP) was determined as follows:
Epiphyton and Bed Floc Biogeochemical Analysis
All biogeochemical analyses of epiphyton and bed floc sediments were performed by the WBL. Analysis included: dry weight of solids, loss on ignition (LOI), total nitrogen (TN), total carbon (TC), and labile, microbial, and refractory (total remaining) phosphorus content of epiphyton and floc samples determined by several extractions and a total digestion. For dry weight of solids, samples were dried to a constant weight (detection limit of 0.01 percent), and for LOI, samples were ignited at 550±50 °C in a muffle furnace. Weights were determined using scales and balances verified daily using National Institute of Standards and Technology (NIST) certified weights. TN was found using a Thermo Electron FlashEA 1112. Epiphyton and floc samples underwent a sequence of NaHCO3 extraction, NaHCO3 plus chloroform extractions, and finally a perchloric acid digestion using a Technicon Autoanalyzer AA3.
The results from NaHCO3 and NaHCO3 plus chloroform extraction were used to determine organic phosphorus fractions consisting of refractory, microbial and labile phosphorus (Ivanoff and others, 1998) as follows:
where L(Laboratory Analytical Results Assessment
Laboratory results from the WBL were delivered in batches corresponding to sample trips. Each batch of analytical results had been flagged to indicate if reported concentrations were less than the minimum detectable level (MDL), that is, the concentration representing 99 percent confidence that the analyte was detected at a value is greater than zero, and a practical quantitation limit (PQL), that is, the estimated lowest concentration which can be reliably determined within the highest confidence limits of precision and accuracy. The MDL and PQL varied across different sampling runs, and their range is reported in table 6.
Table 6.
Principal quality assurance and quality control indicators for flagging of laboratory results.[Measurements were of total dissolved phosphorus of the water column and total phosphorus of soil floc or epiphyton on plants. QA/QC, quality assurance and quality control; µg/L, microgram per liter; mg/kg, milligram per kilogram; MDL, minimum detectable level; PQL, practical quantitation limit]
Laboratory QA and QC included determination of the accuracy of digestion process and calibration curves using commonly used standards such as WBL, NIST and ERA-Waters Corporation (ERA) certified reference materials for complex nutrients including NIST Peach Leave, WBL Standard Soil, ERA Simple Nutrients for total phosphorus of floc samples. When calibrations were inadequate the laboratory reanalyzed the sample. If the same response was obtained, the laboratory prepared new primary and calibration standards. If that failed, the laboratory checked and re-prepared stock solutions, and instrument service as a final resort. All outcomes were noted in a QC result log.
Further laboratory considerations for flagging included sample holding time, actual sample temperature, and minimum amount of sample for reliable data. The actual flags used by the laboratory included “U,” value less than the MDL (non-detect); “I,” value greater than or equal to MDL but less than PQL; “Q,” actual holding time greater than approved holding time; “T,” actual temperature greater than approved temperature; “F,” QC fail; “D,” delay in receiving sample shipment; “B,” sample amount below minimum required; “N,” not enough sample for analysis. To that list, we added a flag “E,” excessively high concentration considered highly unlikely to be accurate. Those flagged values were identified as more than 2 standard deviations larger than the mean of all samples.
Water Quality and Physiochemical Monitoring
Monitoring of water-quality and physiochemical parameters was performed for several reasons, (1) to quantify arrival times of conservatively transported dissolved constituents and suspended sediment with the gravity wave of water released by the opening of the S-152 culverts, (2) to quantify metabolism based on mass balance modeling of continuously measured dissolved oxygen in the water column, and (3) to assess the strength of vertical mixing of the water column and surface-subsurface exchange using continuous measurements of temperature made vertically through the water column and in the sediment.
Specific conductivity is a potentially useful tracer to quantify the relative speed of the released water in sloughs and ridges and of the rate of mixing between those waters, as well as the transport rate of suspended sediment entrained during transport. During the high flow releases in November 2013, November 2014, and November 2015, continuous measurements of specific conductivity and turbidity were collected by deploying YSI, Inc. (YSI) sondes at selected sites prior to the opening of the culverts and collecting them 4 days later. The turbidity probe has the range of 0 to 1,000 NTU, resolution of 0.1 NTU, and accuracy of ±2 percent (YSI, Inc., 2022a). And the conductivity probe has the range of 0 to 100 mS/cm, resolution of 0.00 to 0.1 mS/cm, and accuracy of ±0.5 percent (YSI, Inc., 2022b). Sondes were deployed at sites RS1U_S, S1, UB1, DB1, DB2, and DB3 in 2013 and Z-51_USGS, RS1U_S, RS1U_R, RS1D_S, RS1D_R, Z5-3B_S, RS1-SE_S, RS1-SE_R, Z5-3NE_S, and Z5-3NE_R in 2014, as seen in table 3. Additional data were attained from USGS stations at S-152 Headwater and Tailwater, and Site 69 East for comparison.
To install the sondes in the water column, 1.5-inch PVC pipes were placed in the peat and the YSI sondes were attached to the exterior of the pipes such that the sensors were approximately at the midpoint of the water column. Sampling occurred autonomously on 5-minute intervals. The YSI turbidity probe is an optical probe with an autonomous cleaning mechanism to prevent biofouling. Before deployment, the turbidity probes were checked in deionized water and if they were not reading within ±2 NTU, a 3-point calibration was performed. The conductivity probes were checked in 250 microsiemens per centimeter (μS/cm) standard. If values were not within ±5 μS/cm, a 1-point calibration was performed. If no calibration was necessary, a 2-point check (50 and 1,000 µS/cm) was performed. Immediately before deployment, all sonde readings were checked against one another in southern Florida tap water.
Dissolved Oxygen and Temperature Modeling
Over several years of high-flow experiments (2016–22), water temperature, and dissolved oxygen concentration were monitored on 30-minute intervals with YSI 6920 V2 sondes, and later with EXO3 Multiparameter sondes, for the purpose of modeling aquatic metabolism.
The sensors were deployed using the aluminum pipes supporting boardwalk structures or PVC pipes such that the sensors were located approximately at the midpoint of the water column. The conductance probes were calibrated with 1,000 μS/cm standard. If values were not within ±20 μS/cm, a 1-point calibration was performed. The YSI specific conductance probe has a resolution of 0.001 to 0.1 mS/cm and accuracy ±0.5 percent of reading plus 0.001 mS/cm, and the EXO specific conductance probe has a resolution of 0.0001 to 0.01 mS/cm and accuracy being the greater of either ±1 percent of the reading or 0.002 mS/cm (YSI, Inc., 2022b, d, e).
The optical dissolved oxygen probes were checked for calibration and recalibrated, if necessary, on each site visit. First, the instrument was checked for calibration by comparing the instrument reading in air-saturated water against the expected reading based on the local temperature and “true” barometric pressure. Barometric pressure (BP) readings from the weather service are corrected for elevation and have to be uncorrected using the following equation: “true” uncorrected BP = (corrected BP)−(2.5*[local altitude in feet above sea level/100]). The Everglades are just 9.8 feet above sea level, indicating that the percent saturation in an air-saturated water sample should read approximately 100 percent saturation ±1 percent or 2 percent in an air-saturated water sample. The acceptable uncertainty is selected based on manufacturer specifications for the YSI DO probe, which measured dissolved oxygen (DO) at a resolution of 0.01 mg/L and an accuracy the greater than ±2 percent of readings, or 0.2 mg/L for the range from 0 to 20 mg/L, and for the EXO DO probe, which has an accuracy that is greater than ±0.1 mg/L, or 1 percent of readings for the range from 0 to 20 mg/L (YSI, Inc., 2022c, e84). Calibration checks were performed during each site visit and recalibrations were conducted whenever necessary. It should also be noted that the YSI temperature probe has a resolution of 0.01 °C and an accuracy of ±0.15 °C, and the EXO temperature probe has a resolution of 0.001 °C and an accuracy of ±0.2 °C (YSI, Inc., 2022b, d).
The period and sites where continuous monitoring of dissolved oxygen, temperature, and specific conductivity was conducted is summarized in table 3. Sondes were positioned in the middle of the water column unless noted. Between November 7, 2018, and March 26, 2019, three sondes were deployed at RS1DS to measure conditions in the upper-third, middle-third, and low-third of the water column. During roughly the same period two sondes were deployed at RS1DR to measure in the upper-half and lower-half of the water column.
Late in the study (July 2021), telemetry systems using a SUTRON SatLink 3 Logger/Transmitter were set up at 3B-350E, 3B-1100S, S633GNU-A, and S633GSU-B. In the processing of ADV data that was accessed from telemetry using AQUARIUS (Aquatic Informatics, 2024), thresholds were implemented so that temperature values below 1 °C and above 40 °C were suppressed, specific conductivity values below 20 µS/cm and above 2,000 µS/cm were suppressed, and dissolved oxygen values below −1 mg/L and above 10 mg/L were suppressed to filter out extremely erroneous data that can result from instrument connection failures. Water quality was measured to understand the effects of changes in flow conditions during the high-flow experiments on metabolism at DPM study sites. Eventually, it will help us to predict the effect of the Everglades restoration activities on the aquatic metabolism processes in the Everglades wetland environment.
Temperature Vertical Profile Measurements
Surface-subsurface water interactions are often difficult to detect in wetlands by hydrometric measurements. Attempts have been made in the Everglades using seepage meters (Choi and Harvey, 2000) as well as measuring the hydraulic conductivity by slug tests in piezometers and using it to estimate the vertical flux by combining it with hydraulic head gradient measurements in piezometers using Darcy’s Law (Harvey and others, 2004). Vertical flux across a peat interface also may be detectable by deploying temperature sensors vertically in sediment and modeling heat transport to constrain the vertical flux of water as discussed by Stallman (1965). Traditional piezometers were installed with four temperature sensors in the DPM experimental footprint in the central Everglades at sites RS1D-S, S1, UB2 and Z51-USGS-S. Each piezometer is made of a 0.5-m-long and 1-inch-wide PVC pipe enclosing four TMCx-HD Water/Soil temperature sensors connected to a single HOBO U-12 4-channel data logger (with a resolution of 0.03 °C at 20 °C and an accuracy of ±0.25 °C) (Onset, 2022). The piezometer was inserted into the peat with temperature sensors located at the floc-peat interface (0 cm depth) and three additional depths within the peat sediment. Three depth configurations were used. Configuration 1 had probes located at 0, 5, 15, and 35 cm below the peat surface. Configuration 2 had probes located at 0, 5, 10, and 15 cm below the peat surface. Configuration 3 varied by site and had a probe at the floc-surface water interface, floc-peat interface (0 cm), at half of the peat thickness (69–87 cm), and at the deepest possible point given the length of pipe (133–143 cm). Each sensor collected temperature every 10 minutes. Monitoring periods ranging from 2013 to 2016 of temperature profiles at several sites performed in this way are shown in table 3. Before installation, measurements in a well-mixed, warm bath were used to compare the accuracy of the sensors. For this controlled experiment, the sensors showed an accuracy of approximately 0.05 °C, which is better than the accuracy reported by the manufacturer.
In a later study, HOBO U-12 4-channel data loggers with TMCx water/soil temperature sensors were also used to monitor temperature in more detail at RS1D-S. To install the temperature sensors in the water column, a 1.5-inch PVC pipe was driven into the peat. From January 9, 2018, to February 1, 2018, 8 temperature sensors spaced 7 cm apart, with the bottom-most temperature sensors located 10 cm above the floc surface, were secured to the PVC pipe. The temperature sensor head was positioned to avoid the direct contact with the pipe. On February 1, 2018, one additional temperature sensor was installed 7 cm above the former top-most temperature sensor, as well as one temperature sensor for air temperature. To reduce the effects of solar radiation on temperature measurements, aluminum foil-wrapped thermal shield was installed over the air-temperature sensor. Furthermore, to reduce the effects of thermal conductance along the wires leading to the temperature sensors, the wiring was wrapped with aluminum foil. Temperature profile measurements were taken in this manner from February 1, 2018, to April 10, 2018.
On April 10, 2018, more temperature sensors were installed in the peat and floc. A wooden stake was driven into the peat, with temperature sensors located 120 cm below the peat surface, 75 cm below the peat surface, 25 cm below the peat surface, 5 cm below the peat surface, 1 cm above the peat surface (in floc), 4 cm above the peat surface (in floc), 7 cm above the peat surface (in floc), and 15 cm above the peat surface (in water column). Temperature profile measurements were taken in this manner from April 10, 2018, to March 26, 2019.
Vertical temperature profiles were used to calculate daily Richardson number values at RS1D-S. The Richardson number is a dimensionless number to express the ratio of the buoyancy term to the flow shear term (Rutherford, 1994). The daily maximum water density gradient between adjacent sensors, distance between the sensors at maximum water density gradient, daily average water velocity, and depth to the middle of the water column are needed to calculate the Richardson number.
where is gravitational acceleration (9.81 meters per square second [m/s2]),Vegetation Measurement
Vegetation community structure is an important factor affecting flow velocity and water depth with concordant effects on velocities and shear stress close to the bed affecting entrainment and transport of suspended sediment (Harvey and others, 2009; Larsen and others, 2009b). Vegetation community composition, biomass (biovolume, including separate analysis of periphyton), and stem densities were determined by harvesting of vegetation in 0.25-square-meter (m2) clip plots and measuring the distribution of stem diameters and frontal areas (Lightbody and Nepf, 2006; Harvey and others, 2009). Within a desired sampling area, the vegetation quadrats were positioned using a stratified random sampling scheme at ridge, slough, and transition zones at all sites within the hydrologic monitoring network. Once the quadrats were situated, the vegetation above the water surface was clipped and bagged as one sampling increment. Below the water surface, vegetation was sampled in 10-cm increments from 2010 to 2012 and 15-cm increments from 2013 to 2014 proceeding from the water surface to the sediment water interface. Sample increments intersecting the bed were clipped at the floc surface, bagged, and stored in the dark and on ice for transport to the laboratory for further processing.
In the laboratory, sample increments were spread out and categorized by species. Measurements of stem diameter and length were collected for the purpose of calculating the average diameter and the frontal area of stems, that is, the exposed area of stem per unit volume in the water column. First, the number of stems and leaves were counted for all rooted species, including the major species Cladium jamaicense, Eleocharis spp., Nymphaea odorata, and minor species Cephalanthus occidentalis, Justicia angusta, and Panicum hemitomon. The width of 10 randomly selected stems were measured for each species, with width being measured as the distance across the middle of each stem fragment along the widest dimension (major axis 1) and across the narrowest dimension (minor axis 2) as measured using a micrometer. For every leaf (or if there were greater than 10 leaves, 10 were randomly chosen) the width, length and thickness were measured using a ruler or micrometer. Frontal area was calculated as:
where a is projected frontal area per unit volume (in cm−1), dAxis1 is average stem diameter of major axis 1 (in cm), dAxis2 is average stem diameter of minor axis 2 (in cm), and n is the number of stems per unit area (in cm−2). The following equation was used to calculate dimensional volume fraction for all species except sawgrass: where V is the volume fraction, for example, vegetation volume per unit volume of wetland (unitless). Owing to the “v” shape cross section of sawgrass, a different equation was used to calculate its volume fraction:Vegetation measurements were made in the vicinity of the key sentinel sites and were repeated at many sites during the early years of experimentation (2010–14) to establish a record of temporal variation related to seasonal and interannual variations and fire. Vegetation-flow relationships are expected to be useful in establishing empirical predictive relationships between biomass and flow resistance parameters to help improve hydraulic models (for example, Harvey and others, 2009). In addition, vegetation measurements were intended to help characterize key ecological processes such as ecosystem primary production, decomposition, and nutrient cycling. Samples were collected in the vicinity of sites to capture ridge and slough characteristics on the dates shown in table 7.
Analysis Results
Hydrologic Response to Experimental High Flows
Introduction
Everglades water resources and ecology have been degraded by a loss of patterned microtopography and vegetation communities that are integral to sustaining water resources and high-quality habitats. Returning high flows to the wetlands is central to Everglades restoration, and is expected to improve water storage, flood conveyance, and ecological habitats. Although restoration has the potential to restore lost functions, but with the potential benefits comes an acknowledgement of risks, for example, propagation of contaminants to downstream areas and continued over drying of Everglades wetlands.
There is a recognized need for physically based modeling of Everglades flow dynamics to account for changes in landscape condition. As primary roughness elements that impede flow, vegetation architecture and microtopography are important in determining water velocities and sediment movement as well as water depth in the Everglades. Biophysical modeling is needed to show how changes in Everglades vegetation and microtopography will affect flow dynamics under future conditions of flow restoration with additional challenges of a changing climate.
Here, we implement a simple flow model in the DPM to assess the relative importance of management factors, specifically the discharge and spreading angle of flow releases through culverts, and to compare those with effects of landscape factors that control velocity and depth of sheet flow, as well as the size of the wetland area where bed sediments are entrained in sloughs and deposited on ridges, a condition favorable for maintaining microtopography and open slough habitats. Landscape factors include vegetation community type and architecture that determine hydraulic roughness, as well as the proportions of ridge and slough vegetation communities and their spatial arrangement including the degree of connectivity of the sloughs. We combine the modeling with observations before and after implementation of the Active Marsh Improvement project that increased slough area and connectivity by removing sawgrass that had invaded historical sloughs. We also model scenarios for a well-functioning Everglades with landscape attributes like the historical ridge and slough landscape.
Methods—Radial Flow Dynamics of S-152 High-Flow Releases in DPM
Flow releases between the large, levee-enclosed basins of the Everglades occur actively through gated culverts or passively through gaps in the levee. Active flow releases through gated culverts concentrate the inflows such that they spread radially into the downstream basin (fig. 3). Therefore, a flow model was needed that could track how a radial pattern of flow interacts with downstream vegetation and landscape characteristics.
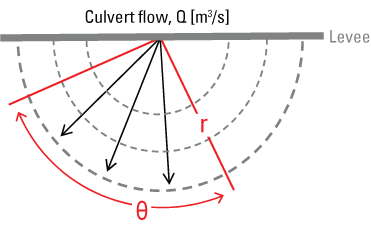
Diagram showing flow release through gated culvert in levee creates a radial pattern of flow spreading across downstream wetland. Arrows indicate that flow discharge per unit width of wetland (q) in meters squared per second (m2/s) is a function of total culvert discharge (Q) in cubic meters per second (m3/s), spreading angle (theta, θ), and radial distance (r).
Governing Equations.—Flow in the DPM is described by a steady-state mass continuity equation that accounts for a radial pattern of water dispersal through the wetland. The continuity equation states that local unit-width discharge, q (in square length over time [L2/T]) declines logarithmically as a function of the radial distance, r in length [L]), total flow through structure, Q (in cubic length over time [L3/T]), and spreading angle, θ (in radians):
and, in terms of the velocity: where U is the vertically integrated flow velocity in length over time [L/T], h is water depth in length [L].To model the flow in terms of wetland properties that control how the flow spreads, we use the biophysical flow rate expression (BioFRE) of Harvey and Choi (2022a). The biophysical flow rate expression is distinguished from other rate expressions like Manning’s rate law in being parameterized from field measurements rather than calibrated versus field-measured water velocities. Using BioFRE can help anticipate how changing wetland conditions will affect flow and how it is partitioned between a change in flow velocity and a change in flow depth. The BioFRE equation is:
and, in terms of velocity: where neff is the upscaled effective roughness coefficient for the wetland landscape (in seconds over meters raised to the 1/3 power [s/m1/3], S is water slope in meters over meters [m/m]), β is the exponent of water depth, for which a value of 0.667 was used, and α is exponent of water slope, for which a value of 1 was used for consistency with previous simulations in the Everglades.The BioFRE rate law expression uses the effective upscaled roughness coefficient for the landscape from field measurements. For example, the depth-dependent roughness of ridge and slough vegetation was calculated from a force balance equation (Harvey and others, 2009) based on theory (Nepf, 1999) and measurements of stem frontal area and stem diameter (Larsen and others, 2009b). Furthermore, those vegetation community specific roughnesses are upscaled to represent an effective landscape roughness (neff) based on the scaling analysis of Larsen and others (2017a) for which we used estimates of aerial coverage, p, microtopographic height difference between ridge and slough communities (zp) fractal dimension (fd), and directional connectivity (DCI), of the slough-ridge landscape pattern (Harvey and others, 2022b). Flow is a power function of water depth and slope for which the exponents, 1 for α and 0.667 for β, were selected to be consistent with previous Everglades modeling (Said and Brown, 2013; Lal and others, 2015).
To use BioFRE expression for radial flow modeling in DPM, the BioFRE equation (28) was substituted into the mass continuity equation (26) to yield a governing equation for wetland flow velocity from a point release into a radial flow field:
where the predicted water depth is:We used the resulting governing equations in radial coordinates to investigate the relative importance of the wetland variables neff and S and the human-managed variables Q and θ in controlling outcomes for U, h, and resulting changes over time, including sediment dynamics, that can determine the effectiveness of high-flow restoration in the Everglades. The total culvert discharge (Q) and the radial spreading angle (θ) are largely under the control of human engineering projects and larger-scale patterns of water availability. In contrast, the hydraulic variables (neff, S, and h) depend on vegetation and landscape conditions (for example, ridge proportion and associated microtopography), which can evolve over time because of changes in the managed variables. For example, a change in Q or θ will interact with the effective roughness (neff) in ways that adjust water slope (S) and water depth (h). In turn, any change in water depth h will feed back by favoring increases or decreases in vegetation stem density or invasion by new vegetation species (Larsen and Harvey, 2011). Structural changes in vegetation communities will further alter hydraulic variables neff, S, and h through biophysical feedbacks that operate on relatively short timescales of just a few years. Because of the interdependencies among variables, we performed many simulations to investigate how various combinations of managed variables and vegetation and landscape conditions can affect future hydrologic and ecological conditions in the Everglades. Although challenging to model, the delicate balance between managed flows and vegetation and landscape conditions is a primary driver of the Everglades ecosystem function and health.
The BioFRE is uniquely capable of simulating changes that could occur in the hydroecology of the Everglades. Unlike the typical hydrologic models used in the Everglades, the effective roughness parameter of BioFRE is calculated from vegetation and landscape measurements rather than being adjusted by trial and error to fit hydrologic observations. As a result, BioFRE can help anticipate future changes brought about by the interdependencies discussed earlier. BioFRE simulations can be verified by comparing simulation against independent observations of hydrology that were not used as inputs to BioFRE. The system-wide hydrologic observations that we used for comparisons are reported in Harvey and Choi (2022a) and Harvey and Choi (2022b), along with the more detailed BioFRE simulations for DPM that we describe in the present document and in Choi and Harvey (2023).
For the present study we used the BioFRE to simulate overland flow in the DPM over the ten-year period of experimental high-flow releases. We used the simulations to assess the relative importance of changing water supply caused by year-to-year precipitation variability and high-flow releases through the S-152 culvert versus changes in wetland vegetation and related landscape factors that control outcomes such as water depth, a master variable that determines Everglades health. We focused on understanding how water velocity was affected, because it has immediate effects on sediment entrainment and downstream delivery of sediment-associated contaminants, such as phosphorus. The product of depth and velocity is the unit-width discharge, which is also an important outcome that controls the rate of downstream transport of sediment and phosphorus.
Three major groups of BioFRE simulations were undertaken for DPM: (1) DPM simulations of observed high flow, (2) DPM scenario simulations that held total discharge constant and varied landscape conditions, and (3) DPM sensitivity analyses that examined the relative importance of factors driving degradation or restoration of wetland functions.
Simulation group 1 tracked observed high-flow conditions in the DPM based on the observations between 2013 and 2020, during which the DPM overland flow conditions varied as a function of changing S-152 culvert discharges as well as changes in wetland landscape resulting from AMI implementation. Simulation group 2 isolated the effects of AMI implementation by holding culvert discharge constant as well as simulating several full restoration scenarios that decreased ridge proportion to historical, pre-drainage levels and altered slough vegetation type. Simulation group 3 quantified sensitivity of the simulation outputs with respect to inputs, as a means to understand the relative importance of the different drivers of Everglades degradation and restoration. Data sources and estimation procedures to create inputs for the three DPM simulation groups are presented below.
Group 1 simulations of observed high-flow releases.—DPM overland flow was simulated for eight steady high flow releases between 2013 and 2021. Simulation group 1 contrasted pre- and post-implementation of the AMI project. Four measurement periods occurred prior to AMI (pre-AMI periods 1, 2, 3, 4) and four occurred after (post-AMI periods 6, 7, 8, 10). Each measurement period also differed in total culvert discharge, ranging from 5.30 to 11.04 m3/s as well as in water surface slopes (table 8).
Eight of the eleven high-flow releases had periods of a month or more when the total culvert discharge was judged to be steady enough for our analysis. To calculate the water slope during each period, a logarithmic function was regressed to achieve a best match to measured surface water elevations for each period as a function of distance from the S-152 culvert. At each distance, slope was computed by taking the derivative of the regressed logarithmic function from table 8. For situations where surface water elevations were not available at an important target site during a measurement period, we used a regression between S-152 discharge and the offset in water elevation between RS1D and that target site, measured at times when both were available, to estimate the missing surface water elevations. The regression parameters used were regression slope coefficients 0.00013, 0.0001198, and 0.00018, respectively, and intercepts 0.08998, 0.02222, and 0.07577, respectively, to predict water surface elevations at Z51_USGS, RS2, and UB2.
Table 8.
Water slope calculation using measured surface water elevations at DPM.[Four periods occurred prior to the Active Marsh Improvement (AMI) project (pre-AMI periods 1, 2, 3, and 4) and four occurred after the project (post-AMI periods 6, 7, 8, and 10). Date ranges in the format of month/day/year. For the example surface water slope estimates at RS1D, radial distance (r) is 446 meters. Small numbers are expressed in exponential notation (E-notation). m3/s, cubic meter per second; SE, surface water elevation; S, surface water slope; WSC, water slope coefficient]
Effective upscaled roughness (neff) for simulation group 1 was calculated based on observations of vegetation architecture, microtopography, slough water depth, and related landscape parameters, including fractal dimension of ridge-slough edges (fd) and directional connectivity index (DCI) (Choi and Harvey, 2023; Larsen and others, 2017a). Effective roughness was affected beginning in late 2016 by implementation of the AMI project that opened two historical sloughs in the DPM. The pre-AMI ridge proportion (0.835) was relatively high and was lowered to a post-AMI ridge proportion of 0.730 by the AMI project’s removal of sawgrass from several historical sloughs. To account for the slough openings in calculation of effective upscaled roughness, we adjusted the pre-AMI binarized ridge and slough map by reassigning the opened historical sloughs as sloughs rather than ridges, which adjusted the ridge proportion (p) in the southern DPM flow way. Other needed inputs, such as fractal dimension of ridge-slough edges (fd) and DCI were re-computed using the correlations between p and other metrics established previously by Harvey and Choi (2022a). Those observations are used to create inputs using equations of Larsen and others (2017a) to recompute effective roughness, as documented in Harvey and Choi (2022a).
Table 9 reports the measurements used to calculate effective upscaled roughness for the eight selected measurement periods of steady high flow in the DPM. Effective roughness was calculated from microtopographic height, ridge proportion, directional connectivity, fractal dimension, and ridge and slough vegetation roughness using the equation outlined in Harvey and Choi (2022a). The ridge-slough microtopographic height difference was held constant for all simulations, but the landscape pattern parameters (p, DCI, fd,) were adjusted between pre- and post-AMI periods. Ridge proportion (p) reflects how the relatively high pre-AMI ridge proportion during the first four periods was lowered somewhat by the AMI project opening of two sloughs for the latter four post-AMI periods. Values of fractal dimension (fd) and DCI were adjusted to account for AMI effects using the correlations of those parameters with p (Harvey and Choi, 2022a).
Table 9.
Inputs for calculating effective upscaled roughness coefficients for group 1 BioFRE simulations.[Input parameters are from Harvey and Choi (2022b) and Choi and Harvey (2023) except where indicated. The measured slough water depth was used to select depth-dependent vegetation roughness. Small numbers are expressed in exponential notation (E-notation). cm, centimeter; DSP, dense spikerush with periphyton; DS, dense spikerush; SS, sparse spikerush; m, meter; AMI, Active Marsh Improvement project]
In summary, 24 sets of input data were prepared for simulation group 1. The simulations predicted overland flow through the DPM for eight flow periods by accounting for effects of various combinations of culvert discharge, landscape conditions such as slough area that was affected by the AMI project), and particular type of vegetation present in the sloughs (for example, dense spikerush with periphyton [DSP], dense spikerush [DS], and sparse spikerush [SS]). Each period had a different average culvert discharge, Q, through the S-152 culvert that spread radially with a spreading angle(θ) that was presumed to be constant across simulations. Water slope for the simulations was calculated from observed surface-water elevations during each period being simulated. Effective upscaled roughness for the simulations was estimated for each period based on observed vegetation-type landscape conditions. The measured average water depth in sloughs in the simulations for each period allowed us to select the appropriate depth-dependent estimates of ridge and slough vegetation roughness. The type of slough vegetation can vary over time, and we tested the influence of slough vegetation type by repeating each period’s simulation three times to reflect how slough vegetation influenced the effective roughness (table 9). The input parameters for all 24 group 1 simulations are provided in table 10.
Table 10.
Inputs for group 1 simulations of observed DPM high-flow releases.[Slope is slope coefficient over radial distance (SC/r). Effective Roughness (neff) is calculated from vegetation and landscape properties. Small numbers are expressed in exponential notation (E-notation). m3/s, cubic meter per second; rad., radian; m, meter; DSP, dense spikerush with periphyton in sloughs; DS, dense spikerush in sloughs; SS, sparse spikerush in sloughs; AMI, Active Marsh Improvement project]
Group 2 simulations of DPM high-flow scenarios.—The S-152 culvert discharge was held to a constant value of 7.38 m3/s (average of eight high-flow periods) for the purpose of investigating vegetation and landscape effects on overland flow. In contrast to group 1, where measured surface-water slopes were used as inputs for simulations, water slope needed to be estimated for the unobserved conditions for group 2. To predict water slope for pre- and post-AMI scenarios, we used the average of the four water slopes from table 8 as estimates for pre-AMI scenarios and the average of the four post-AMI cases from table 8 as estimates for post-AMI scenarios (table 11). For fully restored slough area scenarios, we estimated water slope by extrapolating from a linear regression that related measured water slopes at RS1D, RS2, and UB2 for eight periods (table 8) against the appropriate pre- and post-AMI ridge proportions (0.835 and 0.730, respectively). The resulting regression equation was used to estimate surface water slopes at RS1D, RS2 and UB2 based on best-fit regression slope coefficients 6.2434 E−05, 1.9799 E−05, and 1.0752 E−05, respectively, and intercepts 1.3376 E−04, 4.2418 E−05, and 2.3035 E−05, respectively. When the predicted slopes were multiplied by distances(r) the resulting water slope coefficients were 0.0837 for the flour pre-AMI scenarios and 0.0807 for the four post-AMI scenarios.
We compared group 2 scenarios to assess outcomes for several important variables affecting Everglades wetland health, that is, water depth and sediment transport conditions. For depth, it is well understood that water depth must remain high enough to preserve diverse ridge and slough wetland communities (for example, see Choi and Harvey, 2017). For sediment transport, we assessed outcomes to determine how the area of sediment entrainment from both ridges and sloughs changes, as well as how acres of sediment redistribution from sloughs to ridges (that is, sediment entrainment in sloughs and sediment deposition on ridges) changed. The thresholds for both sediment transport metrics were assessed with velocity criteria based on the experimental observations and modeling results for sediment transport in the Everglades by Harvey and others (2011) and Larsen and others (2009b). Slough velocities of 1.5–3.0 cm/s delimit a typical range wherein sediments are “redistributed” from slough to ridge, meaning that sediments are mobilized in sloughs and transported until they are deposited on ridges. Fine particulate sediments are mobilized by entrainment from their points of attachment to surfaces of floating vegetation and associated periphyton mats, or attachment to stems of rooted vegetation and associated epiphyton coatings (Harvey et al., 2011). At the higher end of the velocity range there also may be entrainment of flocculent organic sediments from the bed. Above a velocity of 3.0 cm/s, sediments are typically entrained in both slough and ridge, and below a velocity of 1.5 cm/s, sediments are not entrained in either ridge or slough (Larsen and others, 2009b).
The last of the group 2 scenarios anticipated how a fully restored slough area, which lowers effective roughness, interacts with total culvert discharge. We raised total culvert discharge by almost a factor of five, from the average observed value of 7.28 m3/s to 35 m3/s, in order to anticipate how much the culvert discharge would have to increase in order to sustain a slough water depth greater than 50 cm in a fully restored DPM where ridge proportion was decreased from its present-day value of 0.735 to 0.421. However, to complete that simulation, we needed an estimate for the water surface slope coefficient, which was uncertain. Since both our observations (table 8) and our governing equation tell us that surface-water slope depends on total culvert discharge, we estimated the water slope coefficient based on the extrapolation of a regression between measured slope coefficient and culvert discharges using all of the observed data for all eleven of the high flow periods (table 8). The estimated Slope Coefficient was 0.155 based on the estimation equation for Q (35 m3/s) which was 0.072+0.003×(35 m3/s−7.38 m3/s ) = 0.155, where 0.072 is the Slope Coefficient that we had estimated for fully restored slough area (Q=7.38 m3/s), and 0.003 is the proportionality between discharge through the S-152 culvert structure (Q) and 11 measured slope coefficients (table 8). Table 11 summarizes the measurements that were used to estimate inputs for the BioFRE base simulations at DPM.
Table 11.
Inputs for effective upscaled roughness calculations for group 2 scenario simulations of DPM high flow.[Scenario name includes acronym for slough vegetation type. Small numbers are expressed in exponential notation (E-notation). cm, centimeter; m, meter; AMI, Active Marsh Improvement project]
Estimating the effective upscaled roughness for group 2 scenario simulation required an estimate of water depth in sloughs. For the pre- and post-AMI base case simulations, we used the average measured water depths for pre- and post-AMI bases cases as the estimate for water depth. For the unobserved bases cases for fully restored conditions, there are no estimates of water depths to use. Therefore, we began by using a guess for water depth that was needed to establish an initial estimate of effective roughness. Those estimates were both updated using an iteration approach that after 25 steps converged on a final solution for water depth and effective roughness. The resulting outcomes for a final simulated water depth had an iteration uncertainty of between 0.2 and 3.2 cm, which was judged to be satisfactory for our purposes in performing the base-case simulations.
Table 12 provides the input parameters for the group 2 scenario simulations of DPM high flow. Simulations 1–7 held culvert discharge constant to illustrate effects of the AMI project compared with full restoration of the slough area. Simulation 8 increased culvert discharge to answer the question, “how much more flow would be needed to avoid shallow water depths and overly dry conditions in a fully restored Everglades landscape?”
Table 12.
Inputs for group 2 scenario simulations of DPM high flow.[Scenario name includes acronym for slough vegetation type. Small numbers are expressed in exponential notation (E-notation). m3/s, cubic meter per second; cm, centimeter; rad., radian; AMI, Active Marsh Improvement project]
Group 3 sensitivity analysis of DPM simulations.—We computed sensitivity for DPM high flow simulations with a purpose to assess the relative importance of controlling factors, some management related and some related to landscape conditions, that together influence the pathways and speed of degradation and restoration processes in the Everglades. We used a local sensitivity approach in which the input variables Q and θ represented management related controls on overland flow, as opposed to the landscape variables ridge proportion, directional connectivity, and fractal dimension, microtopographic height, and vegetation roughness parameters ns and nr. The effective roughness, neff incorporates all controls imposed by landscape, microtopography, and vegetation conditions. Inputs were perturbed one at a time, with perturbations being small, usually an amount equal to 20 percent of the observed range of the input variable (table 12). Ridge proportion (p) was perturbed by an amount equal to 20 percent of its measured range in 23 Everglades landscapes; directional connectivity (DCI) and fractal dimension (fd) were perturbed using a regression equation that predicted the change in those landscape metrics based on the 20 percent change in the ridge proportion previously described. Microtopography (Zp) was perturbed by 20 percent of the range of area-averaged microtopographic height at 57 locations (Harvey and Choi, 2022b). For the base case using a culvert discharge of 7.38 m3/s, the discharge was perturbed by 20 percent of the range of selected 8 periods for restoring direction. For the base case using a discharge of 35 m3/s, the discharge was perturbed by 20 percent of the difference between 35 and 7.38 m3/s. For both base cases, the spreading angle was perturbed by 20 percent of the base case’s spreading angle (90 degrees).
For each perturbation we re-ran the simulation to quantify the relative change in several simulation outputs and computed dimensionless sensitivity of resulting outcomes as:
where “objective” is the value of a simulation output of interest. Here, the objectives of interest were the simulated water depth, sediment entrainment area, and sediment redistribution area. Computations of dimensionless sensitivity were compared and ranked by importance.A local sensitivity analysis approach is valid for situations that are similar to the tested base case. For that reason, we performed sensitivity testing for two relevant endmember scenarios: the first scenario representing a poorly functioning but potentially restorable landscape. To do this, we used the pre-AMI simulation with dense spikerush and periphyton in sloughs (see scenario 1 from table 12) as the base case, and we refer to it as the “restoring” test because we perturbed inputs in a direction to improve wetland function. The second scenario represented a well-functioning, fully restored landscape that can be degraded by lowering of water depth and other factors. For that, we used the fully restored simulation (see scenario 8 from table 12) with dense spikerush and periphyton in sloughs as the base case, and we refer to it as the “degrading” test because we perturbed inputs in a direction that would degrade wetland function (table 13).
Table 13.
Sensitivity analysis bases cases and perturbations for the restoring and degrading DPM wetland scenarios.[Restoring base case represents the degraded DPM landscape conditions that were observed prior to the Active Marsh Improvement project (pre-AMI) with dense spikerush and periphyton in sloughs. Degrading base case represents a fully restored DPM scenario with dense spikerush and periphyton in sloughs. Sensitivity is tested by perturbing base case input variables as shown in red. The perturbations adjusted the input variables by an amount equal to 20 percent of the measured range of that variable in the Everglades (Harvey and Choi, 2022a, b)—see text for explanation. Small numbers are expressed in exponential notation (E-notation). m3/s, cubic meter per second; cm, centimeter; s/m1/3, second over meter raised to the one-third power]
Results and Discussion
Simulating high-flow releases and overland flow through the DPM makes use of BioFRE equations that are appropriate for radial flow to estimate how point-source releases through culverts spread through downstream wetlands. The simulations can help anticipate future restoration outcomes for water availability and ecosystem health of the Everglades. Before discussing those uses, we evaluate the performance of BioFRE in simulating observed hydrologic conditions.
Group 1 Simulations of Observed DPM High-Flow Releases
The simulated flow velocities at DPM for eight measurement periods of steady high flow generally agreed with observed flow velocities in demonstrating a logarithmic pattern of decreasing flow velocities with distance from the S-152 culvert inflow (figs. 4–9). Simulated velocities in the sloughs tended to be lower than observed velocities, especially after the AMI project re-opening of two historical sloughs. BioFRE simulates spatially averaged flow velocities, and those were more similar to observed velocities in ridges. Higher observed velocities in sloughs makes sense given that the roughness of sloughs is much lower (table 11), and the landscape is mostly comprised (approximately 80 percent) of ridges (table 11). Also apparent from simulations in figures 4–9 is that differences between slough vegetation communities, that is, dense spikerush with periphyton (DSP), versus dense spikerush (DS), versus sparse spikerush (SS), had no apparent impact on flow velocity, likely because sloughs comprised such a small area in DPM, approximately 20 percent (table 11).
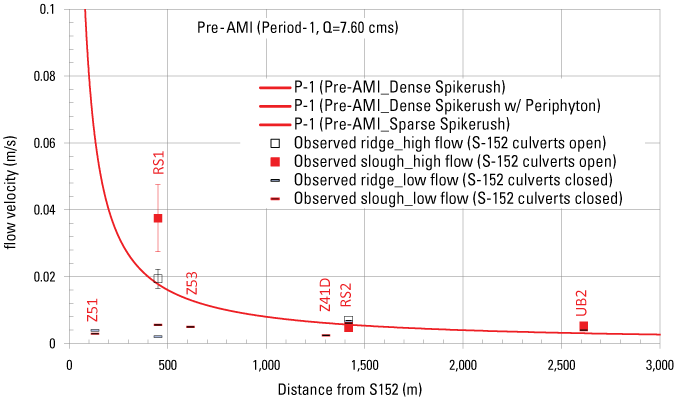
Plot showing period 1 (pre-AMI) velocities versus distance for three different slough vegetation types compared with measured flow speeds at monitoring sites. cms, cubic meter per second; m, meter; m/s, meter per second; Q, discharge.
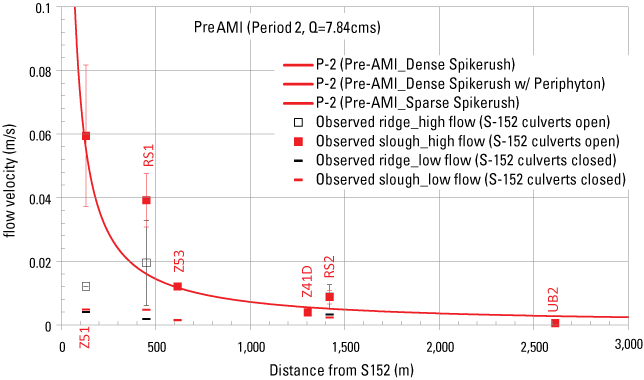
Plot showing period 2 (pre-AMI) velocities versus distance for three different slough vegetation types compared with measured flow speeds at monitoring sites. cms, cubic meter per second; m, meter; m/s, meter per second; Q, discharge.
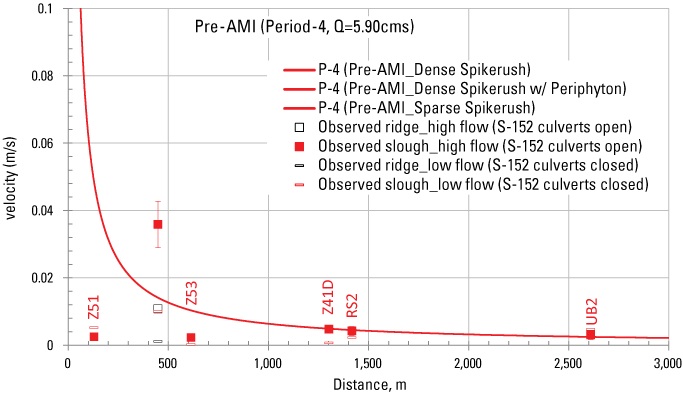
Plot showing period 4 (pre-AMI) velocities versus distance for three different slough vegetation types compared with measured flow speeds at monitoring sites. cms, cubic meter per second; m, meter; m/s, meter per second; Q, discharge.
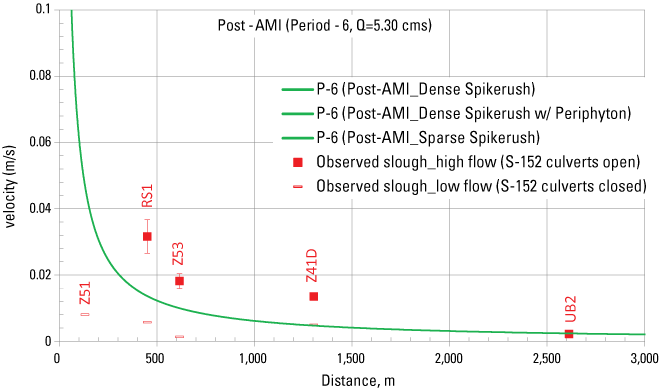
Plot showing period 6 (post-AMI) velocities versus distance for three different slough vegetation types compared with measured flow speeds at monitoring sites. cms, cubic meter per second; m, meter; m/s, meter per second; Q, discharge.
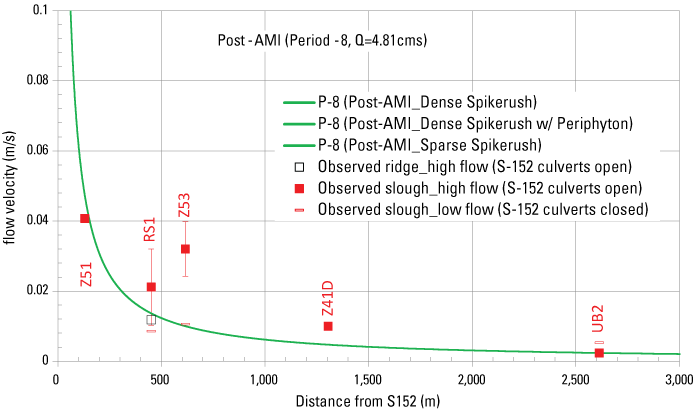
Plot showing period 8 (post-AMI] velocities versus distance for three different slough vegetation types compared with measured flow speeds at monitoring sites. cms, cubic meter per second; m, meter; m/s, meter per second; Q, discharge.
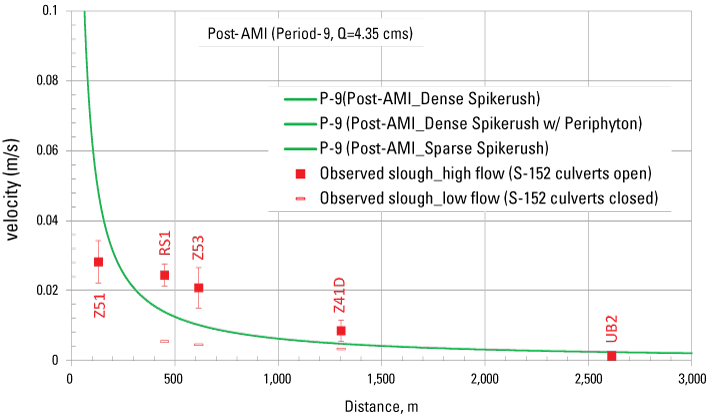
Plot showing period 9 (post-AMI) velocities versus distance for three different slough vegetation types compared with measured flow speeds at monitoring sites. cms, cubic meter per second; m, meter; m/s, meter per second; Q, discharge.
As a second indicator of BioFRE performance, we checked for agreement between water depths calculated from equation 30 and observed slough water depths. BioFRE simulated water depths varied between 0.4 and 1.1 m. There was general agreement with measured water depths however the measured water depths varied less, between 0.6 and 0.85 (fig. 10).
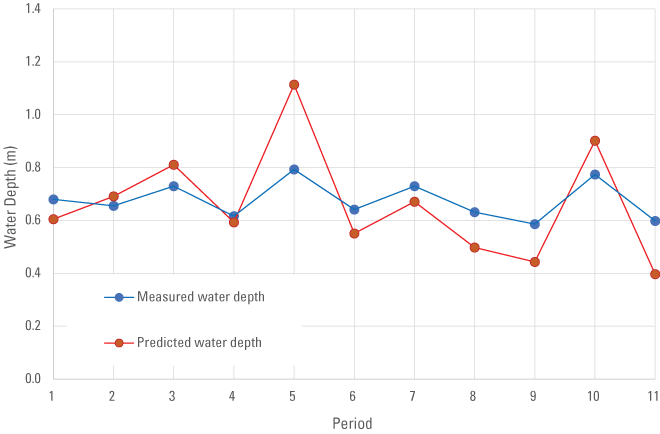
Plot showing time series of measured versus BioFRE-simulated slough water depth during steady high flow at DPM. m, meter.
Simulated water depths therefore underpredicted the measurements when conditions were dry, when measured water depths were below 0.7 m. Simulated water depths overpredicted the measurements when conditions were wet, when measured water depths were above 0.8 m (figure 11). The more stable measured water depths may be the result of backwater effects affected by the restriction of the flow through a gap in the L-67C levee located 2.5 km downstream, or by backwater effects caused by pooled waters in WCA 3B.
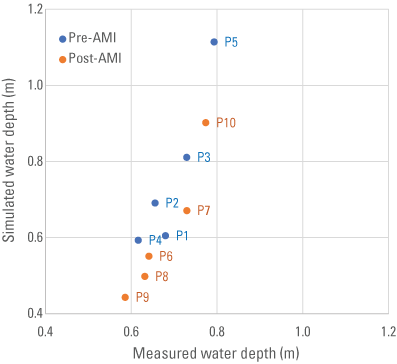
Plot showing BioFRE simulated versus measured slough water depth during steady high flow periods at DPM. m, meter.
How did the DPM AMI project affect overland flow in the DPM? The AMI project reconnected two historical sloughs by removing invasive sawgrass over a length of more than 2 km. Removal of invasive sawgrass was expected to increase flow velocities in sloughs in the post-AMI period (period 6 and after) compared to the flow velocities measured in the pre-AMI simulations (periods 1–4). We examined observed and simulated flows for an indication that the AMI project increased flow velocities. However, the BioFRE simulations of post-AMI velocities differed little compared to pre-AMI simulations (see figures 4–6 for pre-AMI velocities versus figure 7–9 for post-AMI velocities), likely because post-AMI culvert discharges were generally lower, and the post-AMI effective roughness values also were lower, both of which have counteracting effects on velocity. In other words, the lower post-AMI culvert discharges should have lowered post-AMI flow velocities, whereas the lower post-AMI effective roughness should have increased flow velocities, which may have had counterbalancing effects. In support of that interpretation, note that the BioFRE simulations for periods pre-AMI period 2 and post-AMI period 7, which had similar culvert discharges, indicated only minor changes in simulated velocities, suggesting that flow velocities were only slightly affected by the AMI project.
A more detailed comparison of pre- and post-AMI simulations of observed high flows would be difficult because of the culvert discharge variation across periods as well as AMI project decreases in effective roughness caused by opening the sloughs and decreasing average ridge proportion and directional connectivity and fractal dimension of sloughs (see table 11). Regarding the comparison with measured velocities, there was an issue that very few velocity measurements were made in ridges after AMI implementation. Instead, most water depth measurements after AMI were made in the directly affected sloughs, which likely overestimate the landscape-averaged flow velocities.
A question of interest for Everglades restoration is whether variability in culvert discharge or variability in landscape character, for example, ridge proportion and so forth, was more important in controlling downstream velocity. In the next section, AMI project effects are further examined in what we are referring to as scenario simulations that, in some cases, hold culvert discharge constant to control for the positive effect of higher discharge on flow velocity. In other cases, landscape condition is held constant and culvert discharge is varied, to assess the relative importance of managed hydrologic inflows versus landscape conditions in controlling resulting water depth, velocities, and sediment transport dynamics.
In summary, the overall concurrence between simulations and observations suggests a strong logarithmic decline occurs in flow velocities as a function of distance from the inflow culvert, with dependencies on average culvert flow and on average landscape condition influencing the effective roughness. General agreement between observed and simulated velocities and water depths supports the use of BioFRE to approximate DPM flow conditions without calibration to fit hydrologic observations. As a result, BioFRE appears to be a useful tool for exploring the relative importance of factors controlling restored high flows in the Everglades.
Group 2 Scenario Simulations of DPM High Flows
Overland flow in the present-day DPM and possible future conditions were compared in the group 2 scenario simulations. The purpose was to isolate individual controls of vegetation and landscape factors and separate them from controls by water inflows through a culvert. To accomplish that, some scenarios held management factors including S-152 culvert discharge and flow spreading angle constant, and others varied discharge. Several scenarios tracked the AMI project’s re-opening of two sloughs that decreased the ridge proportion in DPM from 84 percent to 73 percent. In addition, other scenarios were created to anticipate what could happen if ridge proportion was decreased all the way to the historical proportion of 42 percent. Figure 12 shows the resulting flow velocities for all scenarios. The scenarios were compared to understand possible restoration outcomes, including changes in water depth and flow velocities, both of which have significant implications for Everglades water resources and ecosystem health.
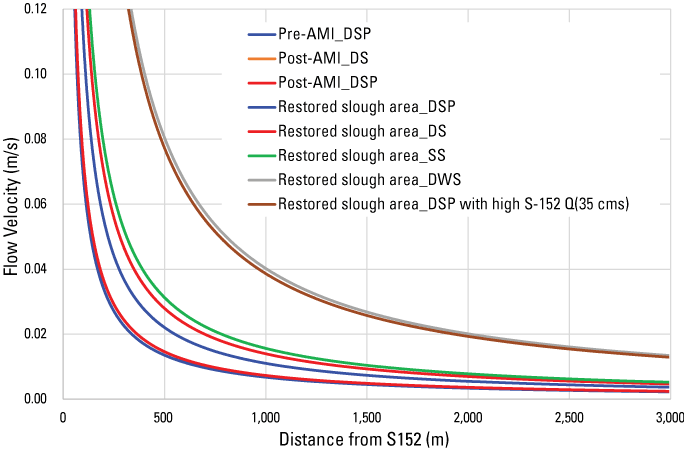
Plot showing BioFRE simulated scenarios for DPM water velocities as a function of distance downstream from the S-152 culverts. All simulations have the same culvert discharge (7.38 cubic meters per second) except for the last simulation, which had a culvert discharge of 35.0 cubic meters per second. cms, cubic meter per second; m, meter; m/s, meter per second.
Three of the group 2 scenarios examined present-day conditions (pre- and post-AMI) and four scenarios examined fully restored slough area outcomes under the same present-day culvert inflows. The last scenario increased the culvert discharge to examine how much more flow would be needed than average present-day flows at DPM to counterbalance the substantial decrease in water depth that was predicted by the other fully restored slough area scenarios.
The type of slough vegetation mattered little when ridge proportion was high (p>0.7; tables 8 and 14). For example, simulations with different slough vegetation have essentially identical velocities (table 12, figs. 4–9). However, for the fully restored slough area simulations where ridge proportion was lowered to the historical pre-drainage value of 0.42, the type of slough vegetation substantially affected the sediment redistribution area (tables 8 and 14). Slough vegetation type, therefore, matters considerably for fully restored slough area landscapes in the Everglades.
The sediment entrainment area increased substantially, depending on the type of slough vegetation, by a factor ranging from 2 to 30, in the fully restored scenarios compared to present-day, pre- and post-AMI scenarios (table 14). Importantly, the simulated water depths declined in the fully restored slough area simulations to a level from 50 to 20 percent of the present-day water depths (table 14). Therefore, in the fully restored slough area scenarios, the positive effect of greater flow is balanced against the negative effects of lowered water depths and larger areas of sediment entrainment. However, the area of sediment redistribution increased by even more than the area of sediment entrainment. Greater areas of sediment redistribution may be a positive for maintaining microtopography that sustains sloughs, although, those substantial changes all occurring together would need to be carefully evaluated in adaptive management efforts in Everglades restoration.
Table 14.
Water depth and sediment transport outcomes of BioFRE group 2 scenario simulations.[Scenario name includes acronym for slough vegetation type; DSP, dense Spikerush with periphyton; DS, dense Spikerush; SS, sparse Spikerush; DWS, deep water slough. Simulations 1–8 held total culvert discharge (Q) constant at 7.43 cubic meters per second (m3/s) to compare outcomes for various landscape conditions. Water velocity and unit-width discharge for each simulation are compared at a single site (RS1D). Simulation 8 increased Q from 7.43 to 35 cubic meters per second for a fully restored slough area to understand how much flow was needed to avoid overdrying of DPM wetlands. m, meter; m/s, meter per second; m2/s, square meter per second; AMI, Active Marsh Improvement project]
Group 3 Sensitivity Testing for Restoring and Degrading Scenarios
Two of the eight scenario simulations were selected for sensitivity analysis. For both the restoring and degrading sensitivity tests it was vegetation roughness that was the most sensitive control on sediment entrainment, water depth, water velocity, and unit-width discharge. Ridge roughness was the most sensitive variable controlling the outcomes of the restoring and slough vegetation roughness was the most sensitive variable controlling the outcomes of the degrading scenarios. Next in the order of relative importance were the management variables, that is, flow spreading angle and S-152 culvert discharge that were approximately equivalent in their sensitivity. Those variables were followed by the “landscape pattern” variables and microtopography which were least important for these simulations (table 15). The landscape pattern variables and microtopographic height were relatively less important in these simulations because the tested water depths (71 and 58.6 cm for slough depths for the restoring and degrading simulations, respectively, were relatively deep and typical for high-flow periods in DPM [table 15]). The sensitivity of landscape pattern variables and microtopographic height can rank near the top for lower water depths (Harvey and Choi, 2022a), especially when slough water depths are just slightly above or below the microtopographic height of ridge tops above slough bottoms.
Water depth and sediment entrainment were strongly sensitive to restoration of landscape pattern variables. Water depth declined and sediment entrainment area increased with decreasing ridge proportion, increasing directional connectivity of sloughs, and increasing fractal dimension of ridge-slough edges (table 15). In contrast, water depth increased, and sediment entrainment area decreased in a restored slough area simulation that was tested for degrading drivers, for example, increased ridge proportion and lowered DCI and ridge-slough fractal area. These sensitivity results emphasize that important outcomes such as water depth and sediment entrainment area may respond in non-obvious or undesirable ways to drivers that are assumed to restore or to degrade functional values of the Everglades.
Table 15.
Sensitivity test results for a pre-AMI landscape and a restored slough area landscape.[Sensitivities greater than (>) 15 percent (%) are in red. <, less than; —, no data; U, water velocity; m/s, meter per second; m2/s, square meter per second; AMI, Active Marsh Improvement project; p, ridge proportion; DCI, directional connectivity; fd, fractal dimension; a, projected frontal area per unit volume, 1/centimeter; d, average stem diameter; centimeter; Q, discharge, cubic meter per second]
Summary
We used BioFRE to simulate sheet flow and water depth adjustments in a changing restoration landscape in the Everglades. Unlike most hydrologic models, BioFRE is based on fundamental physical drivers and can therefore be applied without calibrating to hydrologic data, making it useful for anticipating future changes in flow inputs and related management variables, as well as responses to changing vegetation and landscape conditions. The BioFRE simulations identified relative roles of flow, vegetation, landscape, and management variables that are likely to pose challenges in CEPP-South.
The BioFRE simulations examined conditions that promote positive restoration outcomes, including increased flows, water depths, and wetland connectivity that improve water availability and ecological habitat associated with greater slough area. Simulations indicated what combinations of culvert flow and landscape conditions could maintain water depths that are appropriately deep with small amounts of high velocity areas to minimize entrainment of sediment and sediment-associated phosphorus and transport to downstream areas. Where sediment is entrained in sloughs, the ecosystem functions best if it is redistributed and deposited on nearby ridges, which sustains the microtopography that supports productive slough habitats.
The pre- to post-AMI scenarios indicated that AMI likely increased sediment entrainment slightly along with decreasing water depths slightly. Those slightly negative outcomes may be a reasonable tradeoff for the AMI lowering or ridge proportion from 0.835 to 0.73, which locally increased the slough area by approximately 60 percent in the DPM southern flow way. In contrast, the DPM scenario simulations of fully restored slough area anticipated that sediment entrainment area could increase substantially, and water depth could also decrease substantially, with the magnitudes depending strongly on the slough vegetation type present. The simulated increase in sediment entrainment area and decrease in water depth would be counterproductive for restoration if they occurred at the DPM. A simulation addressing “how much water would be necessary to sustain water depths in a wetland with fully restored slough area suggested that the answer was “more than is available”. Therefore, with the likely limitations of clean water to restore high flows in a fully restored wetland landscape and given the likely capacity of the presently planned culverts in the DPM area, care is warranted not to fully restore “historic” slough areas by decreasing ridge area to 42 percent unless more water becomes available and more capacity to deliver the water becomes available, to control negative impacts on water depth and sediment entrainment.
It is important to note that BioFRE results are preliminary and should be checked with dynamic simulations that could implement the biophysical basis of BioFRE. The BioFRE simulation approach is entirely compatible with SFWMD Regional Simulation Model (SFWMD, 2005) and with other overland flow models, and therefore can be useful for forecasting and adaptive management, for example, developing performance measures for documenting and adjusting restoration projects to achieve desired outcomes for water and ecological resources of the Everglades.
Transport and Fate of Suspended Sediment and Total Phosphorus under High Flow
Introduction
A loss of high flows through a patterned landscape with microtopographic variation and ridge and slough vegetation communities is thought to degrade ecosystem functions, as well as diminish water storage during droughts and diminish the capacity for wetlands to convey flood waters without drowning tree islands or putting urban communities at risk. Modeling supports a hypothesis that high flows entrain organic sediments in sloughs and deposit them on ridges, a process described as sediment redistribution. The process has been investigated in small laboratory flumes and flumes installed in the Everglades (Larsen and others, 2009a; Harvey and others, 2011) and in tracer experiments in the field (Regier and others, 2018), however the flume work was primarily aimed at understanding entrainment of suspended sediment and phosphorus and not deposition. Furthermore, although the tracer work indicated that sediment redistribution is operative, the results are qualitative. Sediment transport is thought to be an important driver of phosphorus transport because much of it is associated with sediment in the Everglades. Confirmation of the magnitude of transfer of sediment and phosphorus from sloughs to ridges is needed at larger spatial scales within ridge and slough ecosystems.
DPM is a 10-square-kilometer area of Everglades wetlands that have been isolated by levees for nearly sixty years. The recent completion of the S-152 gated structures on the L67-A levee had permitted the release of large quantities of water (hundreds of cubic feet per second) that spread across the DPM wetlands and raise velocities substantially, by about two to ten times within a kilometer of the culverts. Here we report on measurements of suspended sediment and total phosphorus in sloughs and ridges and implement a simple flow model to assess the importance of sediment redistribution.
Methods
Methods of data collection and analysis are described in the “Suspended Sediment Sampling” and “Water Quality and Physiochemical Monitoring” sections. Here, we describe the modeling methods used to assess transport and redistribution of suspended sediment.
Model estimation of sediment redistribution.—We used the One-Dimensional Transport with Inflow and Storage (OTIS) model (Runkel, 1998) to simulate the transport and reaction of suspended sediment and total phosphorus during flow releases in 2015. Our modeling approach was designed to distinguish the fate of suspended sediment and total phosphorus flowing through a slough and ridge landscape. The input and downstream movement of water and suspended constituents were simulated before and during the operation of the S-152 culverts, which deliver high flow into the DPM. The OTIS simulation accounts for flow through a main flow path (slough) that exchanges water with secondary flow paths moving through ridges. It also accounts for removal of constituents by settling to the bed, by getting captured on vegetation stems, or by reactive transformation.
The OTIS governing equation is:
where the left side of the equation represents the changing concentration, C, in the primary flow paths through sloughs and the right-hand side of the equation represents effects of several processes including advection, longitudinal dispersion, lateral mixing, hydrologic exchange with secondary flow paths through ridges, and removal of constituents from flowing water, either by settling, capture on vegetation stems, or reactive transformation. The associated governing equation for concentration in the secondary flow paths through ridges is: where the variables of the above equations are defined as:C
is the main flow zone (slough) constituent concentration [M/L3]
Q
is the volumetric flow rate [L3/T]
A
is the main flow zone (slough) cross-sectional area [L2]
AS
is the storage zone (ridge) cross-sectional area [L2]
D
is the longitudinal dispersion coefficient in main flow zone (slough) [L2/T]
qLin
is lateral inflow rate [L3/T-L]
CL
is the lateral inflow constituent concentration [M/L3]
α
is the storage zone water exchange coefficient [1/T]
CS
is the storage zone (ridge) constituent concentration [M/L3]
λ
is the main flow zone (slough) 1st-order constituent removal coefficient [1/T]
λs
is the storage zone (ridge) 1st-order constituent removal coefficient [1/T]
t
is time [T]
x
is distance [L].
The application of the OTIS model for simulating suspended sediment concentration (SSC) and total phosphorus (TP) transport through sloughs, including exchange with ridges and settling and particle capture on vegetations stems, can benefit by first simulating a conservative solute tracer. The conservative tracer helps constrain the transport time and rate of water exchange between the main flow path through a slough and secondary flow paths through ridges. For the conservative solute tracer at DPM we simulated total dissolved solids as detected by measuring specific conductivity (SC). Because the SC of surface water entering the DPM through the S-152 culverts differed from the SC in the DPM wetland, and because total dissolved solids are transported without reaction, the SC signal could be used to quantify conservative transport processes, specifically, advection and longitudinal dispersion of flow through sloughs, and rate of water exchange between the sloughs and secondary flow paths across ridges. With those parameters constrained it was easier to model total phosphorus (TP) which undergoes the same conservative transport processes but also is affected by reactive processes. Specifically, TP concentrations are also affected by settling of phosphorus associated sediment particles and capture of particles on vegetation stems.
Three OTIS models were solved, one for SC and the others for SSC and TP, to determine the “best-fit” estimates of the parameters. The flow data, specific conductivity, suspended sediment, and total phosphorus data used in the calculations are available in Choi and others (2016), Harvey and others (2022a) and the model inputs and outputs are detailed in Choi and Harvey (2023).
The following sections describe two steps to solve OTIS models to arrive at final answers. In the first step, an OTIS model for SC was solved to match the measured time series of SC in the slough which produced the conservative transport parameters for a slough flow path interacting with more slowly moving flow paths though ridges. In the second step, the conservative transport parameters from the SC simulation were used as fixed parameters in the reactive transport simulations, from which the “best-fit” reactive parameters for SSC and TP were determined for slough flow paths interacting with ridge flow paths. The two steps are explained in detail below:
Step 1. Estimating conservative transport of constituents in ridges and sloughs.—The OTIS modeling approach helps interpret measured concentrations by distinguishing the effects of transport and reaction processes. Time-series data from the 2015 flow releases were used to prepare model inputs including water velocities, water depths, and SC, SSC, and TP to optimize parameters describing transport and reaction in the main flow zone through sloughs and secondary flow paths through the more densely vegetated ridges.
Conservative transport simulations were undertaken by modeling transport between sites Z51_USGS and RS1D in 2015. In 2015, there were two flow releases, and we simulated specific conductivity from the second release because there was a better distinction between the SC of the flow-release water and background values in the DPM. To account for slough sinuosity, the measured linear distance of the 2014 and 2015 reaches was increased by multiplying by 1.3. Discharge (Q) was estimated for each simulation as the product of measured velocity and depth at the upstream end of the reach. Lateral inflow (qLin) was set to zero for each simulation. After the flow release had steadied, the differences in measured velocities and depths at the upstream and downstream endpoints were used to estimate cross-sectional area (A) for each reach. We then created input files using the estimated Q and A and the adjusted distance along to run OTIS models that provided optimal estimates of the dispersion coefficient (D), the exchange rate (α) and storage cross sectional area (As) as outputs. Optimization was accomplished using the Parameter Estimation Software Tool (PEST) (White and others, 2020) in tandem with OTIS to objectively fit the measured SC data.
Step 2. Estimating SSC and TP removal rate constants in ridges and sloughs.—Reactive transport simulations were undertaken with the purpose of quantifying and comparing SSC and TP removal in sloughs and ridges. The best-fit conservative transport parameters (Q, A, D, and As) that had been determined from the conservative transport simulation (see section “Dissolved oxygen and temperature modeling”) were used as inputs to the reactive transport simulations. The reactive transport simulations were aimed at estimating SSC and TP mass removal rate constants (λ and λs ) for main flow paths (slough) and secondary flow paths (ridges), respectively. Like the conservative transport simulation, the reactive transport simulations were optimized using the PEST code. As a result, SSC and TP removal rate constants were estimated that achieved the “best fit” to measured SSC and TP concentrations.
Partitioning mass removal between slough and ridge required four simulations each for SSC and TP: (1) a simulation to quantify transport through the reach without removal (simulation A; described in section “Dissolved oxygen and temperature modeling”); (2) a simulation to quantify removal rate constants for both slough and ridge (simulation B); (3) a simulation to quantify the removal rate constant if removal only occurred in the slough (simulation C); and (4) a simulation to quantify the removal rate constant if removal only occurred in the ridge (simulation D). In summary, the four simulations were:
-
1) Simulation A: Conservative transport simulation in the reach with
and both set to zero (Step 1) -
2) Simulation B: Reactive transport simulation in the reach with
and both optimized to determine best fit values (Step 2) -
3) Simulation C: Reactive transport simulation in the reach with
optimized to determine best fit value and set to zero (Step 2) -
4) Simulation D: Reactive transport simulation in the reach with
set to zero, and optimized to determine best fit value (Step 2)
Mass removal fluxes of SSC and TP for slough and ridge were computed from differences between the conservative simulation in step 1 and the appropriate reactive simulations in step 2. First, the mass fluxes through the reaches were computed for each simulation from the simulated concentration curves at the downstream end of the reach multiplied by the average discharge for that period:
Mass fluxes for simulations C and D can be subtracted from simulation A to estimate removal in slough and ridge, respectively. Those estimates are biased slightly high because of assuming independence of removal in slough and ridge. However, dividing those quantities by simulation B accurately computes the fraction of SSC and TP mass removal in slough and ridge, respectively, during the period of interest. For example, (Mass flux_Sim-A – Mass flux_sim-C)/(Mass flux_Sim-A – Mass flux_sim-B) computes the fraction of mass removal that occurred in the slough. Likewise, (Mass_Sim-A – Mass_sim-D)/(Mass_Sim-A – Mass_sim-B) computes the fraction of mass removal that occurred in the ridge. Multiplying the fractions of removal by (Mass_Sim-A – Mass_sim-B) adjusts and finalizes the calculation of mass removal occurring in slough and ridge respectively during the period of interest.
Results and Discussion
We were interested in comparing mass removal that occurred during the initial pulse of a high-flow release with mass removal that occurred later during steady high-flow. The initial pulse lasted approximately 6 hours when SSC or TP concentration spiked, but concentrations tended to settle down during the steady flow period that followed, which lasted as long as the culverts were open, often 30 days or more. To accomplish that, we made mass removal computations for nine distinct periods immediately before and for several months after the flow releases in 2014 and 2015. The periods were selected to distinguish the different flow conditions that were well described by our measurements of SSC and TP concentrations.
The modeling characterized sediment and total phosphorus areal deposition in sloughs and in ridges caused by the 2015 flow release from the S-152 culverts. The areal deposition numbers characterize the average deposition in both sloughs and ridges between sites Z51-USGS and RS1-D. The instantaneous rate of areal deposition is compared for the initial pulse of high flow and a longer period of steady high flow. We also compared a 3-month set of conditions, where it was assumed that only 6-hr pulsed flows occurred every 14 days and allowed time for regrowth of transportable biomass (for example, Harvey and others, 2011), which was compared with deposition associated with a 3-month steady flow (tables 16 and 17).
Table 16.
Areal deposition of suspended sediment transported by 2015 flow release from the S-152 culvert.[Small numbers are expressed in exponential notation. g Sed/s/m2, gram sediment per second per square meter; g Sed/yr/m2, gram sediment per year per square meter; g Sed/m2, gram sediment per square meter; hr, hour]
Table 17.
Areal deposition of total phosphorus transported by 2015 flow releases from the S-152 culvert.[Small numbers are expressed in exponential notation. g TP/s/m2, gram total phosphorus per second per square meter; g TP/yr/m2, gram total phosphorus per year per square meter; g TP/m2, gram total phosphorus per square meter; hr, hour]
Consistent with the sediment redistribution hypothesis, rates of sediment deposition on ridges were 3 to 6 times greater on ridges compared to sloughs during high-flow releases at DPM. Also, the short-term pulsed rates of deposition were higher than the longer-term steady-state rates in the sloughs, especially for the first of the single-day pulses (the second pulse was conducted a day later). The generally higher pulsed rates of sediment deposition reflect the time needed to build up the standing stock of the easily entrainable fine particulates associated with periphyton in the water column (Harvey and others, 2011), which comprises the major source of suspended sediment that settles to the bed (Noe and others, 2010).
Phosphorus deposition followed similar patterns to sediment deposition, being higher on ridges compared to sloughs. Ridge-slough differences during pulses were pronounced, with ridge deposition rates typically a factor of 50 greater than sloughs. The ridge-slough difference during the longer-term period of steady-state high flow was much less, by just a factor of 2, which was more similar to ridge-slough differences in sediment deposition. The steady-state rates of phosphorus deposition were more moderate, falling between the very low values in sloughs during pulses and the very high values for ridges during pulses. These findings suggest a higher efficiency of ridge capture of phosphorus during pulsed flows, however those pulsed-flow behaviors are unsustainable as reflected in the lower deposition rates of the second pulse compared to the first, as well as in the much lower steady-state rates. Like sediment deposition, the lower rates of phosphorus deposition over longer periods are presumed to be the result of the time needed for replenishing the standing stock of easily entrainable phosphorus in the water column (Noe and Childers, 2007).
Most of the rates of phosphorus deposition during high flows in these DPM studies are higher than published values for oligotrophic sloughs by approximately 10 to 20 times for the longer period of steady high flow (Noe and Childers, 2007), and more for pulsed flow, notably on ridges (however, slough deposition rates during pulsed high flow were comparable to the background low-flow values of Noe and Childers [2007]). Reasons for the high- and low-flow difference include the much higher loading of phosphorus that occurs with high flow, typically an order of magnitude higher for steady high flow, assuming that concentration changes little and the increase in transported phosphorus load is the same as the flow increase caused by the culverts. An order of magnitude higher phosphorus loading during steady-state high flows therefore approximately explains the order of magnitude higher phosphorus deposition rates for steady high flow (table 17). The spike in concentrations sometimes observed during pulsed flow indicates even higher load increases for a brief period of 6 hours or so which resulted in deposition rates on ridges as much as 60 times than literature values for low-flow conditions in sloughs. Those high values are for the edge of ridges and not central ridges, which agrees with modeling by Larsen and others (2009b) and Larsen and Harvey (2011) indicating that sediment redistribution is the result of entrainment in sloughs and deposition primarily on ridge edges. Another reason for higher phosphorus deposition values compared to low-flow literature values is the radial flow pattern that causes entrainment upstream and deposition downstream because of the logarithmic decrease in velocity as a function of distance. Our selected test reach encompassed conditions of entrainment in both slough and ridge at the upstream end and conditions near the threshold for entrainment in sloughs but deposition in sloughs at the downstream end. Thus, there are several reasons why our observations of higher phosphorus deposition rates are reasonable for DPM high-flow conditions with radial spreading compared to oligotrophic sloughs under low-flow conditions.
Wetland Sheet Flow Continuity through Levee Gaps and Role of Canals
Introduction
A key aspect of Everglades restoration is the removal of levees to promote sheet flow through wetlands to better mimic the pre-drainage system. The DPM reintroduces sheet flow to an area of the central Everglades that has been isolated for nearly sixty years. An important objective of DPM is to test how levee gap design and the degree of backfill (no backfill, partially backfilled, and completely backfilled) influences sheet flow and conveyance of suspended sediment, total phosphorus, and total particulate phosphorus to downstream areas. In 2012, a 914-m section of the L67-C levee was bulldozed to grade with the surrounding wetlands, and the excess material was used to construct contrasting levels of backfill in three side-by-side treatments within the exposed L67-C canal. We found that backfill treatments differed in how they conveyed constituents, that is whether the different treatments passively conveyed constituents or whether treatments acted as a sink by retaining constituents as a result of deposition or biological uptake, or whether treatments acted as a source of constituents by deposition by entrainment from the canal bottom as water passed through them. We found that the treatment with no backfill (herein referred to as the no-backfill treatment) passively conveyed water and constituents, the partially backfilled treatment (herein, partial-backfill treatment) exhibited tendencies for both sources and sinks of sediment and phosphorus, and the completely backfilled treatment (herein, complete-backfill treatment) tended to retain total phosphorus and total particulate phosphorus. Low-flow and high-flow measurements at the L67-C gap also revealed an unintended effect: the unblocked portions of the L67-C canal adjacent to the gap became a major source of water flowing through the levee gap, which has the potential to transport undesirable levels of phosphorus to areas farther downstream.
Site Information and Methods
The DPM is in a small area in of the central Everglades where the wetlands have been isolated between the L67-A and L67-C levees for nearly sixty years, referred to as the pocket. The only sheet flow that occurred through the wetlands was caused by groundwater exchanges between the two levees and by local precipitation. The recent completion of the 914-m wide L67-C levee gap in August of 2012 permitted sheet flow from DPM to begin flowing directly through the gap and into WCA 3B. Flow through the gap was minimal at first because there was no new source of water to the DPM to sustain flow through the gap. Soon after, in November 2013, the S-152 gated structures on the L67-A levee were completed, which permitted the release of substantial quantities of water from WCA 3A into the DPM, spreading across the wetlands, and passing through the L67-C levee gap into WCA 3B.
The DPM high-flow experiments are being conducted in an area of 3×3 km that lies between two parallel levee-canal systems. At the upstream end of the DPM, a bank of 10 gated culverts was completed in 2013 to convey water from WCA 3A through the L-67A levee at rates up to 650 ft3/s in a radial pattern that spreads across the pocket and eventually passes through an approximately 914-meter gap in the L-67C levee that was bulldozed to the wetland grade to permit flow from the pocket into WCA 3B. The L-67C levee was flattened in 2012, at which time the canal portion directly adjacent to the levee degrade was backfilled to varying degrees in three 300-m treatment areas built to test the conveyance of water, sediment, and phosphorus across the 900-m levee gap into WCA 3B.
The three canal backfill treatments include an undisturbed no-backfill section (CB1), a partial-backfill section that was backfilled with rock to a depth of 30 cm on top of the pre-existing canal bottom, and a complete backfill section (CB3) that had been backfilled with rock to a grade resembling a 1- to 2-m deep slough in the surrounding wetlands (fig. 13). The height of the degraded levee surface is uneven, and it has two deep openings, one at the south end of CB3 and the other at the north end of CB1, where water flowing into WCA 3B is concentrated. There is also a relatively long portion of CB3 where the levee was not flattened sufficiently so water rarely overflows.
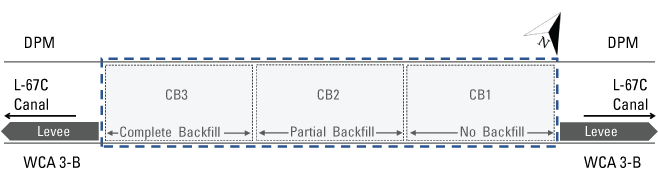
Diagram showing the canal backfilling experimental site at the south end of the Decompartmentalization Physical Model (DPM) experimental site where water and constituents are transported through a constructed 900-meter gap in the L-67C levee in the central Everglades, Florida. WCA, Water Conservation Area.
Here we report on a mass balance analysis of water and constituents entering and leaving the backfill treatments that was conducted during low-flow conditions in November 2015 and during high-flow conditions in November 2016. The purpose was to quantify the source of water and constituents entering the levee gap and to assess whether materials were passively conveyed through the gap, often referred to as “conservatively transported,” whether constituents settle or are removed by biological reactions (referred to as a “sink”), or whether constituents are entrained or released by biological or geochemical reactions (referred to as a “source”) during transport through the gap. Mass balances were computed in three more years (2019, 2020, and 2022); however, only water balances could be computed because suspended sediment and phosphorus concentrations were not measured in those years.
Field Measurements.—All field measurement locations are shown either in figure 1, which provides an overview, or in figure 14, which is a detailed inset of the L67-C canal gap area. Low-flow measurements were made on November 14, 2015, when the S-152 structure gates were closed and not releasing water into the DPM area. Inflows from the unblocked extensions of the L67-C canal just outside of the gap (CCN and CCS) were measured using two continuously recording SonTek Argonaut XRs. Sheet flow measurements during the low-flow campaign were only made at the three locations entering the levee gap (UB1, UB2, and UB3 sites) and three locations exiting (DB1, DB2, DB3 sites) the gap. The measurements were made using six SonTek ADVs, which were positioned at three fixed stations on the upstream and downstream sides of the levee gap.
High-flow measurements were made after flow had steadied following the opening of the S-152 structure gates in the fall of 2016, 2019, 2020, and 2022. Rather than just six measurements of sheet flow, many more measurements of sheet flow were made during the high-flow campaigns. During each flow release, approximately 87 measurements of sheet flow were made, which required roving teams to use hand-held SonTek Flowtrackers.
In 2016, the high-flow measurements were made on several days over two weeks that later were adjusted, as explained below, so that all flows were estimated on a single day, November 29, 2016. Flow adjustments were necessary to meet the assumption of the steady-state (non-changing) flow analysis. Sheet flow measurements entering and leaving the canal were made on November 26, 2016, and suspended sediment and phosphorus concentration measurements were made by SFWMD on November 14, 2016, and November 15, 2016, within the canal and downstream of the canal. Canal velocity cross sections were measured on November 17, 2016, using a roving SonTek acoustic Doppler current profiler (ADCP). However, because of short-term blocking of the S-152 culverts on that day, the flows were lower than the days when the other mass balance measurements were being made. The roving ADCP flows on November 17, 2016, were corrected to represent conditions on November 29, 2016, by using a relationship between S-152 culvert structure discharge and continuous measurements from the fixed ADCP station at cross-section CCN (fig. 14).
In 2019, the high-flow measurements were made on several days during a one-week period that were later adjusted, as explained below, so that all flows were estimated on a single day. Again, this was necessary to meet the assumption of the steady-state (non-changing) flow analysis. Sheet flow measurements entering and leaving the canal were made on October 15–16, 2019, discretely by SonTek FlowTracker. During October 8–10, 2019, canal velocity cross sections were measured discretely, using a roving SonTek ADCP and continuously using a SonTek Argonaut XR at the northern gap (CCN) and a SonTek IQ plus at the two downstream transects (CB2_S to CB3_N and CCS) (fig. 14). We accounted for the temporal changes in flow during the measurement period at the CCN location by replacing the water flux measured on October 8, 2019, with a water flux on October 16, 2019, estimated using a linear relationship between the flux at CCN and the discharge at the S-152 culvert. Furthermore, we used continuous discharge measurements made by a SonTek IQ plus on October 15–16, 2019, (the average of two days), during which times the S-152 culvert discharge was steady, in place of the discrete measurement on October 10, 2019, by the ADCP to better account for the temporal change at two other canal cross sections—CB2_S to CB3_N and CCS (fig. 14).
In 2020, the high-flow measurements were once again made on several days over a one-week period, with some flows being adjusted as explained below to meet the assumptions of the steady-state (non-changing) flow analysis. Sheet flow measurements around the canal were made on September 8–9, 2020, discretely by a SonTek FlowTracker. During September 1–3, 2020, canal velocity cross sections were measured discretely using a roving SonTek ADCP and measured continuously using a SonTek Argonaut XR at the northern gap (CCN) and Sontek IQ plus at the two downstream transects (CB2_S to CB3_N and CCS). We accounted for the temporal changes in flow during the measurement period at the CCN location by replacing the water flux measured on September 2, 2020, with a water flux on September 8, 2020, estimated using a linear relationship between the flux at CCN and the discharge at the S-152 culvert.
In 2022, the high-flow measurements were once again made on several days over a one-week period. Sheet flow measurements around the canal were made on January 25, 2022, and January 26, 2022, discretely by Sontek FlowTracker. Canal velocity cross sections were measured both discretely on January 24, 2022, to January 27, 2022, using a roving SONTEK ADCP. The temporal change in discharge at the S-152 culvert between the periods of sheet flow and cross section measurements was very small, therefore we did not apply any correction on the cross-section measurements.
Mass Balance Approach.—Mass balances were computed for a levee gap control volume, enclosing the 914-m opening in the levee and canal area between DPM and WCA 3B. Mass-balance computations assume steady-state conditions, that is, that no changes in storage occur in the control volume for the time period represented by the input data. Computations were made for water fluxes, suspended sediment fluxes, total phosphorus fluxes, and total particulate phosphorus fluxes entering and leaving the levee gap and backfill treatment control volumes. To compute flows entering and leaving, the local measurements of sheet flow were resolved in the direction normal to the gap and upscaled to account for the flux across an appropriate portion of the control volumes outer boundary. Likewise, canal fluxes were resolved in a direction parallel to the gap and upscaled to account for the flux across an appropriate endpoint of the canal gap.
The much higher number of measurements made during the high-flow campaigns allowed mass balances to be computed for each of the three backfill treatments, CB1 (no backfill), CB2 (partial backfill), and CB3 (complete backfill) (fig. 13). Furthermore, because of several localized areas of channelized flow across the degraded levee, it was beneficial to split the backfill treatments in half, which created a north and south section for each of the three backfill treatment, that is, representing six individual sub-sections of the entire levee gap (fig. 14).
To compute the mass balances for high-flow releases, the 87 sheet-flow measurements were therefore resolved into six fluxes entering a sub-section from the upstream (DPM) side and six fluxes leaving a sub-section on the downstream side to enter WCA 3B. All of the flux measurements were available to compute the balances except for two canal flux measurements, that is, the flux between CB1-south and CB2-north and the flux between CB2-south from CB3-north (fig. 14). Estimates of canal fluxes at those two locations were computed by difference, that is, the flux was computed that was needed to balance water mass exactly in the northern sub-section of each backfill treatment. That approach left a small water balance residual in the southern sub-sections of each backfill treatment which was deemed to be acceptable. Figure 14 names the fluxes that were used as inputs for the mass balance calculations.
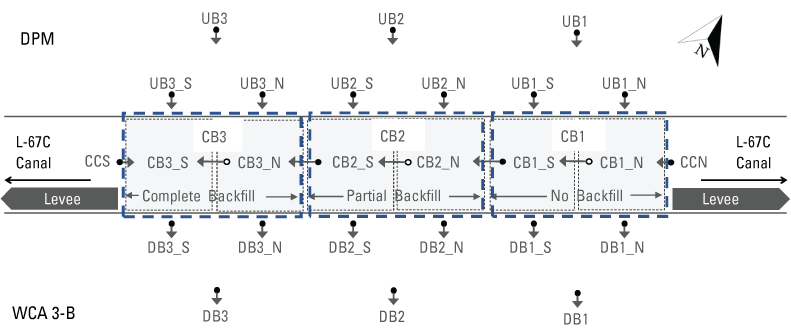
Schematic diagram showing mass-balance computations for the L-67C levee gap in the Everglades, Florida. Mass balances were computed both for low-flow conditions (November 14, 2015) when the S-152 gated structures were closed and for high-flow conditions (November 29, 2016) when the S-152 structure gates were fully open and releasing surface water into the DPM pocket. See text for explanation of specific measurements and how the levee-gap mass balance as a whole was computed, and how mass balances were computed for the three backfill treatments, CB1 (no backfill), CB2 (partial backfill), and CB3 (complete backfill). WCA, Water Conservation Area.
Mass Balance Calculations.—The steady-state mass balance equation is:
where inflows include sheet flow from DPM wetlands directly upstream of the levee gap plus lateral canal flow from the unblocked extensions of the L67-C canal, and outflows are sheet flow to downstream wetlands in WCA 3B.The variableMeasurement uncertainty was estimated separately for water, suspended sediment, total phosphorus, and total particulate phosphorus fluxes by assuming a priori a given value of uncertainty for water fluxes and a given uncertainty for constituent fluxes. We followed the general guidance of Winter (1981) and our experience in measuring Everglades fluxes to assign levels of uncertainty for the DPM levee gap study as 15 percent uncertainty for measurements of water fluxes and as 30 percent uncertainty for measurements of constituent fluxes.
Individual measurement errors propagate and are accumulated in the calculated mass
balance uncertainty (
Estimating sources or sinks of constituents within the levee gap.—If a calculated net flux for water or a constituent computed using equation 35 was larger than the associated computation of uncertainty using equation 36, then it was interpreted as evidence that the levee gap is a source (if the net flux is negative) or a sink (if the net flux is positive) for water or that constituent. Such results provide indicators of source-sink dynamics although they cannot identify what processes are responsible. Interpretations from mass balance analysis can be strengthened using additional measurements that independently support conclusions about the processes involved, for example, direct measurements of sediment settling or entrainment from the canal bed, and so forth.
To isolate individual effects of the canal-backfill treatment, the net-flux calculations were computed for six canal-backfill treatments (CB1-N, CB1-S, CB2-N, CB2-S, CB3-N, CB3-S) that subdivided the backfill treatment areas into north and south sections (fig. 15).
Results and Discussion
During the low-flow measurement period in November 2015, when the S-152 structure gates were closed, we observed 4.8 m3/s flow through the levee gap that had collected in the pocket because of groundwater seepage beneath the L-67A levee as well as local precipitation. At low flow, two-thirds (67 percent) of the water that entered the levee gap came from the unblocked L67-C canal and one third (33 percent) entered as wetland sheet flow from directly upstream (table 17).
During the high-flow measurement periods the total flow of water through the levee gap increased because of the added discharge from the S-152 gated culverts (table 18). Compared to the low flow discharge of 4.8 m3/s into the gap, total discharge through the gap increased by 25 percent to 6.1 m3/s in November 2016, again by 25 percent to 6.0 m3/s in October 2019, and by 102 percent to 9.6 m3/s in September 2020 (table 18).
Table 18.
Water fluxes entering the levee gap accounting for all pathways including wetland sheet flow and flow from the unblocked extensions of the L67-C canal to the north and south of the levee gap for four different years in the Everglades, Florida.[Units are in cubic meters per second.]
Confounded effects of water sources, uneven flows, and backfill treatments.—The unblocked L-67-C canal accounted for slightly more than two thirds of water fluxes entering levee gap during 2015 low-flow and during the 2016 and 2019 high-flow measurement periods (table 19). During the 2020 high-flow period, when S-152 culvert discharge was higher, the unblocked L67-C canal accounted for a slightly smaller percentage of total flow, 62 percent (table 19).
Regardless of flow condition, the flow from the unblocked L67-C dominated water flow into WCA 3B (ranging from 61 percent to 70 percent) compared with smaller sources from wetland sheet flow (ranging from 30 percent to 39 percent) (table 19). Therefore, the unblocked L67-C canal interferes with the desired enhancement of sheet flow to improve direct wetland-to-wetland connectivity between the Water Conservation Areas in the central Everglades (Larsen and others, 2017b).
Table 19.
The percent of the water, sediment, total phosphorus, and total particulate phosphorus entering the L67-C levee gap from the unblocked L67-C canal rather than sheet flow for four different years in the Everglades, Florida.[Values are in percent. SSC, suspended sediment concentration; TP, total phosphorus concentration; TPP, particulate phosphorus concentration; —, not available]
The source of DPM constituents entering the 900-m levee gap was dominated by delivery from the adjacent unblocked L67-C canal rather than from sheet flow (table 19). Approximately two-thirds of the water, suspended sediment, and total phosphorus with more than half of the total particulate phosphorus entering the levee gap from the L67-C canal. There was little change from contributions during low-flow background conditions versus during high-flow releases, with 67 percent versus 69 percent contributed for water, 67 percent versus 73 percent contributed for suspended sediment, and 63 percent versus 65 percent contributed for TP, respectively, during low flow versus high flow in terms of percent contributions from the canal. Accordingly, wetland sheet flow contributed less than half of the water and constituents entering the levee gap. For example, in the 2016 flow release, the contributions from the canal accounted for 31 percent, 27 percent, 35 percent, and 41 percent of the water, sediment, total phosphorus, and total particulate phosphorus that entered the levee gap (table 19).
Furthermore, the canal water flow from the unblocked L67-C canal enters the gap unevenly from the north end and the south end, with 96 percent entering from the north side of the gap (table 19). The dominance of the north side extension of the L67-C canal is explained by the easterly trending direction of DPM high-flow releases which, although spreading radially from the S-152 gated structures, trend eastward with a center of mass that reached the L67-C canal at a location several kilometers north of the levee gap. Therefore, sheet flow entering the levee gap might be somewhat higher than measured at DPM because of an unplanned consequence of the DPM design.
Drawing conclusions about canal backfill functions must confront challenges involving the uneven source contributions and flow distribution in the levee gap. A first challenge was that the unblocked L67-C canal, rather than wetland sheet flow, is the major supplier of water and constituents (approximately 94 percent) in the no-backfill treatment, the most northerly section of the levee gap closest to unblocked canal to the northeast that receives most of the flow. The other backfill sections received less canal flow, more than half in the partial-backfill treatment, and approximately a quarter of the flow entering the complete-backfill treatment (table 20).
Table 20.
The percentage of constituents entering the levee gap and each canal backfill treatment area through the unblocked L67-C canal during the high-flow measurement period for three different years in the Everglades, Florida.[L67-C levee gap individual treatments: “no backfill” treatment, “partial backfill” treatment, and “complete backfill” treatment for high flow at each site (CB1, CB2, CB3). Values are in percent. SSC, suspended sediment concentration; TP, total phosphorus concentration; TPP, particulate phosphorus concentration; —, not available]
A second challenge was that the magnitude of water and constituent fluxes passing through the levee gap into WCA 3B were much greater in the northernmost no-backfill treatment compared with the other treatments (table 21). Approximately sixty percent of the water and constituents entering WCA 3B passes through the no-backfill treatment on the northern side of the gap, one quarter of the water and constituents passes through the complete-backfill treatment on the southern side, and the smallest amount of water and constituents (less than one-fifth) passes through the partial-backfill treatment, which is located in the middle of the levee gap (table 21). As a result, the loading of constituents into WCA 3B was the highest by far since it was directly downstream of the no-backfill treatment rather than being evenly spread out through the entire 900-m levee gap.
Table 21.
The percent contribution of three backfill treatments to total fluxes of water, sediment, phosphorus, and particulate phosphorus fluxes through the L67-C levee gap into Water Conservation Area 3B during the high-flow measurement period in 2016 in the Everglades, Florida.[Values are given in percent. SSC, suspended sediment concentration; TP, total phosphorus concentration; TPP, particulate phosphorus concentration]
The ramifications of uneven contributions of canal water in the three treatments are obscuring the effects of the different backfill functions. It would be easier to uniquely distinguish the effects of contrasting levels of canal backfill if treatments received similar mixes of wetland sheet flow and canal water, and if the total flow through each backfill treatment was closer to equal.
Evidence for canal backfill treatment sources and sinks of constituents in the levee gap.—We found that backfill treatments differed in the degree to which they passively conveyed constituents or whether the levee gap retained or was a source of constituents. Constituents might be retained because of settling of sediments or biological uptake of phosphorus. Or there might be a source of constituents because of the entrainment of sediments from the bed or a biogeochemical release of phosphorus from sediments as water passed through the gap (table 22).
Table 22.
The percent of water, sediment, phosphorus, and particulate phosphorus entering that was retained by the north and south portions of the three backfill treatments in the Everglades, Florida.[The three backfill treatments are as follows: CB1, no-backfill treatment; CB2, partial-backfill treatment, and CB3, complete-backfill treatment. All values and associated uncertainties (±) given in percent. A positive value greater than uncertainty suggests that retention occurred in that portion of the canal-levee gap. A negative value greater (in terms of absolute values) than associated uncertainty suggests a source occurred in that portion of the canal-levee gap. SSC, suspended sediment concentration; TP, total phosphorus concentration; TPP, particulate phosphorus concentration; ±, plus or minus]
The no-backfill treatment area, which had a very high flux as well as a high fraction of L67-C water passing through it, passively transported the materials entering it in both its northern and southern sections, causing significant loading of sediment, phosphorus, and particulate phosphorus into WCA 3B. (table 22, fig. 15).
The partial-backfill treatment area was a transition area where, in its northern half, suspended sediment was retained, probably by deposition, and particulate phosphorus was released from the canal bottom. In the southern section of the partial-backfill treatment, total and particulate phosphorus were retained. Sediment and phosphorus dynamics in the partial-backfill treatment could be the result of a slowdown of water as southerly flow from the unblocked L67-C canal crosses the ramp of backfill material in the partial-backfill treatment and causes deposition of sediment in its northern section and deposition of phosphorus in its southern section (table 22, fig. 15). That result also could reflect model errors that indicated a retention of water in the partial-backfill treatment (table 22), an unexpected result that is unlikely to have occurred.
Because of its southern position in the gap, the complete-backfill treatment was largely out of reach of inflows from the unblocked extension on the L67-C canal. Therefore, the complete-backfill treatment had lesser influence of canal input, receiving a quarter of its inflow from the unblocked extensions of the L67-C canal and three quarters of its inflow from wetland sheet flow (table 20). In its northern half, the complete-backfill treatment acted as a sink for phosphorus and particulate phosphorus, which is consistent with the observed presence of submerged aquatic vegetation in the complete-backfill treatment, which adds abundant surface area for microbial and algal communities with demand for phosphorus (fig. 15).
Thus, the three backfill treatments differed in how they conveyed constituents, however the observed effects are confounded to a degree with the uneven flow of water and constituents and the non-uniform canal versus sheet flow contributions. Nonetheless, it appears that the compete-backfill treatment, which best represents a backfilled canal receiving a dominant source of water from wetland sheet flow, did exhibit desirable retention of TP and TPP.
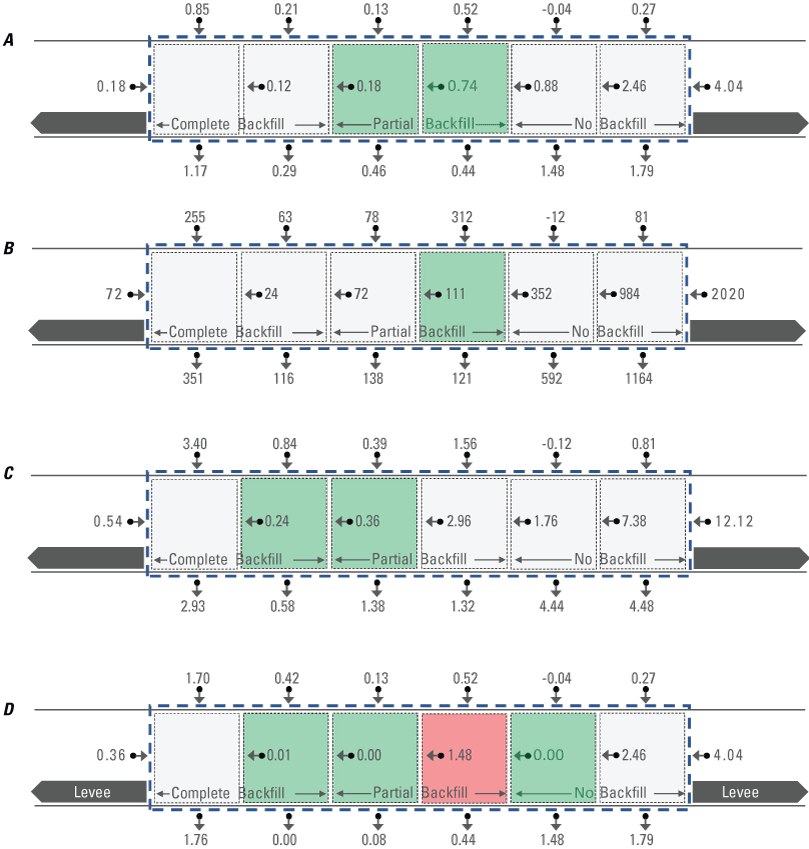
Levee gap mass balance during the 2016 high-flow release showing fluxes of water (A), suspended sediment (B), total phosphorus (C), and total particulate phosphorus (D). A grey cell indicates that the material is passively transported from DPM through the levee gap into WCA 3B, a green cell indicates that retention occurs in that portion of the levee gap, and a red cell indicates that the levee gap acts is a source for that material. Water fluxes are in units of cubic meters per second (m3/s), and suspended sediment, total phosphorus, and total particulate phosphorus are in units of milligrams per second (mg/s).
In conclusion, The DPM levee gap mass balance provided important insights and supported the following conclusions in spite of experimental design difficulties: 1) the unblocked extensions of L67-C canal significantly diminished wetland to wetland connectivity by sheet flow, which is one of the major goals of removing Everglades levees, and 2) the compete-backfill treatment dominantly received sheet flow from directly upstream and exhibited the desirable outcome of retaining TP and TPP. Thus, mass balance analysis suggests that backfilling canals can favorably influence wetland to wetland connectivity and can also perform the ecosystem function of retaining phosphorus which slows its movement to downstream areas. Our mass balance results can be combined with independently collected information such as sediment and phosphorus settling measurements and measurements of canal bed and wetland phosphorus content to provide further insights and strengthen interpretations.
Metabolism Indicators of Wetland Ecological Response to High Flow
Introduction
Many experimental re-introductions of high flow to low-gradient floodplains have been short term and small-scale experiments in flumes (for example, Larsen and others, 2009a; Harvey and others 2011). Recently, however, a kilometer-scale experimental facility was established to increase floodplain flows in an area of the central Everglades for several months each year. The DPM included extensive measurements to characterize short- and -longer term hydraulic, sedimentary, and ecological outcomes.
Here, we report on ecosystem outcomes of restored high flows at DPM using aquatic metabolism as an integrated measure of ecosystem response to high flows. Metabolism quantifies the balance between production by photosynthesizing organisms and consumption of organic carbon by respiring plants, animals, algae, and bacteria and through decomposition pathways. Metabolism can characterize ecological outcomes such as changing capacities for processing carbon and nutrients that may also affect geomorphic outcomes in an organic floodplain landscape. We also assessed metabolism at multiple ridge and slough sites in the DPM experimental area located in the central Everglades.
Analysis Approach
We used a Bayesian state-space model in the open-source statistical program R, streamMetabolizer, version 0.12.0, (Appling and others, 2018a) to inversely estimate aquatic metabolism in the Everglades. The model inversely estimates the parameters of a one-station oxygen mass balance equation where the change in oxygen concentration is the sum of three volumetric process rates affecting dissolved oxygen concentration in the water column:
where d[O2]/dt is the rate of change in O2 in milligrams of O2 per liter per day (mg O2/L/d) with time, t; Pt (in g O2/m3/d) is the rate of productivity that is modeled as mean daily gross primary productivity (GPP, in g O2/m2/d) using functional relationships with mean water depth (in meters), and light expressed in units of photosynthetic photon flux density (PPFD, in micromoles of photons per square meter per day [μmol photons/m2/d]); Rt is the rate of respiration that is modeled to estimate mean daily ecosystem respiration (ER, in g O2/m2/d) using a functional relationship with mean water depth (in meters); Dt (in g O2/m3/d) is the rate of O2 gas exchange with the atmosphere that is modeled to estimate mean daily gas exchange as the product of the temperature dependent gas exchange rate constant for oxygen,We set the specifications of streamMetabolizer to incorporate both observation and process error. Observation error results from errors in measurement associated with instruments or the measurement techniques; process error occurs when some physical or chemical process is not included (for example advection and dispersion of dissolved oxygen, DO) or is mis-specified (for example, assuming ER is constant within a day, and so forth) (Holtgrieve and others, 2010; Appling and others, 2018a). According to Appling and others (2018a), the inclusion of process error in the model removes a main source of autocorrelation of DO residuals that arises from the persistence of process effects (for example clouds that dampen GPP or afternoon wind that increases gas exchange).
Bayesian hierarchical partial pooling was used to constrain the across day variability of K600 for days with similar discharge (Appling and others 2018a) by establishing an across-day piecewise linear relationship between ln(K600) and ln(Q). Default values of the priors were used for partial pooling because results were not sensitive to a change in these initial values. Each low and high-flow period at a site was modeled separately because of the large differences in flow conditions.
State-space metabolism models can in some cases identify erroneous solutions with
high covariance of ER and K600 when GPP is low and K600 is high (Payn and others, 2017; Appling and others, 2018a; Appling and others, 2018b). Generally, we did not have those issues with the Everglades sites; however, to
ensure good performance we monitored for when the coefficient of determination, R2, of daily ER estimates with daily K600 estimates exceeded 0.5 for the modeled period; if so those models were re-run with
a smaller
In total, thirteen sites were modeled to produce metabolism estimates for 5,248 site-days of which 93 percent (4,876 site-days) passed quality assurance filtering. Ten of the 13 sites were used to compare annual and seasonal low-flow trends in sloughs and ridges after removing days affected by experimental high flow releases. That data set comprised 2,597 site-days from sloughs that were compared with 1,424 site-days from ridges. Before comparing slough and ridge sites, each site with multi-year data was summarized by averaging daily data from across years using a Julian calendar. High flow metabolism responses were evaluated at two key sites, a slough site (RS1D-S) and a ridge site (RS1-R). Sensor data are available in Harvey and others (2022a) and model inputs and outputs are detailed in Harvey and others (2022b).
Dual Gas-Tracer Experiment
Gas exchange between the water column and atmosphere has broad importance to ecosystem processes (Ho and others, 2018) as well as affecting the estimation of metabolism. To verify gas exchange modeling using streamMetabolizer, we conducted field-tracer measurements using dual-gas tracers. Experiments were conducted on two days, one representing low-flow conditions and the other representing high-flow conditions. For the dual gas-tracer method, the water column is saturated with two gases of very different solubilities at an injection location and dissolved concentrations are measured at several downstream locations. For this experiment, we used helium-3 (3He) and sulfur hexafluoride (SF6). Gas-exchange velocities in surface waters were determined using measured concentrations of the two gaseous tracers using the method of Wanninkhof and others (1993).
Dual gas-tracer experiments were performed on November 15, 2017, during low-flow conditions and on January 30, 2018, during high-flow conditions (S-152 structure discharge was 250 ft3/s). We first determined the transport path and travel time of water to determine the sampling locations and times for the injected tracer gases. During low-flow conditions, 100 grams of a 20-percent solution Rhodamine was mixed with 20 liters of Everglades water and released from site RS1U to determine the flow path and travel times to two downstream sampling sites. During high-flow conditions, 250 grams of a 20-percent solution Rhodamine was mixed with 20 liters of Everglades water and released from site RS1U to determine flow path and arrival times at three downstream sampling sites. The arrival and travel times of the Rhodamine solution were estimated by measuring fluorescence with a Scufa Submersible Fluorometer at each site. For the low-flow dual gas-tracer experiment, the peak arrival time at the first site (34 m) was measured to be 87 minutes after injection, and 167 minutes at the second site (58 m). For the high-flow experiment, the peak arrival time at the first site (34 m) was measured to be 12 minutes after injection, at the second site (155 m) it was 63 minutes, and at the third site (278 m) it was 215 minutes.
To begin the tracer experiments, 3He was injected at a constant rate of total 500 milliliters per minute (mL/min) using six diffusion stones, and SF6 was injected at a constant rate of total 60 mL/min using two diffusion stones at site RS1U. The diffusion stones were distributed vertically to avoid local variation and to achieve homogeneous gas concentrations through the water column.
Five samples were collected for each gas at the tracer gas injection site and each sampling site during the low-flow experiment, two samples were collected for each gas at the tracer injection site, and three samples were collected at each sampling site during the high-flow experiment. The sample collection methods were as follows: for water samples of 3He, the glass septum bottles were placed underwater while a 140-milliliter (mL) syringe was drawn through the water column. The contents of the syringe were then discharged into the bottle, and a rubber stopper with a needle pushed through was placed on the bottle. Performing this process underwater ensured that no gas in the air entered the sample bottle and that there was no headspace in the bottle. For water samples of SF6, a 140-mL syringe was drawn through the water column. A needle was affixed to the syringe, and the syringe and needle combination was pushed through the rubber stopper of a nitrogen-purged glass septum bottle. The contents of the syringe were discharged into the bottle. Samples were shipped on ice overnight to the USGS Reston Groundwater Dating laboratory (Reston, Virginia) where gas concentrations were analyzed.
Two-Station Metabolism Model Comparison with One-Station Model
The one-station metabolism modeling approach (streamMetabolizer) described in section “Metabolism Indicators of Wetland Ecological Response to High Flow” could be affected by upstream discontinuities in physical and biological conditions. For example, the source of high flow to the DPM is from the L-67-A canal, which could be different enough to interfere with estimation of metabolism in the DPM wetland. The distance downstream from the discontinuity where the one-station model may be applied is approximated by the turnover length of oxygen by gas exchange between water and air, that is, U/K600, where U (m/day) is a distance-weighted water flow velocity calculated from a tracer experiment in the wetland and K600 (1/day) is the air-water gas exchange constant.
The estimated turnover length for oxygen during the high-flow condition for site RS1D is slightly shorter than the distance between sites S152 and RS1D (446 m), which means the measured DO at RS1D potentially misrepresents the effects of metabolism in the wetland at that site during high flow(table 23). However, the metabolism length provides a very conservative and rough approximation of how upstream discontinuities might affect results. Therefore, we undertook the additional step of comparing the one station modeling approach against a two-station metabolism modeling approach for a short representative time period.
Table 23.
Dissolved oxygen turnover length estimation for low and high-flow conditions using the air-water gas exchange coefficient estimated from dual gas tracer experiment on January 30, 2018.[Information on the experiment can be found in the “Dual Gas Tracer Experiment” section. U, distance-weighted velocity; K600, air-water gas exchange constant]
The two-station model includes the effects of upstream discontinuities by tracking the change in oxygen concentration as it is transported downstream. The two-station model used dissolved oxygen concentrations measured at the S-152 culverts and at RS1D for a two-day period between October 18, 2016, at 13:00:00 and October 20, 2016, at 11:00:00 (fig. 16).
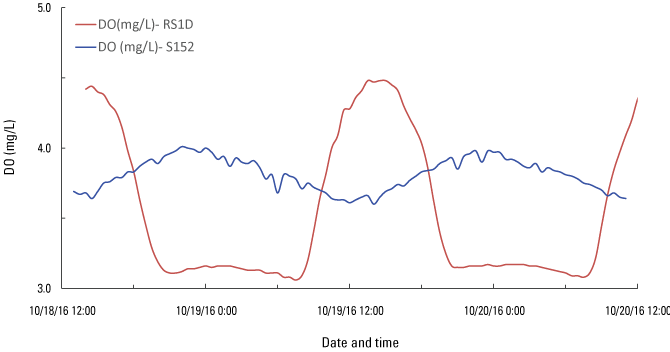
Dissolved oxygen (DO) measurements at S-152 culverts and at RS1D slough during time period of comparison of one and two-station metabolism modeling in the Everglades, Florida. mg/L, milligram per liter.
The transport of dissolved oxygen between two sites (S152 and RS1D) was modeled using the following governing equation:
where C is dissolved oxygen concentration in the main channel (in milligrams per liter [mg/L]); CSAT is saturated dissolved oxygen concentration (mg/L);In the two-station model, ER was inversely estimated by matching the DO concentration during nighttime and then GPP was inversely estimated by matching DO concentration during daytime. The parameters of the two-station model were:
-
1. Simulation time = 166,000 seconds (46 hours);
-
2. Distance from site S152 to site RS1D = 530 m;
-
3. Average velocity = 0.0642 m/s, estimated based on the OTIS modeling of specific conductivity (SC) using distance-weighted average area of the slough ("Dissolved Oxygen and Temperature Modeling”);
-
4. Dispersion coefficient = 0.057 m2/s (from OTIS model of SC);
-
5. Gas exchange rate = 6.09/day, which was acquired from the best-fit streamMetabolizer estimate of K600 for the modeling period (October 18–20, 2016); and
-
6. Water depth = 0.706 m.
The two-station metabolism estimates for the high-flow period (October 18–20, 2016) at site RS1D were −25.6 g O2/m2/day for ER and 4.77 g O2/m2/day for GPP (fig. 17). Those estimated differed by approximately 25 percent from the one-station streamMetabolizer estimates (−32.86 g O2/m2/day and 3.72 g O2/m2/day for ER and GPP, respectively). Those results are in reasonable agreement considering the substantial differences in modeling approach. The one-station model may be in error because it assumes homogenous upstream conditions within a turnover length, and two-station model may be in error because of errors in transport estimation and in measurements at two locations instead of just one. Even during high-flow conditions in DPM when the dissolved oxygen turnover length is shortest, our one- and two-station metabolism model comparison supports the use of the one-station streamMetabolizer metabolism model. We chose the one-station approach because of the availability of the one-station Bayesian state-space model streamMetabolizer that estimates gas exchange as well as GPP and ER, and because it does not require paired stations which permitted us to model metabolism at thirteen sites in DPM.
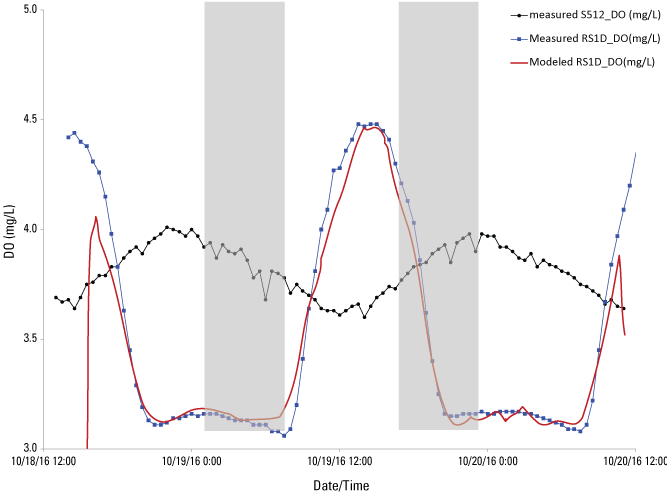
Dissolved oxygen (DO) model estimation at RS1D slough using two-station model with measured DO at S-152 culverts as the upstream boundary condition in the Everglades, Florida. mg/L, milligram per liter.
Results and Discussion
The primary impacts of flow, based on the 1-station streamMetabolizer model, included changes in temperature and DO (fig. 18) and large increases in ER and gas exchange at sites nearest S-152 (fig. 19, table 24). During high-flow, ER at RS1 (−31.81 g O2/m2/d) and Z5-3 (−34.75 g O2/m2/d) sites were double those during low-flow, and 5- to 6-fold higher than at C2 (control). K600 at RS1 and Z5-3B also increased 6.4- and 3.8-fold, respectively, over low-flow values. Using flow-period averages as replicates, t-tests were used to evaluate flow differences for C2 and RS1. These analyses indicated high- and low-flow values were not different at C2 but were significantly different at RS1 for ER (p<10−5), K600 (p<10−5), and NEP (p<0.01). While high flow increased GPP at the intermediate-flow sites, Z5-3B (+68 percent) and Z4-1D (+58 percent), such results are tentative given the shorter periods of record (1 flow) for those sites.
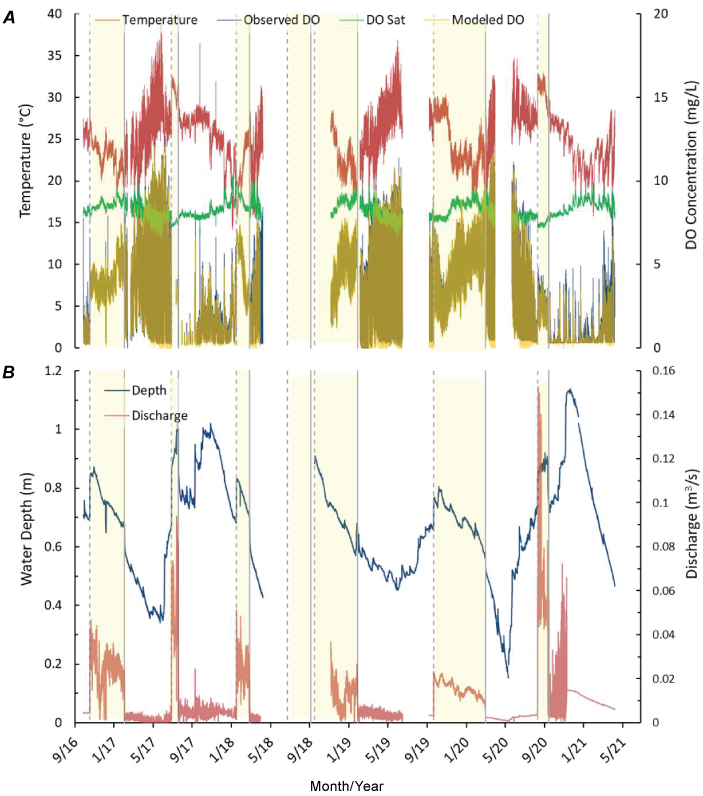
A, Water temperature, dissolved oxygen concentration (DO) and saturated dissolved concentration (DO Sat), and Bayesian partial pooling model predictions of DO at site RS1D-slough. B, Measured discharge (per unit width of wetland) and water depth at RS1D-slough. High-flow release periods from the S-152 culvert indicated by yellow bars. Dates are in month/year. °C, degrees Celsius; m, meter; m3/s, cubic meter per second; mg/L, milligram per liter.
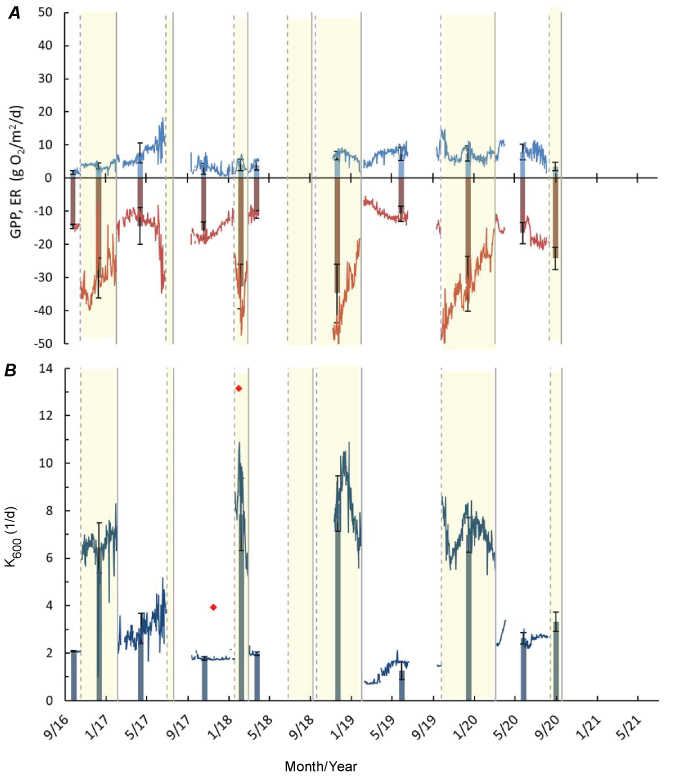
Plots showing metabolism estimates reported as daily values (lines) and flow-period averaged values (bars). A, Gross primary productivity (GPP; light blue lines) and ecosystem respiration (ER; red lines). B, gas exchange rates (K600; dark blue lines). Error bars represent the standard deviation from mean over the flow-period. Red diamonds indicate K600 values (3.9 and 13.2 per day) obtained from dual gas tracer measurements conducted on November 15, 2017, and January 30, 2018, respectively. O2/m2/d, oxygen per square meter per day.
Table 24.
Average daily gross primary productivity, ecosystem respiration, K600, and net ecosystem productivity (±1 standard deviation) estimated by streamMetabolizer.[Record length is longest for RS1D (September 2016–April 2021: 6 high-flow, 7 low-flow periods) and C2 (September 2016–September 2019; 4 high flows, 5 low flows), and shorter for Z5-3B (October 2017–April 2018, one high flow), Z4-1D (same as Z5-3B), and UB1 (September 2018–February 2020; 2 high flows). High-flow velocities flows are approximate values. km, kilometer; cm/s, centimeter per second; GPP, gross primary productivity; g O2/m2/d, gram of oxygen per square meter per day; ER, ecosystem respiration; NEP, net ecosystem productivity; <, less than]
Lessons Learned
Introduction
Restoring a well-functioning Everglades ridge and slough landscape is part of whole system restoration of the Everglades that serves both human needs and ecosystem needs. The patterned landscape with deep water sloughs between intervening ridges improved habitat by increasing primary productivity and providing for dispersal pathways in sloughs that supported biodiverse food webs in sloughs with abundant ridge edges for rearing and refugia. The patterned landscape also provided for wetland water storage in sloughs during droughts and efficient flood conveyance after extreme rainfall.
After over draining the Everglades in the early 20th century, which contributed to loss of the patterned landscape, the system has been managed better by storing water in water conservation areas (WCAs), that is, wetland basins hundreds of thousands of acres in size enclosed by levees. The canals that are adjacent to the levees are the primary conveyors of water that bypass wetlands and deliver water through gated structures into the next downstream basin. Consequently, most flow occurs in canals rather than as natural sheet flow through wetlands. Over time, it became clear that the reduction in sheet flow in the managed Everglades has contributed to the loss of deep-water sloughs and associated ecological benefits of a well-functioning ridge and slough ecosystem (McVoy and others, 2011; Harvey and others, 2017). The overall flattening of the landscape and loss of up to several feet of elevation is attributed to oxidation that had been induced by past drainage as well to a reduction of the velocity of sheet flow through wetlands that had caused a redistribution of sediments entrained by flow in open, deep-water sloughs that was deposited on sawgrass ridges. In addition, the increased phosphorus loading to the Everglades, which was historically a low-nutrient system, also has contributed to degraded functions by replacing the large expanses of well-functioning ridge and slough ecosystem in the Everglades with cattail monocultures (Hagerthey, and others, 2008; Noe and Childers, 2007).
Restoring sheet flow to the Everglades is expected to deepen water depths and increase the velocity of sheet flow which is expected to re-activate the natural redistribution of sediments that can rebuild topography by scouring sloughs and building ridge elevations (Larsen and others, 2009a, b34). Whether sheet flow can easily re-connect wetland basins by flowing across remnant canals without backfilling the canals was a key question addressed by the Decompartmentalization Physical Model (DPM), a multi-agency experiment that was designed and implemented as an adaptive management component of the Comprehensive Everglades Restoration Plan’s Decompartmentalization and Sheet Flow Enhancement Project. DPM tested whether a lack of canal backfilling will counteract the benefits of restored sheet flow by capturing sediments and (or) enriching them with phosphorus. The DPM high-flow experiments were implemented in the heart of the central Everglades and its structures are now becoming a part of the Central Everglades Planning Process (CEPP-South).
Experimental high flows in the DPM provided a testbed to evaluate key factors that can influence restoration success. The role and effectiveness of specific adaptive management features were examined, including radial spreading of high flows from culvert structures in levees as well as creation of broad gaps in levees to convey sheet flow between wetlands that are presently enclosed by the levees. Effects of the Active Marsh Improvement project that re-opened historical sloughs that had become clogged with vegetated in recent decades also was examined. The overall purpose of the management features was to maximize high levels of sheet flow through wetlands and minimize flow bypassing of wetlands through canals in order to hydrate downstream wetlands in Everglades National Park.
Flow Lessons
The limited availability of water of suitable quality is a limitation in restoring sheet flow as it was in previous centuries before drainage began. Much of the available water comes in abundance with tropical storms that threaten flooding and required rapid routing of floodwater through canals toward coastal discharges that damaged estuaries.
To increase water flows through wetlands, culvert flows spread in a radial pattern through the downstream wetlands, causing substantial increases in flow velocity and water depth near the culverts that decayed logarithmically with distance. Beyond a kilometer from the culverts, the high-flow hydraulic effects are a challenge to detect because of the radial spreading.
In their analysis of the pre-drainage Everglades, McVoy and others (2011) concluded that sheet flow velocities were rarely negligible and more often ranged between 1 to as high as 20 centimeters per second (cm/s) at specific locations, based on an interpretation of historical observations as well as modeling. Shear stress associated with high-flow velocities within the low range of estimated pre-drainage flow velocities, that is, between 1.5 and 3cm/s, are likely to entrain organic sediments in sloughs and deposit them on ridges. Flume and field measurements of sediment transport (Larsen and others, 2009a; Harvey and others, 2011) and modeling of the evolution of the Everglades landscape (Larsen and Harvey, 2011) point to the sediment redistribution process as a key process that maintains deep water sloughs interspersed between slightly higher and more densely vegetated ridges.
Flow velocities are currently low (often less than 0.5 cm/s) in the Everglades and sloughs are currently rare in many areas (15 percent or less) where the landscape tends to be uniformly vegetated by sawgrass which has a relatively high roughness value. An improved landscape condition generally means that the slough proportion on the landscape has grown, typically to 55–60 percent, which lowers the landscape-scale roughness since slough vegetation has a lower stem frontal area and a greater stem diameter. Because slough vegetation is sparse relative to ridges, an increase in the slough proportion also increased as well as increasing the connectivity of sloughs, both of which contribute to decreasing the landscape-scale roughness. In our modeling, the landscape condition is captured in a single “upscaled” roughness parameter called “effective” roughness that represents the landscape by accounting for both the average stem density and diameter of the individual communities, as well as relative proportions and spatial arrangement of the vegetation communities, including microtopographic differences.
DPM data collection and modeling indicated that the area of favorable sediment redistribution conditions on the landscape, with sediment entrainment occurring in sloughs and deposition on ridges, is more strongly associated with small perturbations of the current landscape condition affecting landscape-scale roughness than it is to management factors such as total culvert discharge and spreading angle (both affecting flow per unit width of wetland) in controlling how much wetland area is favorably influenced by sediment redistribution. Thus, for a given discharge and spreading angle of culvert flow, a slight improvement in slough area can be self-reinforcing by permitting greater velocities that support a larger area of sediment redistribution from sloughs to ridge, which may help preserve remaining sloughs over large areas.
How much more important are small improvements in landscape condition compared to small increases in water discharge through culverts. We found that small perturbations of landscape conditions were approximately 50 percent more sensitive in affecting the sediment redistribution area than were the management factors. With the high ridge proportions that occur in DPM and WCA 3B at present, the wetland landscape condition is dominated by ridge proportion and sawgrass architecture. Under such high ridge proportions (0.84 and 0.73, respectively, for the pre- and post-AMI cases in the DPM), small changes in the type of slough vegetation does almost nothing to improve entrainment and clearing of organic sediments. However, in a landscape with a higher proportion of sloughs (>35 percent), small changes in the type of slough vegetation becomes increasingly important to how the landscape reacts tohigh-flow restoration. Particularly important is how much spikerush there is, in terms of how much flow velocity and the resulting area of sediment redistribution are improved. If spikerush and periphyton mats are thick in sloughs, there is little improvement with high-flow restoration. But if spikerush has only begun to invade, high-flow restoration can remove thick periphyton mats and the resulting slough improvements can be self-sustaining if high flows are maintained for months per year.
Our research therefore shows that if there is a reasonable amount of remaining slough (for example, above 40 percent) and low or moderate spikerush invasion, that high flow restoration can promote self-reinforcing feedbacks that help preserve the remaining sloughs and that may be self-reinforcing in causing slough expansion into areas of historic sloughs where organic sediment has accumulated. However, it is not yet clear whether recovery of sloughs now clogged with organic sediment can occur with high-flow restoration, especially if spikerush or sawgrass have fully invaded the sloughs. Based on a modeling study, Larsen and Harvey (2011) concluded that reversing slough loss by creating conditions that can re-open sloughs clogged with organic sediment is likely impossible after spikerush has invaded, because of the irreversibility of restarting sediment entrainment in sloughs with high flows once spikerush is established. However, at DPM there was field testing of AMI techniques that remove macrophytes from historical sloughs by herbicide application showed that the kickstarting the expansion of degraded deep-water sloughs is possible. AMI can potentially restore enough sloughs to make major improvements in landscape condition, including supporting a productive and biodiverse Everglades, with greater water storage during droughts that conserves critical dry season feeding habitats. Sloughs also provide the conveyance capacity for extreme high flows that can help minimize the height increase in water levels during extreme events that threaten tree island health.
A key finding from our work is that it is possible to do too much restoration that can harm the Everglades. Our scenario simulations in DPM suggested that restoring a well-functioning landscape condition could cause a lowering of the water depth for a given discharge. Therefore, too much human intervention to restore historical landscape conditions could be deleterious if water depths are decreased to the point of overly drying the landscape. The finding that restoration of historical landscape conditions could result in over drying seems counterintuitive and antithetical to restoration, but it is consistent with the fact that there is not enough clean water available to fully restore the historical levels of water inputs to the Everglades. Given less water for restoration than there was historically, our finding that it could be possible to “overdo” restoration of the landscape and cause more harm by lowering water levels, may be one of our most important “flow” lessons learned.
There is a future potential in using remote sensing spectral signatures to detect changes in vegetation biomass, and therefore in vegetation resistance, that can help make flow modeling a predictive tool that can update and improve flow predictions analyses by incorporating new data about changes on the landscape every few years. For example, such tools can potentially be used to help forecast likely changes in flow that may emerge because of adaptive management projects such as re-opening historical sloughs by selective application of herbicide.
Finally, we reiterate that attempting to restore to match the pre-drainage Everglades conditions may come at a cost because too much restoration could potentially decrease vegetation roughness that could result in excessive lowering of water depths. The reason for concern is that the amount of flow needed to fully restore the Everglades is not expected to be available, and the danger is that too much restoration could cause the water depth to decline such that ridge tops are no longer protected from peat-burning fires. There likely is an optimum ridge proportion with accompanying slough vegetation mixture that balances the availability of clean water for restoration that can result in the desired outcomes for sustaining sloughs with high enough water while protecting tree islands from excessive high water. The “right” balance that has a chance of being self-sustaining is probably on the order of a ridge proportion of 50 percent with sloughs vegetated with only modest amounts of floating periphyton mats and spikerush in addition to deepwater slough species such as water lily.
Moving the discussion to how to connect flows between water conservation areas through levee gaps, a key finding was that most of the water passing through gaps comes from the unblocked canals on either side of the gaps, which is not at all ideal. The proportion of total amount of water passing through the L67-C levee gap at DPM was nearly 70 percent from the L67-C canal, meaning that only about 20 percent of the total flow entering the gap came directly from wetland sheet flow. In other words, most of the water avoided flow through the wetland and instead “short-circuited” the wetlands by flowing through the canal to reach the gap where it passed to the next basin, which has implications for pollutant transport to downstream areas.
A further example of canal bypassing is the influence of the L67-A canal as a major source of water for the DPM high-flow experiments. Wetland sheet flow from WCA 3A is largely blocked from entering the S-152 culverts by spoil mounds on the upstream side across the canal from the S-152 culverts. The proportion of water entering the culverts from wetland sheet flow has not yet been quantified, however, some relevant data suggests that most water passing though the culverts come from the L-67A canal on the upstream side of the levee. Thus, spoil mounds would need to be degraded along canals to stop the blocking of water flow from the interior wetlands from entering the canal and flowing through the culverts.
Water Quality Lessons
Even with strict adherence to phosphorus criteria that prevent culverts from opening when total phosphorus is greater than 10 micrograms per liter (μg/L), higher flows through Everglades wetlands will transport higher total phosphorus loads to downstream areas. This outcome contrasts with the past seventy years of management where canals have been the major transporters of phosphorus, creating points of eutrophication where they discharge to wetlands that evolve toward low-quality cattail habitats. Because much of the transported phosphorus is associated with suspended particulate organic matter, it is likely that restored sheet flow will decrease downstream load of TP with high-flow restoration. What matters then is whether high-flow processes such as sediment redistribution from sloughs to ridges may help slow the rate of downstream movement of the phosphorus front. Suspended sediment capture on sawgrass stems in ridges is likely the most effective process slowing the downstream loading of TP. Interestingly, the observed increase in ecosystem respiration that may help open and maintain sloughs also may quicken the downstream movement of phosphorus by mineralizing more organic matter that releases phosphorus. The AMI management, which clears vegetation with herbicide that then degrades, also may boost phosphorus mineralization and transport substantially more, at least initially until sprayed vegetation completely decays.
Phosphorus contributions from unblocked canals adjacent to levee gaps also continue to be an issue that delivers high amounts of sediment and phosphorus to downstream areas. Regardless of flow condition, the flow from the unblocked L67-C dominated water flow into the gap (ranging from 61 percent to 70 percent) compared with smaller sources from wetland sheet flow (ranging from 30 percent to 39 percent). Therefore, the unblocked L67-C canal interferes with the desired enhancement of sheet flow to improve direct wetland-to-wetland connectivity between the Water Conservation Areas in the central Everglades.
The unblocked L67-C canal also dominates the delivery of constituents, with more than two-thirds of the water (69 percent) and sediment (73 percent) and the majority of the total phosphorus (65 percent) and total particulate phosphorus (59 percent) entering the 914-meter levee gap from the unblocked extensions of the L67-C canal. In contrast, wetland sheet flow accounted for less than half of the throughput of materials into WCA-B, that is, accounting for 31 percent, 27 percent, 35 percent, and 41 percent of the water, sediment, total phosphorus, and total particulate phosphorus entering the levee gap, respectively. Furthermore, the canal water flow from the unblocked L67-C canal enters the gap unevenly from the north end and the south end, with 96 percent entering from the north side of the gap (table 17). The dominance of the north side extension of the L67-C canal is explained by the easterly trending direction of DPM high-flow releases that reach the L67-C canal at a location several kilometers north of the levee gap, an unplanned consequence of DPM design. Our research study of canal backfill functions was therefore confronted by challenges involving the unplanned source contributions from unblocked canals that degrade the intended purpose of maximizing sheet flow. Such canals may need to be partially backfilled or completely backfilled in strategic areas to reduce the short-circuiting flows that they create because canal sediments tend to have high phosphorus, and canal velocities tend to be higher than the wetlands, and therefore the entrainment of canal sediments causes transport of particulate phosphorus to downstream areas. Blocking the L67-C canal flow by compete backfilling should increase the fraction of wetland sheet flow that directly reaches the gap, but a practical concern is that there is not enough fill to backfill the entirety of canals to grade, and there are also concerns about losing recreational opportunities for fishing in canals. It therefore could be difficult to design the right size and spacing of canal “plugs” that match available fill and concerns for preserving recreational opportunities, because canal water could just flow around the plugs through a small area of wetland before re-entering the canal. Hydrodynamic model-based design could be helpful to accomplish the objective of maximizing beneficial wetland flows and minimizing detrimental canal flows.
References Cited
Appling, A.P., Hall, R.O., Jr., Yackulic, C.B., and Arroita, M., 2018a, Overcoming equifinality—Leveraging long time series for stream metabolism estimation: Journal of Geophysical Research. Biogeosciences, v. 123, no. 2, p. 624–645, https://doi.org/10.1002/2017JG004140.
Appling, A.P., Read, J.S., Winslow, L.A., Arroita, M., Bernhardt, E.S., Griffiths, N.A., Hall, R.O., Jr., Harvey, J.W., Heffernan, J.B., Stanley, E.H., Stets, E.G., and Yackulic, C.B., 2018b, The metabolic regimes of 356 rivers in the United States: Scientific Data, v. 5, no. 1, p. 180292, https://doi.org/10.1038/sdata.2018.292.
Arroita, M., Elosegi, A., and Hall, R.O., Jr., 2019, Twenty years of daily metabolism show riverine recovery following sewage abatement: Limnology and Oceanography, v. 64, no. S1, p. S77–S92, https://doi.org/10.1002/lno.11053.
Aquatic Informatics, 2024, Aquarius—Analytics software for the natural environment to monitor flooding, water quality, Stormwater, and more: Aquatic Informatics web page, accessed August 1, 2024, at https://aquaticinformatics.com/products/aquarius-environmental-water-data-management/.
Biron, P.M., Robson, C., Lapointe, M.F., and Gaskin, S.J., 2004, Comparing different methods of bed shear stress estimates in simple and complex flow fields: Earth Surface Processes and Landforms, v. 29, no. 11, p. 1403–1415, https://doi.org/10.1002/esp.1111.
Blaszczak, J.R., Delesantro, J.M., Urban, D.L., Doyle, M.W., and Bernhardt, E.S., 2019, Scoured or suffocated—Urban stream ecosystems oscillate between hydrologic and dissolved oxygen extremes: Limnology and Oceanography, v. 64, no. 3, p. 877–894, https://doi.org/10.1002/lno.11081.
Choi, J., and Harvey, J.W., 2000, Quantifying time-varying groundwater discharge and recharge in wetlands—A comparison of methods in the Florida Everglades: Wetlands, v. 20, no. 3, p. 500–511, https://doi.org/10.1672/0277-5212(2000)020<0500:QTGDAR>2.0.CO;2.
Choi, J., and Harvey, J.W., 2013, Relative significance of microtopography and vegetation as controls on surface water flow on a low-gradient floodplain: Wetlands, v. 34, no. 1, p. 101–115, https://doi.org/10.1007/s13157-013-0489-7.
Choi, J. and Harvey, J.W. (2017), Predicting outcomes of restored Everglades high flow: a model system for scientifically managed floodplains. Restoration Ecology, v. 25, no. S1, p. S39-S47. https://doi.org/10.1111/rec.12479
Choi, J., Harvey, J., Larsen, L., Skalak, K., Buskirk, B., Swartz, A., Lewis, J., Gomez-Velez, J., Maglio, M., Sinclair, G., Langstrom, T., Singh, J., and Walker, A., 2016, High-flow field experiments to inform Everglades restoration—Experimental data for July 2010 to March 2016: U.S. Geological Survey data release, https://doi.org/10.5066/F7PR7T3B.
Choi, J., and Harvey, J., 2023, Hydrologic analysis of flow and modeling of suspended sediment and total phosphorus transport during experimental high flows for the Everglades DPM experiments: U.S. Geological Survey data release, https://doi.org/10.5066/P9ME2FLN.
Davis, S.M., Gunderson, L.H., Park, W.A., Richardson, J.R., and Mattson, J.E., 1994, Landscape dimension, composition, and function in a changing Everglades ecosystem, chap. 17 of Davis, S.M., and Ogden, J.C., eds., Everglades—The ecosystem and its restoration: St. Lucie, Delray Beach, CRC Press, p. 419–444, https://doi.org/10.1201/9781466571754-25.
Davis, S.M., Childers, D.L., Lorenz, J.J., Wanless, H.R., and Hopkins, T.E., 2005a, A conceptual model of ecological interactions in the mangrove estuaries of the Florida Everglades: Wetlands, v. 25, no. 4, p. 832–842, https://doi.org/10.1672/0277-5212(2005)025[0832:ACMOEI]2.0.CO;2.
Davis, S.M., Gaiser, E.E., Loftus, W.F., and Huffman, A.E., 2005b, Southern marl prairies conceptual ecological model: Wetlands, v. 25, no. 4, p. 821–831. https://doi.org/10.1672/0277-5212(2005)025[0821:SMPCEM]2.0.CO;2.
Everglades Depth Estimation Network (EDEN), 2020, Everglades Depth Estimation Network (EDEN) for support of biological and ecological assessments—Explore and view EDEN (EVE): U.S. Geological Survey website, accessed December 20, 2022, at https://sofia.usgs.gov/eden/eve/index.php.
Goring, D.G., and Nikora, V.I., 2002, Despiking Acoustic Doppler Velocimeter Data: Journal of Hydraulic Engineering (New York, N.Y.), v. 128, no. 1, p. 117–126. https://doi.org/10.1061/(ASCE)0733-9429(2002)128:1(117).
Hagerthey, S.E., Newman, S., Rutchey, K., Smith, E.P., and Godin, J., 2008, Multiple regime shifts in a subtropical peatland—Community-specific thresholds to eutrophication: Ecological Monographs, v. 78, no. 4, p. 547–565, https://doi.org/10.1890/07-0538.1.
Harvey, J.W., Krupa, S.L., and Krest, J.M., 2004, Ground water recharge and discharge in the central Everglades: Ground Water, v. 42, no. 7, p. 1090–1102, https://doi.org/10.1111/j.1745-6584.2004.tb02646.x.
Harvey, J.W., Schaffranek, R.W., Noe, G.B., Larsen, L.G., Nowacki, D.J., and O’Connor, B.L., 2009, Hydroecological factors governing surface-water flow on a low gradient floodplain: Water Resources Research, v. 45, no. 3, article no. W03421, 20 p., https://doi.org/10.1029/2008WR007129.
Harvey, J.W., Noe, G.B., Larsen, L.G., Nowacki, D.J., and McPhillips, L.E., 2011, Field flume reveals aquatic vegetation’s role in sediment and particulate phosphorus transport in a shallow aquatic ecosystem: Geomorphology, v. 126, nos. 3–4, p. 297–313, https://doi.org/10.1016/j.geomorph.2010.03.028.
Harvey, J.W., Choi, J., Larsen, L., Skalak, K., Psaltakis, J.W., Buskirk, B.A., Swartz, A.G., Lewis, J.M., Gomez-Velez, J.D., Maglio, M.M., Langstrom, T., and Walker, A., 2018, High-flow field experiments to inform Everglades restoration—Experimental data 2010 to 2018: U.S. Geological Survey data release, https://doi.org/10.5066/P9T2624X.
Harvey, J.W., Choi, J., Larsen, L.G., Skalak, K.J., Maglio, M., Quion, K., Lin, T., Psaltakis, J., Buskirk, B., Swartz, A., Lewis, J., Gomez-Velez, J., and Schmadel, N., 2022a, High-flow field experiments to inform Everglades restoration—Experimental data 2010 to 2022 (ver. 2.0, October 2023): U.S. Geological Survey data release, https://doi.org/10.5066/P9SP0HM1.
Harvey, J.W., Choi, J., Quion, K., Lin, T., Swartz, A., Larsen, L.G., Haase, K., and Schmadel, N., 2022b, High-flow experimental outcomes for Everglades hydraulics and aquatic metabolism: U.S. Geological Survey data release, https://doi.org/10.5066/P9A9SQ85.
Harvey, J.W., and Choi, J., 2022a, Biophysical Methods and Data Analysis for Simulating Overland Flow in the Everglades: ESS Open Archive, 51 p., https://doi.org/10.1002/essoar.10511451.1.
Harvey, J.W., and Choi, J., 2022b, Biophysical data for simulating overland flow in the Everglades: U.S. Geological Survey data release, https://doi.org/10.5066/P9DQYB1O.
Harvey, J.W., Wetzel, P.R., Lodge, T.E., Engel, V.C., and Ross, M.S., 2017, Role of a naturally varying flow regime in Everglades restoration: Restoration Ecology, v. 25, no. S1, p. S27–S38, https://doi.org/10.1111/rec.12558.
Ho, D.T., Engel, V.C., Ferrón, S., Hickman, B., Choi, J., and Harvey, J.W., 2018, On factors influencing air-water gas exchange in emergent wetlands: Journal of Geophysical Research. Biogeosciences, v. 123, no. 1, p. 178–192, https://doi.org/10.1002/2017JG004299.
Holtgrieve, G.W., Schindler, D.E., Branch, T.A., and A’mar, Z.T., 2010, Simultaneous quantification of aquatic ecosystem metabolism and reaeration using a Bayesian statistical model of oxygen dynamics: Limnology and Oceanography, v. 55, no. 3, p. 1047–1063, https://doi.org/10.4319/lo.2010.55.3.1047.
Ivanoff, D.B., Reddy, K.R., and Robinson, S., 1998, Chemical fractionation of organic phosphorus in selected histosols: Soil Science, v. 163, no. 1, p. 36–45, https://doi.org/10.1097/00010694-199801000-00006.
Knight, T.M., and Soderqvist, L.E, 2021, Water velocity mapping of the L-67C canal in support of the decompartmentalization physical model (DPM): U.S. Geological Survey data release, https://doi.org/10.5066/P9UOVNZO.
Lal, A.M.W., Moustafa, M.Z., and Wilcox, W.M., 2015, The use of discharge perturbations to understand in situ vegetation resistance in wetlands: Water Resources Research, v. 51, no. 4, p. 2477–2497, https://doi.org/10.1002/2014WR015472.
Larsen, L.G., Harvey, J.W., and Crimaldi, J.P., 2009a, Morphologic and transport properties of natural floc: Water Resources Research, v. 45, no. 1, 13 p., https://doi.org/10.1029/2008WR006990.
Larsen, L.G., Harvey, J.W., and Crimaldi, J.P., 2009b, Predicting bed shear stress and its role in sediment dynamics and restoration potential of the Everglades and other vegetated flow systems: Ecological Engineering, v. 35, no. 12, p. 1773–1785, https://doi.org/10.1016/j.ecoleng.2009.09.002.
Larsen, L.G., and Harvey, J.W., 2011, Modeling of hydroecological feedbacks predicts distinct classes of landscape pattern, process, and restoration potential in shallow aquatic ecosystems: Geomorphology, v. 126, p. 279–296, https://doi.org/10.1016/j.geomorph.2010.03.015.
Larsen, L.G., Ma, J., and Kaplan, D., 2017a, How important is connectivity for surface water fluxes? A generalized expression for flow through heterogeneous landscapes: Geophysical Research Letters, v. 44, no. 20, p. 10349–10358, https://doi.org/10.1002/2017GL075432.
Larsen, L. G., S.Newman, C.Saunders, and J. W.Harvey, 2017b, Complex networks of functional connectivity in a wetland reconnected to its floodplain: Water Resources Research, v. 53, no. 7, p. 6089–6108, https://doi.org/10.1002/2017WR020375.
Lightbody, A.F., and Nepf, H.M., 2006, Prediction of velocity profiles and longitudinal dispersion in salt marsh vegetation: Limnology and Oceanography, v. 51, no. 1, p. 218–228, https://doi.org/10.4319/lo.2006.51.1.0218.
Martin, V., Fisher, T.S.R., Millar, R.G., and Quick, M.C., 2002, ADV Data Analysis for Turbulent Flows—Low Correlation Problem, in Wahl, T.L., Pugh, C.A., Oberg, K.A., and Vermeyen, T.B., eds., Hydraulic measurements and experimental methods 2002—Hydraulic measurements and experimental methods specialty conference, July 28, 2002: American Society of Civil Engineers, 10 p., https://doi.org/10.1061/40655(2002)101.
Nepf, H.M., 1999, Drag, turbulence, and diffusion in flow through emergent vegetation: Water Resources Research, v. 35, no. 2, p. 479–489, https://doi.org/10.1029/1998WR900069.
Noe, G.B., and Childers, D.L., 2007, Phosphorus budgets in Everglades wetland ecosystems—The effects of hydrology and nutrient enrichment: Wetlands Ecology and Management, v. 15, no. 3, p. 189–205, https://doi.org/10.1007/s11273-006-9023-5.
Noe, G.B., Harvey, J.W., Schaffranek, R.W., and Larsen, L.G., 2010, Controls of suspended sediment concentration, nutrient content, and transport in a subtropical wetland: Wetlands, v. 30, no. 1, p. 39–54, https://doi.org/10.1007/s13157-009-0002-5.
Nortek, 2020, Vectrino—Technical specifications: Nortek web page, accessed August 15, 2022, at https://www.nortekgroup.com/export/pdf/Vectrino.pdf.
Ogden, J.C., Browder, J., Gentile, J.H., Gunderson, L.H., Fennema, R., and Wang, J., 1999, Environmental management scenarios—Ecological implications: Urban Ecosystems, v. 3, no. 3, p. 279–303, https://doi.org/10.1023/A:1009508718195.
Ogden, J.C., Davis, S.M., and Brandt, L.A., 2003, Science strategy for a regional ecosystem monitoring and assessment program—The Florida Everglades example, chap. 5 of Busch, D.E., and Trexler, J.C., eds., Monitoring systems—Interdisciplinary approaches for evaluating ecoregional initiatives: Washington, D.C., Island Press, p. 135–160.
Ogden, J.C., Davis, S.M., Jacobs, K.J., Barnes, T., and Fling, H.E., 2005, The use of conceptual ecological models to guide ecosystem restoration in south Florida: Wetlands, v. 25, p. 795–809, https://doi.org/10.1672/0277-5212(2005)025[0795:TUOCEM]2.0.CO;2.
Onset, 2022, Air/Water/Soil temperature sensor: Onset web page, accessed August 30, 2022, at https://www.onsetcomp.com/products/sensors/tmcx-hd/.
Payn, R.A., Hall, R.O., Jr., Kennedy, T.A., Poole, G.C., and Marshall, L.A., 2017, A coupled metabolic-hydraulic model and calibration scheme for estimating whole-river metabolism during dynamic flow conditions: Limnology and Oceanography: Methods, v. 15, no. 10, p. 847–866, https://doi.org/10.1002/lom3.10204.
Regier, P., He, D., Saunders, C.J., Jara, B., Hansen, C., Newman, S., Tate-Boldt, E., Coronado-Molina, C., and Jaffé, R., 2018, Sheet flow effects on sediment transport in a degraded ridge and-slough wetland—Insights using molecular markers: Journal of Geophysical Research. Biogeosciences, v. 123, no. 10, p. 3124–3139, https://doi.org/10.1029/2018JG004648.
Riscassi, A.L., and Schaffranek, R.W., 2004, Flow velocity, water temperature, and conductivity in Shark River Slough, Everglades National Park, Florida—June 2002–July 2003: U.S. Geological Survey Open-File Report 2004–1233, 64 p., https://doi.org/10.3133/ofr20041233.
Rudnick, D.T., Ortner, P.B., Browder, J.A., and Davis, S.M., 2005, A conceptual ecological model of Florida Bay: Wetlands, v. 25, no. 4, p. 870–883, https://doi.org/10.1672/0277-5212(2005)025[0870:ACEMOF]2.0.CO;2.
Said, W. P., and Brown, M. C., 2013, Hydrologic simulation of the predrainage greater Everglades using the Natural system regional simulation model v3.5.2: West Palm Beach, Fla., South Florida Water Management District, Water Resources Division, 626 p., accessed August 1, 2024, at https://www.sfwmd.gov/sites/default/files/documents/nsrsm_v3_5_2_documentation.pdf.
Science Coordination Team, 2003, The role of flow in the Everglades ridge and slough landscape: South Florida Ecosystem Restoration Working Group, accessed December 1, 2005, at http://sofia.usgs.gov/publications/papers/sct_flows/index.html.
Sequoia Scientific, Inc., 2009a, LISST-100x particle size analyzer user’s manual version 4.65: Bellevue, WA, Sequoia Scientific, Inc., accessed December 14, 2022, at https://www.sequoiasci.com/wp-content/uploads/2013/07/manual-4-65.pdf.
Sequoia Scientific, Inc., 2009b, LISST bin sizes: Bellevue, WA, Sequoia Scientific, Inc., accessed August 29, 2022, at https://www.sequoiasci.com/article/lisst-bin-sizes/.
Sequoia Scientific, Inc., 2019, LISST-Portable|XR Manual, Version 1.5: Bellevue, WA, Sequoia Scientific, Inc., accessed December 14, 2022, at https://www.sequoiasci.com/wp-content/uploads/2015/06/LISST-PortableXR-Manual-Version-1_5-for-firmware-2.pdf.
Sequoia Scientific, Inc., 2022, LISST-100X: Bellevue, WA, Sequoia Scientific, Inc., accessed August 29, 2022, at https://www.sequoiasci.com/product/lisst-100x/.
SonTek, 2015, SonTek-IQ series standard, plus and pipe—Flow, total volume, level and velocity: San Diego, Calif., SonTek, 4 p., accessed December 14, 2022, at https://www.ysi.com/File%20Library/Documents/Brochures%20and%20Catalogs/sontek-iq-brochure.pdf.
SonTek, 2021a, Argonaut-ADV—Ultra-precise 3-D velocity measurement: San Diego, Calif., SonTek, 2 p., accessed December 14, 2022, at https://www.ysi.com/File%20Library/Documents/Brochures%20and%20Catalogs/argonaut-adv-brochure.pdf.
SonTek, 2021b, Argonaut-XR—Multi-cell doppler current profiler: San Diego, Calif., SonTek, 2 p., accessed December 14, 2022, at https://www.ysi.com/File%20Library/Documents/Brochures%20and%20Catalogs/argonaut-xr-brochure.pdf.
SonTek, 2022, FlowTracker2 ADV—Versatile wading discharge & lab acoustic doppler velocimeter: San Diego, Calif., SonTek, 7 p., accessed December 14, 2022, at https://www.ysi.com/File%20Library/Documents/Brochures%20and%20Catalogs/flowtracker2-brochure.pdf.
South Florida Water Management District [SFWMD], 2005, Table 2.4.2.1.—Overland flow coefficients for effective roughness as used in the south Florida water management model (cell-to-cell overland flow) in Documentation of the south Florida water management model—Version 5.5: West Palm Beach, Fla., South Florida Water Management District, accessed May 22, 2024, at https://www.sfwmd.gov/sites/default/files/documents/sfwmm_final_121605.pdf.
Stallman, R., 1965, Steady one-dimensional fluid flow in a semi-infinite porous medium with sinusoidal surface temperature: Journal of Geophysical Research, v. 70, no. 12, p. 2821–2827, https://doi.org/10.1029/JZ070i012p02821.
TE Connectivity, 2017, KPSI 501: TE Connectivity web page, 4 p., accessed December 14, 2022, at https://www.te.com/commerce/DocumentDelivery/DDEController?Action=showdoc&DocId=Data+Sheet%7FKPSI_501%7FA5%7Fpdf%7FEnglish%7FENG_DS_KPSI_501_A5.pdf%7F CAT-WLS0018.
U.S. Geological Survey [USGS], 2016, USGS water data for the Nation: U.S. Geological Survey National Water Information System database, accessed June 10, 2016, at http://waterdata.usgs.gov/nwis/.
Wanninkhof, R., Asher, W., Weppernig, R., Chen, H., Schlosser, P., Langdon, C., and Sambrotto, R., 1993, Gas transfer experiment on Georges Bank using two volatile deliberate tracers: Journal of Geophysical Research, v. 98, no. C11, p. 20237–20248, https://doi.org/10.1029/93JC01844.
White, J.T., Hunt, R.J., Fienen, M.N., and Doherty, J.E., 2020, Approaches to highly parameterized inversion—PEST++ version 5, a software suite for parameter estimation, uncertainty analysis, management optimization and sensitivity analysis: U.S. Geological Survey Techniques and Methods 7C26, 51 p., https://doi.org/10.3133/tm7C26.
Winter, T.C., 1981, Uncertainties in estimating the water balance of lakes: Journal of the American Water Resources Association, v. 17, no. 1, p. 82–115, https://doi.org/https://doi.org/10.1111/j.1752-1688.1981.tb02593.x.
Yamartino, R.J., 1984, A comparison of several “single-pass” estimators of the standard deviation of wind direction: Journal of Climate and Applied Meteorology, v. 23, no. 9, p. 1362–1366, https://doi.org/10.1175/1520-0450(1984)023<1362:ACOSPE>2.0.CO;2.
YSI, Inc., 2022a, 6136 turbidity sensor: YSI Incorporated webpage, accessed August 30, 2022, at https://www.ysi.com/accessory/id-6136/6136-turbidity-sensor.
YSI, Inc., 2022b, 6560 temperature/conductivity sensor: YSI Incorporated webpage, accessed August 30, 2022, at https://www.ysi.com/accessory/id-6560/6560-temperatureconductivity-sensor.
YSI, Inc., 2022c, 6562 rapid pulse dissolved oxygen sensor: YSI Incorporated webpage, accessed August 30, 2022, at https://www.ysi.com/accessory/id-6562/6562-rapid-pulse-dissolved-oxygen-sensor.
YSI, Inc., 2022d, EXO wiped conductivity & temperature sensor: YSI Incorporated webpage, accessed September 29, 2022, at https://www.ysi.com/WipedCT.
YSI, Inc., 2022e, EXO optical dissolved oxygen smart sensor: YSI Incorporated webpage, accessed September 29, 2022, at https://www.ysi.com/Product/id-599100-01/EXO-Optical-Dissolved-Oxygen-Smart-Sensor.
Appendix 1. Aerial Images of DPM
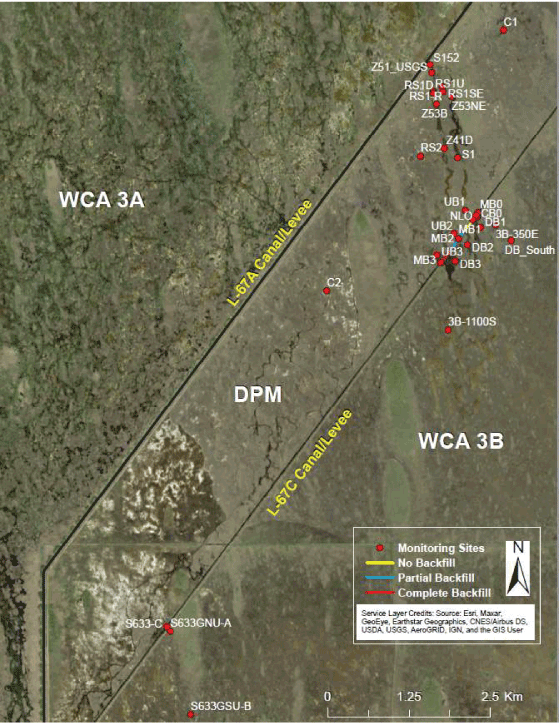
Aerial image of the Decompartmentalization Physical Model (DPM) experimental site located between the L-67A and L-67C canal and levees. This figure is a larger scale version of figure 1 in the main text and includes sites to the southwest located within and downstream of the S-633 gap in the L67-C levee.
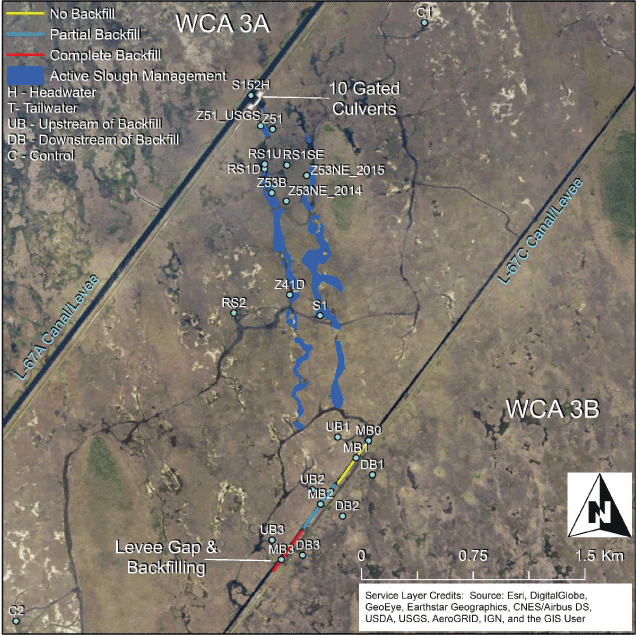
Aerial image of the Decompartmentalization Physical Model (DPM) experimental site located between the L-67A and L-67C canal/levees. This version highlights the areas of active slough vegetation management, also known as Active Marsh Improvement (AMI) project, where herbicide was applied to remove macrophytes from selected historical sloughs that had become overgrown with sawgrass (described in the “Active Marsh Improvement” section of the main text).
Appendix 2. S-152 Culvert Discharge Measurements
Table 2.1.
Discharge measurements at the outlet, or east end, of S-152 during the 2013–14 experiment.[October 2013–September 2014 (U.S. Geological Survey, 2022). Dates shown as month/day/year. Times shown as HH:MM:SS in Eastern daylight time or Eastern standard time depending on date.]
Table 2.2.
Discharge measurements at the outlet, or east end, of S-152 during the 2014–15 experiment.[October 2014–September 2015 (U.S. Geological Survey, 2022). Dates shown as month/day/year. Times shown as HH:MM:SS in Eastern daylight time or Eastern standard time depending on date]
Table 2.3.
Discharge measurements at the outlet, or east end, of S-152 during the 2015–16 experiment.[October 2015–September 2016 (U.S. Geological Survey, 2022). Dates shown as month/day/year. Times shown as HH:MM:SS in Eastern daylight time (ET) or Eastern standard time (EST) depending on date.]
Discharges were measured during emergency flow releases (U.S. Geological Survey streamgage station no. 255154080371300; U.S. Geological Survey, 2022).
Table 2.4.
Discharge measurements at the outlet, or east end, of S-152 during the 2016–17 experiment.[October 2016–September 2017 (U.S. Geological Survey , 2022). Dates shown as month/day/year. Times shown as HH:MM:SS in Eastern daylight time or Eastern standard time depending on date.]
Discharges were measured during emergency flow releases (U.S. Geological Survey streamgage station no. 255154080371300; U.S. Geological Survey, 2022).
Table 2.5.
Discharge measurements at the outlet, or east end, of S-152 during the 2017–18 experiment.[October 2017–September 2018 (U.S. Geological Survey, 2022). Dates shown as month/day/year. Times shown as HH:MM:SS in Eastern daylight time or Eastern standard time depending on date.]
Table 2.6.
Discharge measurements at the outlet, or east end, of S-152 during the 2018–19 experiment.[October 2018–September 2019 (U.S. Geological Survey, 2022). Dates shown as month/day/year. Times shown as HH:MM:SS in Eastern daylight time or Eastern standard time depending on date.]
Table 2.7.
Discharge measurements at the outlet, or east end, of S-152 during the 2019–20 experiment.[October 2019–September 2020 (U.S. Geological Survey, 2022). Dates shown as month/day/year. Times shown as HH:MM:SS in Eastern daylight time or Eastern standard time depending on date.]
Table 2.8.
Discharge measurements at the outlet, or east end, of S-152 during the 2021–22 experiment.[December 2021–March 2022 (U.S. Geological Survey, 2022). Dates shown as month/day/year. Times shown as HH:MM:SS in Eastern daylight time or Eastern standard time depending on date.]
Reference Cited
U.S. Geological Survey, 2022, USGS water data for the Nation: U.S. Geological Survey National Water Information System database, accessed August 29, 2022, at https://doi.org/10.5066/F7P55KJN.
Conversion Factors
International System of Units to U.S. customary units
Multiply | By | To obtain |
---|---|---|
centimeter (cm) | 0.3937 | inch (in.) |
millimeter (mm) | 0.03937 | inch (in.) |
meter (m) | 3.281 | foot (ft) |
kilometer (km) | 0.6214 | mile (mi) |
meter (m) | 1.094 | yard (yd) |
square meter (m2) | 0.0002471 | acre |
hectare (ha) | 2.471 | acre |
square kilometer (km2) | 247.1 | acre |
square meter (m2) | 10.76 | square foot (ft2) |
hectare (ha) | 0.003861 | square mile (mi2) |
square kilometer (km2) | 0.3861 | square mile (mi2) |
6.290 | barrel (petroleum, 1 barrel = 42 gal) | |
liter (L) | 33.81402 | ounce, fluid (fl. oz) |
liter (L) | 2.113 | pint (pt) |
liter (L) | 1.057 | quart (qt) |
liter (L) | 0.2642 | gallon (gal) |
cubic meter (m3) | 264.2 | gallon (gal) |
cubic meter (m3) | 0.0002642 | million gallons (Mgal) |
cubic centimeter (cm3) | 0.06102 | cubic inch (in3) |
liter (L) | 61.02 | cubic inch (in3) |
cubic meter (m3) | 35.31 | cubic foot (ft3) |
cubic meter (m3) | 1.308 | cubic yard (yd3) |
cubic meter (m3) | 0.0008107 | acre-foot (acre-ft) |
cubic meter per second (m3/s) | 70.07 | acre-foot per day (acre-ft/d) |
meter per second (m/s) | 3.281 | foot per second (ft/s) |
meter per day (m/d) | 3.281 | foot per day (ft/d) |
cubic meter per second (m3/s) | 35.31 | cubic foot per second (ft3/s) |
cubic meter per second (m3/s) | 22.83 | million gallons per day (Mgal/d) |
millimeter per year (mm/yr) | 0.03937 | inch per year (in/yr) |
gram (g) | 0.03527 | ounce, avoirdupois (oz) |
kilogram (kg) | 2.205 | pound avoirdupois (lb) |
gram per cubic centimeter (g/cm3) | 62.4220 | pound per cubic foot (lb/ft3) |
liter per second per meter ([L/s]/m) | 4.831 | gallon per minute per foot ([gal/min]/ft) |
Temperature in degrees Celsius (°C) may be converted to degrees Fahrenheit (°F) as follows:
°F = (1.8 × °C) + 32.
Temperature in degrees Fahrenheit (°F) may be converted to degrees Celsius (°C) as follows:
°C = (°F – 32) / 1.8.
Datums
Vertical coordinate information is referenced to the North American Vertical Datum of 1988 (NAVD 88).
Abbreviations
ADV
acoustic Doppler velocimeter
ADVM
acoustic Doppler velocity meter
ADCP
acoustic Doppler current profiler
AMI
active marsh improvement
BP
barometric pressure
BioFRE
biophysical flow rate expression
CPVC
chlorinated polyvinyl chloride
CEPP-S
Central Everglades Planning Project for the southern Everglades
CERP
Comprehensive Everglades Restoration Plan
CTD
convention temperature device
DCI
directional connectivity index
DPM
Decompartmentalization Physical Model
DECOMP
Decompartmentalization and Sheet Flow Enhancement Project
DO
dissolved oxygen
DS
dense spikerush
DSP
dense spikerush with periphyton
DTW
down to water
DWS
deep water slough
ER
ecosystem respiration
ERA
ERA-Waters Corporation
EDEN
Everglades Depth Estimation Network
GPP
gross primary productivity
KPSI
kilopound per square inch
LISST
laser in situ scattering and transmissometry
LOI
loss on ignition
MDL
minimum detectable level
NAVD 88
North American Vertical Datum of 1988
NEP
net ecosystem productivity
NGS
National Geodetic Survey
NIST
National Institute of Standards and Technology
OTIS
one-dimensional transport with inflow and storage model (Runkel, 1998)
PQL
practical quantitation limit
PEST
Parameter Estimation Software Tool (White and others, 2020)
PPFD
photosynthetic photon flux density
QA
quality assurance
QC
quality control
RM
reference marker
SNR
signal-to-noise ratio
SFWMD
South Florida Water Management District
SS
sparse spikerush
SC
specific conductivity
SSC
suspended sediment concentration
TC
total carbon concentration
TDP
total dissolved phosphorus concentration
TN
total nitrogen concentration
TP
total phosphorus concentration
TPP
total particulate phosphorus concentration
USGS
U.S. Geological Survey
WBL
Wetland Biological Laboratory
WCA
Water Conservation Area
Disclaimers
Any use of trade, firm, or product names is for descriptive purposes only and does not imply endorsement by the U.S. Government.
Although this information product, for the most part, is in the public domain, it also may contain copyrighted materials as noted in the text. Permission to reproduce copyrighted items must be secured from the copyright owner.
Suggested Citation
Harvey, J., Choi, J., Larsen, L., Skalak, K., Maglio, M., Quion, K., Swartz, A., Lin, J.T.Y., Gomez-Velez, J., and Schmadel, N., 2024, High-flow experimental outcomes to inform Everglades restoration, 2010–22: U.S. Geological Survey Open-File Report 2024–1063, 72 p., https://doi.org/10.3133/ofr20241063.
ISSN: 2331-1258 (online)
Study Area
Publication type | Report |
---|---|
Publication Subtype | USGS Numbered Series |
Title | High-Flow Experimental Outcomes to Inform Everglades Restoration, 2010–22 |
Series title | Open-File Report |
Series number | 2024-1063 |
DOI | 10.3133/ofr20241063 |
Year Published | 2024 |
Language | English |
Publisher | U.S. Geological Survey |
Publisher location | Reston VA |
Contributing office(s) | WMA - Earth System Processes Division |
Description | Report: xi, 72 p.; 3 Data Releases |
Country | United States |
State | Florida |
Other Geospatial | Everglades |
Online Only (Y/N) | Y |