Remote Sensing for Monitoring Mine Lands and Recovery Efforts
Links
- Document: Report (8.88 MB pdf) , HTML , XML
- Download citation as: RIS | Dublin Core
Abstract
Under the Bipartisan Infrastructure Law Ecosystem Restoration Program, the U.S. Department of the Interior has invested in assessing and recovering degraded ecosystems to promote healthy human communities and wildlife habitats. One priority established by the program is the need to address degraded ecosystems associated with mine lands, including active, inactive, and abandoned mines. Mine lands occur in every State of the United States and present a range of environmental hazards and safety risks to human communities and wildlife habitats. However, limited information compiled across the United States exists on the whereabouts of mining activities and their potential environmental effects on landscapes. Remote sensing is the process of acquiring information about the landscape from ground platforms, aircraft, or satellites to assess surface characteristics such as topography, vegetation, and soil properties and can therefore provide important cost-effective methods for identifying mining sites and assessing their environmental effects. Based on a literature review of remote sensing applications that assessed land health conditions and monitoring of mine lands, this report highlights important approaches, capabilities, considerations, and case studies using a breadth of techniques. The report identifies considerations for setting appropriate vegetation recovery targets and demonstrates how remote sensing can inform the prioritization, recovery design, and long-term assessments of recovery to help support decision makers and land managers. Applications of remote sensing to mine recovery will be most effective when recovery targets are clearly defined and quantifiable from data collected before mining activity, per the Surface Mining Control and Reclamation Act of 1977 and similar laws. Additionally, the collected data would need to be accessible and maintained in databases for baseline references used to establish these recovery targets.
Introduction
The Bipartisan Infrastructure Law, also known as the Infrastructure Investment and Jobs Act (Public Law 117–58), was passed by the U.S. Congress in 2021. The Bipartisan Infrastructure Law, in part, funds the recovery of degraded ecosystems to promote healthy human communities and wildlife habitat. Under the Law, the U.S. Department of the Interior established a priority to identify the need to address degraded ecosystems associated with mine lands, including active, inactive, and abandoned mine lands and areas adjacent to, and affected by, current and past (primarily since the mid-19th century) mining activity. Mine lands present a range of environmental hazards and safety risks to human communities and wildlife habitats and are in every U.S. State (fig. 1). As a result, the Department aims to effectively prioritize, execute, and track risk mitigations and recovery efforts on mine lands across the United States using new geospatial web tools for decision makers and land managers. The prioritization of recovery of mine lands requires myriad data including inventories of mining activity and hazards, and status of site conditions (for example, hydrology, soils, vegetation, and ecosystem health). In addition, methods for assessing recovery progress, and tools to support decision making are needed to make cost-effective choices to prioritize sites for recovery. Remote sensing is one tool that can aid site condition assessments, track recovery success, and support decision making. Remote sensing is the process of acquiring reflected and emitted radiation at a distance—typically from ground platforms, satellites, or aircraft—to assess surface characteristics such as vegetation properties (Lillesand and others, 2015).
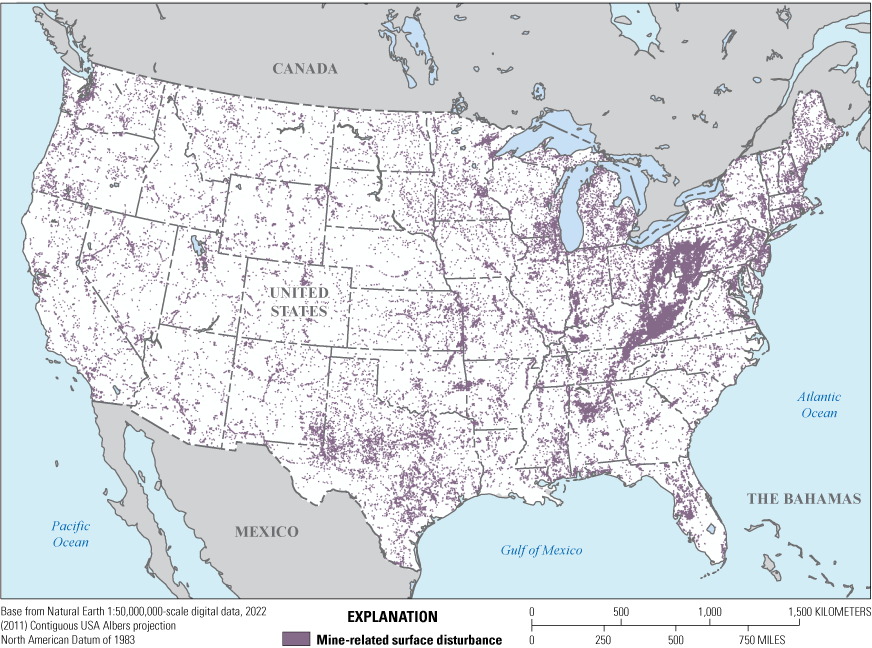
Mine-related surface disturbances in the continental United States. The displayed surface-related mine polygons (pits, strip mines, trenches, quarries, diggings, slag piles, mine dumps, and glory holes) were selected from the mine- and prospect-related symbols dataset (1:24,000-scale) that was digitized and modified from U.S. Geological Survey 7.5- and 15-minute topographic quadrangle maps (Horton and San Juan, 2016). Data portrayed here are historical and do not reflect mining activity after 2006.
Throughout the report, the word “recovery” is broadly used to refer to returning mine lands to their premined condition or a determined environmental target (for example, fig. 2); the term encompasses reclamation, remediation, rehabilitation, and restoration. However, these terms are used differently throughout the general restoration literature, so the definitions provided by Lima and others (2016) are presented here to provide clarity and bold terms are defined in the “Glossary” section of the report. Reclamation refers to the geotechnical stabilization of land (for example, soils and topography), allowing for the return of flora and fauna or species that are endemic to the area but not necessarily the same composition or exact condition as predisturbance. Remediation focuses on removing contaminants from the environment (soils, water, and air) and managing risks to humans and wildlife. Restoration generally refers to actions resulting in the recovery of an ecosystem to its original state prior to disturbance. Unlike restoration, rehabilitation aims to reinstate ecosystem services that benefit human communities and livelihoods (such as farming or development).
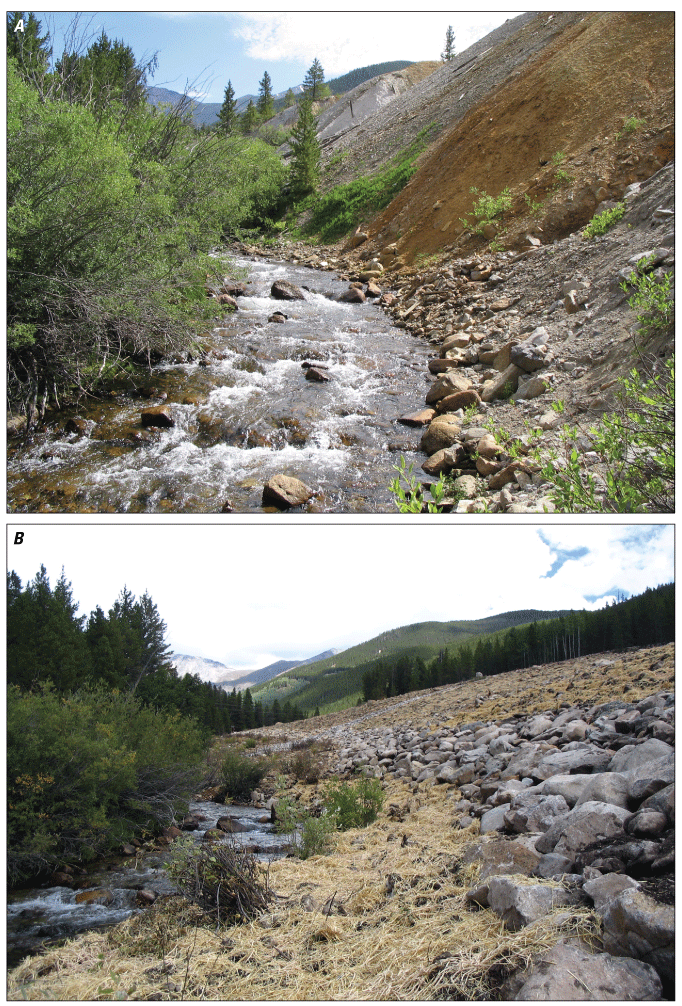
The Akron Mine and Mill Site is an abandoned mine and ore processing site near Whitepine, Colorado, that was active from 1885 to 1893. A, Waste rock and tailings in direct contact with Tomichi Creek [Photograph by U.S. Department of Agriculture Forest Service Rocky Mountain Region]. B, Restoration actions that were completed in August 2016 that removed waste material away from the creek and restored the affected channel and floodplain [Photograph by U.S. Department of Agriculture Forest Service Rocky Mountain Region].
Here, based on a literature review of remote sensing studies, we summarize how remote sensing methods and data can be used to evaluate mine land condition and monitor mine recovery. Specifically, we provide (1) background information on mining practices, their potential environmental effects, and laws guiding recovery efforts; (2) an overview of remote sensing and its use in assessing mine land condition and recovery; (3) considerations for setting appropriate recovery targets and how different types of targets may relate to different types of remote sensing; (4) example studies using remote sensing for monitoring mine land condition and recovery (app. 1); (5) concluding remarks that highlight the benefits and potential shortcomings and challenges associated with using remote sensing to assess mine land recovery; and (6) a nonexhaustive list of helpful resources for potential users (for example, regulatory documents, web pages, and literature review publications [app. 2]). This review is intended to benefit practitioners and stakeholders interested in how remote sensing can be applied to evaluating recovery targets. This work was supported by Section 40804—Ecosystem Restoration of the Infrastructure Investment and Jobs Act of 2021 (Public Law 117–58, 40804).
Mining Background
Numerous regulatory guidelines exist for mining activities and recovery efforts, such as the Surface Mining Control and Reclamation Act (SMCRA) of 1977 (Public Law 95–87; intended for regulating coal mines), the Abandoned Mine Reclamation Act of 1989 (Public Law 101–508, 104 Stat. 1388; amends the Act of 1977, regarding the handling of reclamation fees), and State regulations (some predating the SMCRA of 1977). In general, recovery of mines requires removing unwanted structures and equipment; stabilizing dumps or impoundments; and returning topography, soils, vegetation, and water quality to meet environmental health and safety standards defined by Federal, State, and Tribal regulations. Tribal standards are specified in the Indian Mineral Development Act of 1982 (Public Law 97–382; 25 U.S.C. 2101–2108; Royster, 1994), and Federal and State regulatory resources are provided by the Office of Surface Mining Reclamation and Enforcement (https://www.osmre.gov/laws-and-regulations) and Brown (2023). Surface mines established after 1977 may have baseline ecosystem data to inform recovery targets because of the reporting requirements identified in SMCRA; however, surface mines established before 1977 and nonsurface mines may not have any baseline data. During the permitting stage, SMCRA requires that an adequate description of the existing environmental conditions (such as climate, soils, topography, and vegetation) within and around the proposed mine plan area is documented and cross-sections, maps, and plans illustrating those conditions are developed (30 CFR 779). Knowledge of both preenvironmental and postenvironmental conditions can help inform recovery targets for postmining landscapes. However, the long history of mining activity means much information may be unknown; thus, development of recovery targets may require alternative methods, such as identification of undisturbed sites that reflect conditions before mining activity (hereafter, “reference sites”).
The type of mine and surrounding ecosystem may also affect objectives and complexity of the recovery process. Hardrock mines built underground for extracting rare earth elements (for example, gold and silver) may have unstable structures, cavernous shafts, adits (tunnel entrances), tunnels, tailings, and leftover chemicals and explosives that can pose threats of varying degrees to humans, flora, and fauna. On the other hand, surface mines (such as open pits, strip mining, mountaintop removal, and dredging) require different recovery methods with extensive soil remediation, reclamation, and vegetation recovery. The less common method of solution mining extracts minerals (for example, copper and uranium deposits) by injecting solvents into wells. These solvents can negatively affect groundwater quality and cause surface disturbance from aboveground activities. Examples of recovery goals for different mining types, necessary steps, and the role of vegetation recovery are described in Sidebar 1 (U.S. Environmental Protection Agency, 2000).
Sidebar 1. Goals of the Recovery Process by Mine Type
Hardrock (Underground) Mining Recovery Goals
Safety hazards.—Reduce safety hazards by eliminating access to adits, shafts, exposed stopes, and other features posing a risk to wildlife and humans (for example, fencing and metal grates). These methods are intended to allow for bat roosting within tunnels.
Water and soil properties.—Remediate environmental issues associated with chemicals used during onsite mineral extraction (for example, cyanide was used to extract gold from ore), mitigate leaching of tailing piles into hydrologic systems, and address similar vulnerabilities that affect aquatic health and pose risks to humans, wildlife, and vegetation.
Hydrology.—Reclamation using impoundments to address common flooding problems (for example, water quality and water exiting adits with heavy metals or contaminants) that affect aquatic health and pose risks to humans, wildlife, and vegetation.
Topography.—Reclamation to address subsidence of the surface that can affect surface structures, water drainages, vegetation (for example, can affect the groundwater table), and pose risks to humans, wildlife, and vegetation.
Surface Mining Recovery Goals
Topography.—Return to approximate original contour.
Hydrology.—Monitor surface water and groundwater before, during, and after mining activities. Remediate any contaminants. Postreclamation conditions should reflect characteristics of premining drainage area, stream length, and elevation differences, allowing topography and hydrology to tie into established drainages outside the mine.
Soil properties.—Return soils to premining conditions (for example, soil series, suitability [chemical and physical properties], and depth), salvage overburden soils, and prepare seedbeds (for example, multiple phases of tillage, mulching, and erosion control methods).
Vegetation.—Restore native plants that stabilize soils and can self-regenerate and support succession.
Wildlife—Establish native functioning communities.
Solution Mining Recovery Goals
Water and soil properties—Remediate groundwater affected by introduced solvents and extraction methods. For example, nontarget minerals that unintentionally dissolve can affect groundwater, surface water, and surrounding vegetation.
Vegetation—Restore native plants that stabilize soils and can self-regenerate and support succession.
Remote Sensing Supporting Mine Land Recovery
Although field-based measurements at individual mine sites are critical methods for assessing site conditions and recovery needs, these methods cannot be applied retrospectively to provide insight into previous site conditions and can be time- and cost-prohibitive when applied across large geographic extents. Remote sensing, however, can provide measurements of past and present surface characteristics and can be applied across large geographic extents, often for substantially smaller costs. During the past two decades, the use and availability of remote sensing data have increased considerably, providing numerous methods for evaluating degradation and recovery of mine lands. These data and methods offer a broad spatial and temporal scope (some dating back half a century) that land managers can leverage for site prioritization, recovery design, and long-term assessments of recovery. However, mine lands occur in many ecosystem types characterized by different minerals, geology, hydrology, and vegetation; therefore, the use of remote sensing to address region-wide recovery tasks requires technical expertise and some amount of field data to inform analytical methods and provide evaluation and validation of remote sensing data products.
Remote sensing is the activity of assessing surface characteristics with data acquired by a sensor that is not in direct contact with the surface (Lillesand and others, 2015). The concept behind remote sensing is that different surface materials reflect and absorb different regions of the electromagnetic spectrum (fig. 3), enabling their surface properties to be assessed from a sensor at a distance. Many remote sensing instruments (sensors) are passive and measure electromagnetic radiation (for example, visible light, radio waves, gamma rays, and X-rays) emitted by the sun and reflected from an object’s surface. Conversely, active sensors send pulses of electromagnetic radiation that reflect off a surface and return back to the sensor. Sensors can be ground based, such as instruments mounted on tripods or towers used to measure surface properties of a single plant or a small patch of grass, on aircraft (for example, balloons, remotely piloted aircraft systems, helicopters, or airplanes), or on satellites orbiting the Earth, such as Landsat Operational Land Imager and the Moderate Resolution Imaging Spectroradiometer (MODIS) sensors. Tradeoffs among spectral, temporal, and spatial resolutions (Sidebar 2) generally depend on the type of sensor and platform; for example, sensors that are ground- or aircraft-mounted often have a higher spatial resolution, whereas satellite-based sensors have a lower spatial resolution.
Understanding sensor types and wavelength properties is crucial for selecting appropriate sensors for specified applications. Each remote-sensing platform (ground, airborne, or satellite) may include one or more sensors that capture spectral data as point or gridded surfaces. Biotic and abiotic features, such as dense forests, grasslands, or exposed rocks absorb and reflect differently across the electromagnetic spectrum and exhibit different spectral curves that can be distinguished separately (fig. 3). Passive sensors, such as photographic cameras, spectroradiometers, and passive microwave radiometers, measure emitted and reflected radiation across differing wavelengths and are commonly used to map land cover types and vegetation. Active sensors can often function at night and are less affected by clouds and weather—although some may work in conjunction with passive sensors that require daylight. Sensors detecting microwaves (wavelengths between 1 millimeter [mm] and 1 meter [m]) may be affected less by atmospheric conditions because microwaves more effectively penetrate water droplets than visible wavelengths (380–780 nanometers [nm]) and infrared wavelengths (780 nm–1 mm).
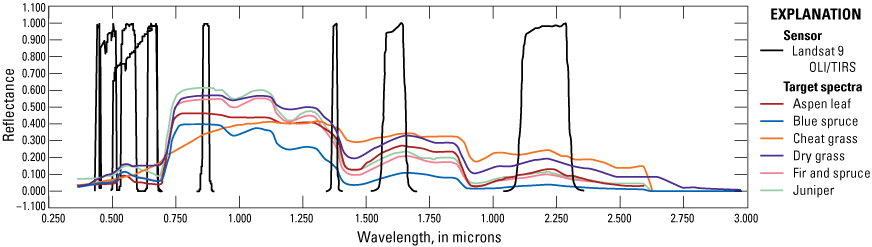
Spectral signatures (continuous measurements of reflectance by means of hyperspectral data) for multiple types of vegetation by wavelength (microns) and corresponding bands (discrete bins of the electromagnetic spectrum) of Landsat 9 operational land imager (OLI) and thermal infrared sensor (TIRS). Modified from U.S. Geological Survey (2023).
Microwave sensors are often used for meteorology (for example, measuring surface winds), oceanography (for example, mapping sea ice and currents), or measuring soil moisture. Multispectral sensors provide spectral bands of varying bandwidths of the visible (380–780 nm), near-infrared (780–1,300 nm), and short to midinfrared (1,300–3,000 nm) ranges, which can help identify vegetation (fig. 3). Hyperspectral imaging captures narrow, contiguous spectral bands with hundreds or thousands of spectra, allowing for significantly greater discrimination of features. Multispectral sensors are commonly used on satellites and hyperspectral sensors are generally mounted on aircraft; therefore, costs vary substantially.
Numerous satellites used for remote sensing have been launched in the past 50 years (for example, Landsat 1 was launched in 1972). The orbits of these satellite platforms and the type of sensors used on any platform determine four types of resolution that should be considered when using remote sensing products—spatial, spectral, temporal, and radiometric (Sidebar 2). Matching study objectives to these four resolutions is important for identifying which satellites and sensors may be most useful for the desired monitoring.
Sidebar 2. Four Resolutions of Remote Sensing
Spatial resolution.—The smallest distance between two objects that can be distinguished by a sensor. Remote sensing imagery obtained at coarse spatial resolutions (greater than 500 m) may only be able to infer broad landscape characteristics such as coarse estimates of vegetation cover, land cover type and extent, snow cover extent, or surface temperature. Remote sensing obtained at very high spatial resolutions (for example, less than 5 m) are more appropriate for measuring properties of vegetation at the patch, stand, or individual plant level. Light Detection and Ranging (lidar), aerial photography, and some multispectral sensors (for example, Planet Labs Rapid Eye Satellite and QuickBird) are examples of high spatial resolution products (less than 30 m). Landsat platforms (30–60 m) are generally considered as medium resolution, and MODIS is considered as coarse or low resolution (500 m). Considerations for using low- compared to high-resolution data includes increased data volume, processing time, and scale of acquired data. Similarly, the higher the spatial resolution, the smaller the footprint (tiles) for data and therefore high spatial resolution products are commonly used for local studies and less so for regional studies.
Spectral resolution.—Spectral resolution represents the range and granularity of wavelength bins that a sensor can capture. Sensors with low spectral resolution collect reflectance values across fewer ranges of wavelengths and are typically noncontiguous. Higher spectral resolution sensors capture reflectance across narrow, contiguous spectral bands with hundreds or thousands of spectra. Multispectral sensors, such as those sensors found on the Landsat 1–9 satellites, collect multiple reflectance values across a range of wavelengths but with fewer bands.
Hyperspectral sensors can measure hundreds of narrow ranges of wavelengths, such that each band may only span 3 nm rather than 60 nm. Multispectral imagery is useful for assessing vegetation communities, land cover types, and similar characteristics, and hyperspectral imagery can identify detailed properties of vegetation such as water stress, nutrient concentration, presence of contaminants, and species identification. Studies that have highlighted differences between multispectral and hyperspectral data may be helpful when designing studies (for example, Gendaram and Damdinsuren, 2021; Jarocińska and others, 2022). The higher the spectral resolution, the easier it is to discern features from the electromagnetic spectrum. The costs of using high compared to low spectral resolution include greater data volumes and the Hughes phenomenon (Hughes, 1968), where more bands require more training data to achieve similar accuracy.
Temporal resolution.—Temporal resolution describes the frequency with which areas are imaged. Satellites with high temporal resolution image the same location more frequently compared to satellites with low temporal resolution. However, a higher temporal resolution results in an increased spatial resolution and larger footprint relative to lower temporal resolution. For example, MODIS returns to the same location once a day, whereas Landsat returns to the same location every 16 days. One benefit of a higher temporal resolution is that cloud cover is easier to mitigate by using multiday image composites.
Radiometric resolution.—Radiometric resolution is the ability of a sensor to discriminate between different values of a signal intensity (in other words, amplitude). Radiometric resolution affects the number of bits that the sensor can record. High radiometric resolution can detect small differences in reflected or emitted energy but may come at the expense of spatial and spectral resolution. Different sensors rely on different radiometric resolution, although generally users pay less attention to radiometric resolution when selecting imagery.
In mining applications, remote sensing provides a method for assessing surface characteristics (such as vegetation type and condition) or soil properties (such as texture, the presence of minerals, or moisture content). Remote sensing is commonly used to evaluate vegetation recovery on mine lands (refer to McKenna and others, 2020) and to map the geographic extent of mining operations and related disturbances (for example, Hao and others, 2019; Gong and others, 2021). For example, remote sensing can monitor changes in the spatial footprint of mining activities and vegetation recovery through time (fig. 4) to document relations between mining activities and vegetation or soil conditions. Residual effects of mining, such as the presence of toxic metals (for example, arsenic, chromium, copper, lead, nickel, and zinc), can also be assessed using a combination of field data and remote sensing imagery (Peng and others, 2016). Remote sensing methods, such as lidar, can also be used to measure changes in topographic relief caused by excavation, deposition, and infilling that was done during mining activities (for example, Banerjee and Raval, 2022). Recent advances in computing capacity and the increased availability of remote sensing data have led to a sharp increase in the use of remote sensing to assess mine lands in the past decade (McKenna and others, 2020).
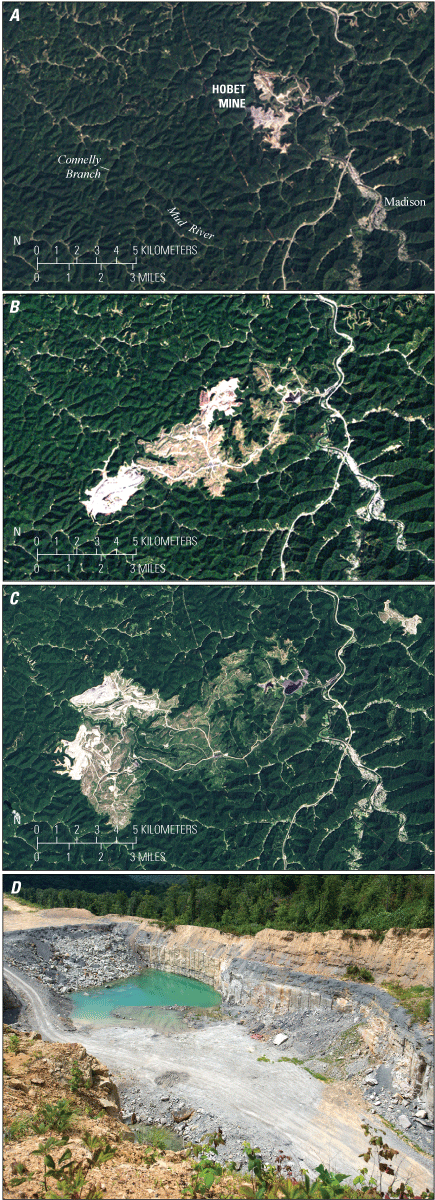
The Hobet-21 coal mine in the Appalachian Mountains of southern West Virginia was one of the State's largest mountaintop removal mines. This figure illustrates how mine footprints can be visible with remote sensing and may change through time as mining activities progress. Revegetation may also be discernable, but identification of vegetation types or community properties may be difficult to assess. Images from Landsat 5, 7, and 8 (A–C) illustrate how the disturbed area of this mine increased to about 10,000 acres in 31 years. A, The Hobet-21 mine in 1984 when the total disturbed area was small [Satellite image by National Aeronautics and Space Administration]. B, By 1998, the Hobet-21 mine had grown westward [Satellite image by National Aeronautics and Space Administration]. C, Mining ended in 2015 when some vegetation recovery was visible from Landsat imagery as light green [Satellite image by National Aeronautics and Space Administration]. D, Photograph of the Hobet-21 mine in 2018 [Photograph by Edwin L. “Bo” Wriston courtesy of West Virginia National Guard]. For additional information, two studies (Wickham and others, 2013; Pericak and others, 2018) used remote sensing to explore the changes and effects of mining activity on the landscape at the Hobet-21 mining site.
Remote sensing alone may not provide enough information to guide recovery efforts, or to thoroughly assess vegetation recovery through time. For example, at sites where the onset of mining activities occurred prior to the availability of remote sensing data, premining conditions are nearly impossible to determine. In nearly all reviewed studies, in situ field surveys were conducted to measure vegetation or soil properties to use in conjunction with remote sensing. Field surveys are important for monitoring vegetation change; for example, one study evaluated 15 mines in southwestern Virginia solely using field surveys and historical vegetation records to define vegetation recovery success (Holl, 2002). However, field studies are usually labor intensive, limiting the scope of their temporal and spatial extents and precluding analyses of long-term or regional trends. Remote sensing offers a method to complement field surveys by providing surface characteristics for unsurveyed periods at low costs and for back-in-time assessments. Thus, researchers frequently pair field data with remote sensing data to train models to characterize mine land conditions in areas other than those areas where field data are collected. For example, field data from a single mine or collected during a single time period can be used to estimate surface conditions on other mine lands or at other time periods. Doing so allows more efficient use of limited, and often expensive, field sampling. Applications of remote sensing also often incorporate ancillary data, such as topographic characteristics or soil type, to provide additional information to characterize mine lands. Combining remote sensing with ancillary data can significantly increase the precision and accuracy of monitoring mine land recovery. Sidebar 3 briefly summarizes key benefits of using remote sensing to assess vegetation recovery.
Sidebar 3. Benefits of Using Remote Sensing to Monitor Vegetation Recovery
• Suitable imagery for assessing vegetation attributes (for example, primary productivity) has been acquired daily since the early 1980s for much of the Earth, enabling remote sensing users to measure vegetation conditions for time periods for which field data may not have been collected. New remote sensing platforms are continually being developed and may provide opportunities to measure vegetation attributes more frequently or with improved capabilities in the future.
• The global coverage of many imagery sources enables analyses to span regions much larger than possible with in situ measurements alone and facilitates data collection in remote areas.
• Certain remote sensing archives (for example, U.S. Geological Survey Landsat, https://www.usgs.gov/landsat-missions/landsat-data-access) offer open access to imagery for all users, thereby reducing the cost of monitoring activities.
• Depending on the imagery source, an abundant amount of information is available to assess recovery success, which may be difficult to obtain from field surveys alone.
• Remote sensing costs may be far less than that of field observations; however, computing and analytical costs may vary depending on the geographic or temporal extent and the complexity of measured vegetation characteristics.
Remotely sensed satellite data are often preprocessed to increase their utility prior to use. Preprocessing is most often done by data providers such as the National Aeronautics and Space Administration (NASA). These providers apply radiometric and geometric corrections to their data to create multiple data processing levels. Radiometric corrections often include the use of calibration coefficients that are applied to raw, unprocessed instrument observations to correct for variations in lighting, sensor angle, atmospheric noise, sensor data storage capacity, and transmission efficiency. Geometric processing may also include the use of calibration coefficients to compensate for geometric distortions across the viewing angle. Preprocessing can also be performed using many different software applications for some platforms, such as the Landsat Ecosystem Disturbance Adaptive Processing System (LEDAPS), which produces top-of-atmosphere reflectance for Landsat (Schmidt and others, 2013). Active sensors, such as lidar and synthetic aperture radar (SAR), require substantial preprocessing using different methods and software applications. For example, point clouds of lidar data can include outliers and generally require classification and editing of errors, downsampling, filtering, transforming, aligning, and other preprocessing. Different methods are also necessary for preprocessing hyperspectral data (for example, Sulaiman and others, 2022).
Analysis-ready imagery products (that is, imagery that has been preprocessed using standard methods) significantly reduce data preparation efforts, but they may not completely eliminate the need for data manipulation prior to analyses. For example, these products often do not address normalization of reflectance between scenes (footprints) or temporal periods, which is crucial for improving precision of results (for example, Canty and others, 2004; Chen and others, 2023). Some preprocessed data products have included errors and may require additional steps to ensure accuracy (for example, Qiu and others, 2019; Teixeira Pinto and others, 2020). Although many off-the-shelf remote sensing data are already preprocessed (for example, Landsat analysis-ready data, https://www.usgs.gov/landsat-missions/landsat-us-analysis-ready-data), preprocessing often remains a necessary step if, for example, (1) multiple scenes are required (for example, Landsat paths and rows), (2) high temporal frequency is required (for example, 30 years of data acquired during peak vegetation productivity [green-up]), (3) multiple data types are required (for example, a mixture of Landsat sensors on platforms 1–9), or (4) lidar and hyperspectral data are used.
Recovery Targets and Performance Indicators
Vegetation recovery is a key component of recovering mine lands to achieve agreed-upon management targets. Operational targets can be used by project managers to guide recovery efforts during or after cessation of mining activities and can range from vague statements such as “return to pre-existing landcover” to detailed statements such as “by 2040, restore 70% of the abundance of native perennial plants found in reference sites” (Young and others, 2022, p. 24). Targets may include restoring structural or functional aspects of vegetation communities, such as landscape connectivity of habitat for key wildlife species (for example, large areas of connected habitats are required for Ursus arctos [grizzly bears]; Proctor and others, 2015) or vegetation structure that is necessary for specific life stages of wildlife (for example, large shrubs and tall grasses for nesting Centrocercus spp. [sage-grouse]; Connelly and others, 2000). Recovery target dates may need to incorporate lag periods for extirpated species to repopulate mine lands after recovery activities have begun. Recovery targets can also specify a geographic extent across which the target is intended to be achieved (for instance, a single site or multiple watersheds).
Remote sensing can be used to monitor progress toward meeting recovery targets. Performance indicators of site condition that can be monitored range from simple descriptors of whether surface vegetation is present to complex indicators describing biological interactions such as herbivory and nutrient cycling (table 1). Indicators can be used to assess incremental changes in vegetation conditions across time, often to assess how well the recovery targets have been met. For example, Han and others (2021) assess recovery efforts by categorizing areas based on evidence for recovery, degradation, degradation–recovery, and no change in conjunction with the Landsat-based detection of Trends in Disturbance and Recovery (LandTrendr) method (Kennedy and others, 2010). Sometimes, categories of recovery success (such as recovery and degradation) may provide more useful measures to land managers than continuous indices of vegetation change (for example, normalized difference vegetation index). Sidebar 4 provides some examples of vegetation change indicators that can be assessed with remote sensing and how these relate to spectral properties.
Sidebar 4. A Subset of Vegetation Change Indicators Assessed with Remote Sensing
Vegetation greenness (multispectral properties).—Multispectral imaging can be used to measure greenness, a dominant vegetation characteristic, by relying on the fundamental principle that chlorophyll in green plant material absorbs red and blue wavelengths of light but reflects near-infrared wavelengths. This principle can be used to create multiple spectral vegetation indices. For example, the Normalized Difference Vegetation Index (NDVI) is capable of distinguishing areas with high densities of vegetation from areas with little or no vegetation (for example, Xue and Su, 2017). These indices can also be used to measure seasonal or cyclic properties of growth and have been used extensively to map and monitor changes in vegetation because they are strongly related to primary productivity and are simple to compute.
Land cover (multispectral properties).—Surface properties such as soil type, vegetation community, and human-made structures are often categorized into land cover types, such as “barren (rock, sand, or clay)”; “deciduous forest”; or “urban” that fall into a predefined land use and land cover system (Anderson and others, 1976). Datasets such as the National Land Cover Database (https://www.mrlc.gov/data, derived from Landsat) provide 16 categories of land cover at a 30-m resolution dating back to the early 2000s. Similarly, the U.S. Landscape Fire and Resource Management Planning Tools (LANDFIRE, https://landfire.gov/) website provides many products for the U.S. products that use Landsat data to describe discrete and continuous data, for example, vegetation (cover, type, and height), fuel loads, and ground disturbance for the years 2001, 2010, 2012, 2014, 2016, and 2020. Identifying categories of vegetation communities is important because such identification allows for assessments of primary productivity per functional group or community, such as pairing the NDVI or a similar index with a vegetation community type (Xue and Su, 2017). The NDVI and other indices only describe spectral properties, so ecological interpretation of such indices improves when the vegetation type and its associated taxa are known.
Plant physiology (hyperspectral properties).—Hyperspectral imaging uses hundreds of spectral bands representing narrow divisions of wavelengths to measure spectral properties beyond the capabilities of multispectral sensors (tens of bands). This type of imaging enables identification of plant physiological characteristics, such as growth stage (for example, Lu and others, 2022), nitrogen uptake (for example, Schlemmer and others, 2013), and disease prevalence (for example, Lowe and others, 2017), and identification of plant species (for example, Ballanti and others, 2016). Hyperspectral sensors are typically used on airborne or ground-based platforms and, as such, will often have exceptionally high spatial- and spectral-resolution with the tradeoff of only being able to image a small area at any given time and at a project-level cost.
Structural properties (lidar and SAR).—Active sensors, such as lidar, use light pulses to measure an object’s distance from the sensor. Structural properties, such as canopy height, density, and biomass, and understory structural characters (for example, height and density), can be estimated for study sites (for example, Bergen and others, 2009). As part of the U.S. Geological Survey’s ongoing National Geospatial Program, the 3D Elevation Program (https://www.usgs.gov/3d-elevation-program) provides preprocessed Level-2 (accuracy of ±3.3 centimeters [cm] in height) lidar point cloud data for most of the continental United States at a single time period after 2016. Publicly available lidar data from satellites are available from the Global Ecosystem Dynamics Investigation program (https://gedi.umd.edu/). Unlike lidar, SAR sensors use radio waves at multiple wavelengths and measure the energy reflected back to the sensor to determine surface structure characteristics. Radio waves with longer wavelengths (for example, 15–100 cm) can penetrate some forest canopy structures and can be used to measure vegetation biomass and canopy structure (for example, Quegan and others, 2019), even in cloudy or poor-weather conditions because they are not impeded by water droplets.
Remote sensing techniques can be used to determine many vegetation indices that are of value for mapping landcover and vegetation characteristics. For example, Xue and Su (2017) provide information on more than 100 vegetation indices. The choice of which index to use often depends on location (for example, soil conditions often affect vegetation indices) and the target recovery objectives. When simple indices are used, remote sensing imagery can quickly provide information about the presence or greenness (productivity) of vegetation. Other indices, however, may require complex methods to identify functional groups or land cover types at a mine site (table 1). For example, simple vegetation indicators, such as NDVI, measure vegetation productivity but are inefficient at measuring species richness or endemism. Li and others (2021) highlight some of the complexities of using NDVI for time-series analyses of vegetation cover, such as contradicting trends in greenness during the temporal period of the assessment. In contrast to NDVI and other vegetation indices that measure vegetation greenness, more complex methods can be used to, for example, model discrete vegetation classes and calculate fractional cover estimates.
Potential gradients of targets can be defined very broadly or finely, which can affect the level of effort and complexity of monitoring efforts (for example, types and frequency of field surveys, remotely sensed hyperspectral or multispectral data accompanied by field surveys). For targets assessing ecosystem function, combining multiple data sources and methods may be necessary. For instance, multispectral imagery can often differentiate among functional vegetative types, but hyperspectral imagery and field surveys are commonly needed to distinguish species (for example, Asner and Martin, 2016). Because of the breadth of approaches used for each objective, we provide only a list of key methods in table 2; however, many of these approaches are mentioned or briefly described in various sections of this report.
Table 1.
Example approaches (performance indicators) for measuring success of vegetation recovery.Table 2.
Example model approaches to produce desired remotely sensed vegetative indicators.[Discrete vegetation classes include land cover, land use, taxa, or community groupings. Continuous estimates of fractional cover include percentage of areal cover of a species canopy within a pixel]
Sometimes recovery targets may reference baseline environmental conditions observed prior to the onset of mining operations. However, baseline vegetation conditions may be unknown, or difficult to obtain for sites where mining activity occurred before SMCRA was enacted in 1977 or the widespread availability of remote sensing imagery. In such situations, additional information may be needed to establish recovery targets. Nearby sites containing undisturbed vegetation are often used as reference sites to define recovery targets (for example, Nauman and Duniway, 2016; Monroe and others, 2022). Reference sites may be selected to reflect natural conditions with similar terrain, soils, climate, vegetation, and wildlife (in other words, ecological potential) that are desired for recovery at a target site. In the United States, a comprehensive set of vegetation reference conditions for terrestrial ecosystems has been developed to describe the dynamics, structure, composition, and disturbance regimes before European-American settlement (Blankenship and others, 2021; Comer and others, 2022). These reference conditions were developed using more than 900 quantitative vegetation dynamic models through LANDFIRE. Reference conditions may also be identified using soil and climate characteristics (Brown, 2010), which can identify recovery targets for vegetation restoration, because soil, climate, topography, and similar abiotic properties affect vegetation recovery rates.
In addition to monitoring the current progress of vegetation recovery, understanding the trajectories of vegetation change can inform expectations for setting realistic recovery targets. For example, remote sensing and statistical analysis of reference site characteristics can estimate the time to recovery based on observed recovery rates within a given ecosystem (Monroe and others, 2022). Understanding the time to recovery under different abiotic conditions, such as differences in fire frequency, or persistence of drought or flooding, may help prioritize restoration by focusing on areas where success is expected or time to recovery is quicker. Conversely, these methods can be used to identify sensitive areas to direct disturbance away from those sites and to establish reasonable expectations.
Many methods exist for modeling vegetation communities and temporal trends using remote sensing data. No single method is likely to work best under all environmental conditions or with all remote sensing data. For example, modeling of fractional vegetation cover has been demonstrated using hyperspectral (Dennison and others, 2019) and multispectral (Gao and others, 2020) remote sensing data, and other methods have combined radiative transfer and dynamic vegetation models (Tu and others, 2020). Methods of vegetation classification using deep learning and machine learning of aerial photogrammetric imagery have also been demonstrated (Drobnjak and others, 2022), indicating that no single best approach exists. Researchers have also highlighted the importance of measuring nonlinear vegetation trends (PolyTrend algorithm) because vegetation changes often follow nonlinear dynamics (Ghorbanian and others, 2022). Selecting the best modeling approach will depend on the desired indicators, data availability, ecosystems, and funding availability. The approach may not necessarily matter if the results inform the recovery targets within the precision and accuracy specified by the recovery targets.
Altogether, methods using remote sensing data can benefit evaluations of progress toward recovery targets and refine monitoring workflows (fig. 5). A combined understanding of the type of mining activity, extent of environmental effects, environmental settings (topography and hydrography), and local ecology will help identify mines that present the greatest risk to humans and ecosystems. Through an iterative process of refining recovery targets and adjusting input data, practitioners can successfully track recovery success (fig. 5B).
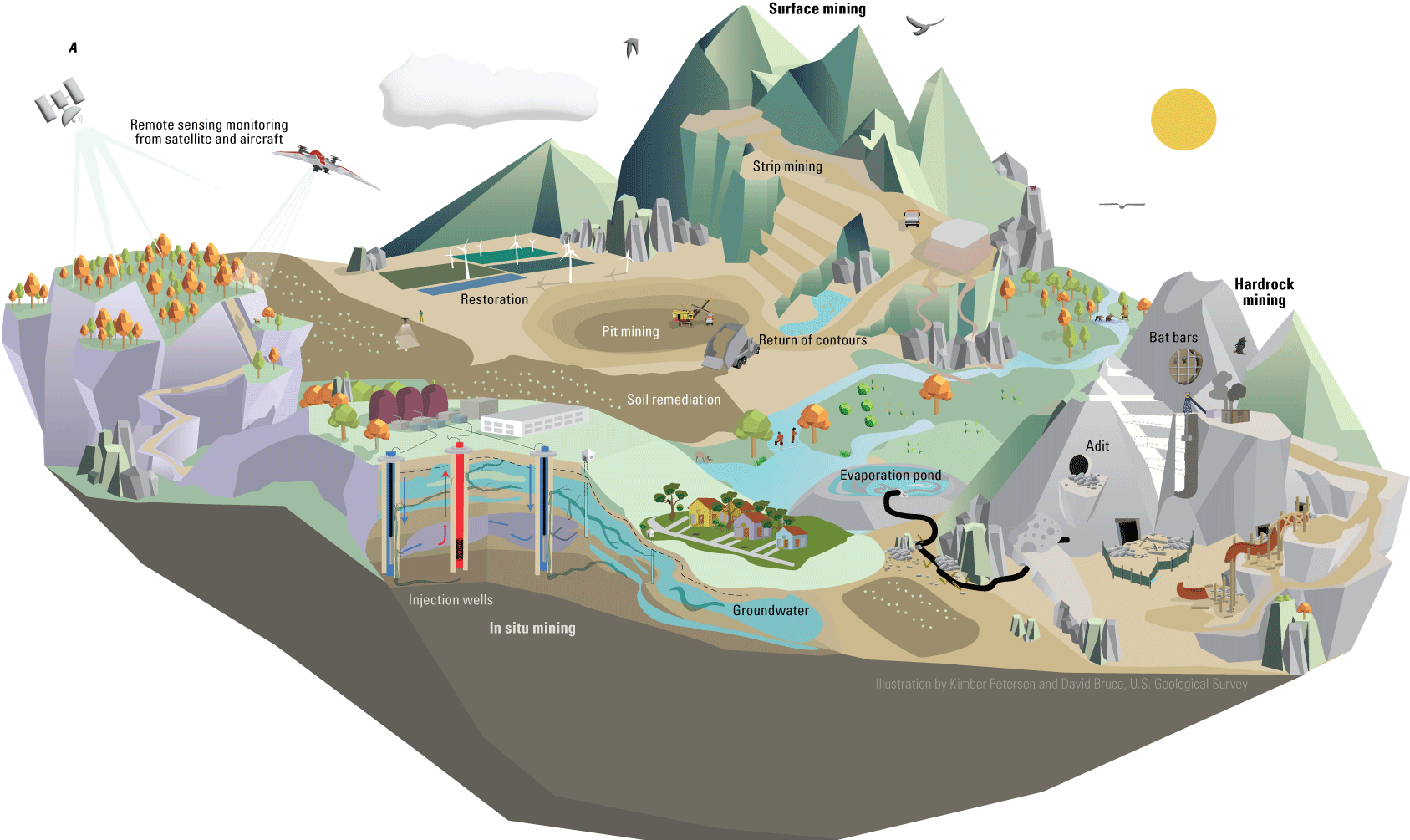
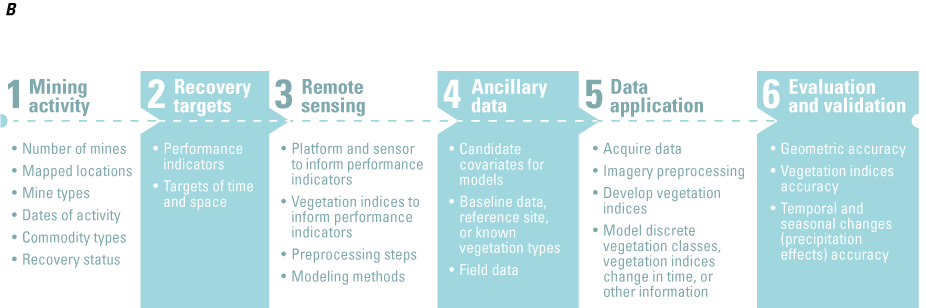
A, The three main mining types and associated hazards (groundwater contamination, potential effects on surface water from runoff, open adits, and open pits), mitigation efforts (bat bars, fences, evaporation ponds, soil remediation), and monitoring methods (groundwater monitoring wells, remote sensing monitoring, field monitoring). [Part A is oversized and is available to view in full screen at https://pubs.usgs.gov/circ/1525/images/cir1525_fig05a.png.] B, An example workflow for prioritizing recovery targets of mine lands using remote sensing, from left to right: (1) identify the mining activity by gathering mine characteristics; (2) select recovery targets and performance indicators; (3) select appropriate remote sensing data, vegetation indices (VIs), and modeling methods to inform performance indicators; (4) select ancillary data to inform models; (5) acquire and apply data (remote and ancillary) to model VIs (for example, discrete vegetation classification, VIs change in time); and (6) evaluate and validate derived VIs and ascertain if methods appropriately evaluate progress towards recovery. Update performance indicators as needed and repeat steps 2–6 until recovery targets are met.
Methods for Literature Review and Examples of Studies Using Remote Sensing for Monitoring Mine Land Recovery
The information provided in this report was informed by a review of recent remote sensing studies on mine lands (app. 1). Specifically, we used the BiblioSearch tool (Kleist and Enns, 2022) to search the Scopus database for recent (from 2005 to 2022) studies of mine lands that used remote sensing to broadly measure recovery success (including reclamation, restoration, rehabilitation, or remediation) or specifically measure vegetation indices. We also used the Google search engine to gain a broader understanding of mine land monitoring and find relevant government articles, regulatory documentation, and general mine land monitoring literature. We found 102 articles describing the use of remote sensing to assess mine land recovery and we retained 85 because of accessibility and peer-review status. We selected a subset (eight case studies) of recently published papers to demonstrate the diversity of platforms and sensors, performance indicators, vegetation indices, and modeling procedures available for evaluating mine land recovery (app. 1). These case studies align with the following topics: multispectral, moderate spatial resolution at multiple sites (case study 1); multispectral, high spatial resolution at a single site (case study 2); multispectral, high spatial resolution at multiple sites (case study 3); multispectral, very high spatial resolution at a single site (case study 4); multispectral, high spatial resolution with radar at a single site (case study 5); multispectral, very high spatial resolution with radar at a single site (case study 6); hyperspectral at a single site (case study 7); and radar only at a single site (case study 8). These studies highlight the diversity of potential approaches for assessing single mine sites, multiple sites, data processing (including preprocessing), postmodeling, and degrees of complexity.
Summary
A variety of remote sensing platforms, sensors, and derived vegetation indices exist to account for variability in spectral reflectance characteristics that differ among ecosystems. These indices, and their use in predictive models, are useful for assessing and monitoring restoration efforts. Successful monitoring and evaluation of mine land recovery requires careful selection of tools that can capture the appropriate indicators of vegetation recovery while also considering the level of effort and expertise needed to use the selected tools. This report highlighted differences in efforts to obtain information about performance indicators, such as vegetation presence or absence and ecosystem function, that are affected by species diversity and biological interactions. For example, determining if vegetation is present for soil stabilization requires significantly less modeling and data than determining if mines have been restored to natural and functioning ecosystems.
Although there are many benefits to using remote sensing for monitoring vegetation recovery, there are also numerous constraints to consider. For example, many remotely sensed indices may be available, but they cannot always be extrapolated to areas with dissimilar spectral reflectances. Often indices must be modified for different ecosystems and may require ancillary inputs and modeling, especially when evaluating mines across time and space. When temporal and geographic extents are large, substantial computing resources and modeling expertise may be required. Specifically, environmental context becomes a critical consideration when evaluating multiple mines in different areas because different abiotic and biotic conditions add complexity when assessing progress toward recovery targets. For example, abiotic conditions such as precipitation affect spectral signatures of soil and vegetation (near infrared reflects more strongly from vegetation with high water content). Substantial preprocessing of remote sensing imagery may be required, especially when long time periods or large spatial extents are considered. Additionally, as advances in remote sensing continue to evolve and more data become available at finer spatial and spectral resolutions, computational costs may also increase. A balance between spatial and spectral resolutions may be advantageous depending on the objective.
The breadth of knowledge captured in the literature searches, and the case studies highlighted here, provide examples of how remote sensing data can be used for monitoring mine lands. Although field observations are often needed, they can be cost- and labor-intensive to acquire. Remote sensing platforms offer another approach for collecting data across longer time periods and larger areas with less effort that can often be used to augment and improve assessments of mine recovery. For example, active sensors like Light Detection and Ranging (lidar) fitted to aircraft or satellites can collect data on topography and vegetation structure (for example, to assess height or biomass), and may be able to help discern plant communities or individual plant species across large swaths of land. The possibilities of remote sensing to monitor mine lands appear endless, and carefully considering recovery objectives, mine type, and ecosystem will help users determine the best methods to use to gain the most benefit. Overall, applications of remote sensing to mine recovery will be most effective when recovery targets are clearly defined and quantifiable from data collected before mining activity. Additionally, these collected data would need to be accessible and maintained in databases for baseline references used to establish the recovery targets.
References Cited
Anderson, J.R., Hardy, E.E., Roach, J.T., and Witmer, R.E., 1976, A land use and land cover classification system for use with remote sensor data: U.S Geological Survey Professional Paper 964, 34 p., accessed April 22, 2023, at https://pubs.er.usgs.gov/publication/pp964.
Asner, G.P., and Martin, R.E., 2016, Spectranomics—Emerging science and conservation opportunities at the interface of biodiversity and remote sensing: Global Ecology and Conservation, v. 8, p. 212–219, accessed April 22, 2023, at https://doi.org/10.1016/j.gecco.2016.09.010.
Ballanti, L., Blesius, L., Hines, E., and Kruse, B., 2016, Tree species classification using hyperspectral imagery—A comparison of two classifiers: Remote Sensing, v. 8, no. 6, 18 p., accessed April 22, 2023, at https://doi.org/10.3390/rs8060445.
Banerjee, B.P., and Raval, S., 2022, Mapping sensitive vegetation communities in mining eco-space using UAV-lidar: International Journal of Coal Science & Technology, v. 9, 16 p., accessed April 22, 2023, at https://doi.org/10.1007/s40789-022-00509-w.
Bergen, K.M., Goetz, S.J., Dubayah, R.O., Henebry, G.M., Hunsaker, C.T., Imhoff, M.L., Nelson, R.F., Parker, G.G., and Radeloff, V.C., 2009, Remote sensing of vegetation 3-D structure for biodiversity and habitat—Review and implications for lidar and radar spaceborne missions: Journal of Geophysical Research—Biogeosciences, v. 114, no. G2, 13 p., accessed April 22, 2023, at https://doi.org/10.1029/2008JG000883.
Blankenship, K., Swaty, R., Hall, K.R., Hagen, S., Pohl, K., Shlisky Hunt, A., Patton, J., Frid, L., and Smith, J., 2021, Vegetation dynamics models—A comprehensive set for natural resource assessment and planning in the United States: Ecosphere, v. 12, no. 4, 22 p., accessed April 22, 2023, at https://doi.org/10.1002/ecs2.3484.
Brown, J.R., 2010, Ecological sites—Their history, status, and future: Rangelands, v. 32, no. 6, p. 5–8, accessed April 22, 2023, at https://doi.org/10.2111/Rangelands-D-10-00089.1.
Brown, M., 2023, USA, in Dewar, J., ed., Mining laws and regulations 2024—International comparative legal guides: London, Global Legal Group, p. 134–143, accessed April 22, 2023, at https://iclg.com/practice-areas/mining-laws-and-regulations.
Canty, M.J., Nielsen, A.A., and Schmidt, M., 2004, Automatic radiometric normalization of multitemporal satellite imagery: Remote Sensing of Environment, v. 91, no. 3-4, p. 441–451, accessed April 22, 2023, at https://doi.org/10.1016/j.rse.2003.10.024.
Chen, L., Ma, Y., Lian, Y., Zhang, H., Yu, Y., and Lin, Y., 2023, Radiometric normalization using a pseudo-invariant polygon features-based algorithm with contemporaneous Sentinel−2A and Landsat−8 OLI imagery: Applied Sciences, v. 13, no. 4, 16 p., accessed April 22, 2023, at https://doi.org/10.3390/app13042525.
Comer, P.J., Hak, J.C., Dockter, D., and Smith, J., 2022, Integration of vegetation classification with land cover mapping—Lessons from regional mapping efforts in the Americas: Vegetation Classification and Survey, v. 3, p. 29–43, accessed April 22, 2023, at https://doi.org/10.3897/VCS.67537.
Connelly, J.W., Schroeder, M.A., Sands, A.R., and Braun, C.E., 2000, Guidelines to manage sage grouse populations and their habitats: Wildlife Society Bulletin, v. 28, no. 4, p. 967–985, accessed April 22, 2023, at https://www.jstor.org/stable/3783856.
Dennison, P.E., Qi, Y., Meerdink, S.K., Kokaly, R.F., Thompson, D.R., Daughtry, C.S.T., Quemada, M., Roberts, D.A., Gader, P.D., Wetherley, E.B., Numata, I., and Roth, K.L., 2019, Comparison of methods for modeling fractional cover using simulated satellite hyperspectral imager spectra: Remote Sensing, v. 11, no. 18, 23 p., accessed April 22, 2023, at https://doi.org/10.3390/rs11182072.
Drobnjak, S., Stojanović, M., Djordjević, D., Bakrač, S., Jovanović, J., and Djordjević, A., 2022, Testing a new ensemble vegetation classification method based on deep learning and machine learning methods using aerial photogrammetric images: Frontiers in Environmental Science, v. 10, 14 p., accessed April 22, 2023, at https://doi.org/10.3389/fenvs.2022.896158.
Gao, L., Wang, X., Johnson, B.A., Tian, Q., Wang, Y., Verrelst, J., Mu, X., and Gu, X., 2020, Remote sensing algorithms for estimation of fractional vegetation cover using pure vegetation index values—A review: ISPRS Journal of Photogrammetry and Remote Sensing, v. 159, p. 364–377, accessed April 22, 2023, at https://doi.org/10.1016/j.isprsjprs.2019.11.018.
Gendaram, O., and Damdinsuren, A., 2021, Comparison of spectral signatures in hyperspectral and multispectral data, in Environmental Science and Technology International Conference (ESTIC 2021)—International Conference on Environmental Science and Technology, 2021, Ulaanbaatar, Mongolia, September 23, 2021, Proceedings: Atlantis Press International B.V., p. 116–120.
Ghorbanian, A., Mohammadzadeh, A., and Jamali, S., 2022, Linear and non-linear vegetation trend analysis throughout Iran using two decades of MODIS NDVI imagery: Remote Sensing, v. 14, no. 15, 23 p., accessed April 22, 2023, at https://doi.org/10.3390/rs14153683.
Gong, B., Shu, C., Han, S., and Cheng, S.G., 2021, Mine vegetation identification via ecological monitoring and deep belief network: Plants (Basel), v. 10, no. 6, 22 p., accessed April 22, 2023, at https://doi.org/10.3390/plants10061099.
Han, Y., Ke, Y., Zhu, L., Feng, H., Zhang, Q., Sun, Z., and Zhu, L., 2021, Tracking vegetation degradation and recovery in multiple mining areas in Beijing, China, based on time-series Landsat imagery: GIScience & Remote Sensing, v. 58, no. 8, p. 1477–1496, accessed April 22, 2023, at https://doi.org/10.1080/15481603.2021.1996319.
Hao, L., Zhang, Z., and Yang, X., 2019, Mine tailing extraction indexes and model using remote-sensing images in southeast Hubei Province: Environmental Earth Sciences, v. 78, no. 15, 11 p., accessed April 22, 2023, at https://doi.org/10.1007/s12665-019-8439-1.
Holl, K.D., 2002, Long-term vegetation recovery on reclaimed coal surface mines in the eastern USA: Journal of Applied Ecology, v. 39, p. 960–970, accessed April 22, 2023, at https://doi.org/10.1046/j.1365-2664.2002.00767.x.
Horton, J.D., and San Juan, C.A., 2016, Prospect- and mine-related features from U.S. Geological Survey 7.5- and 15-minute topographic quadrangle maps of the United States (ver. 10.0, May 2023): U.S. Geological Survey data release, accessed April 22, 2023, at https://doi.org/10.5066/F78W3CHG.
Hughes, G., 1968, On the mean accuracy of statistical pattern recognizers: IEEE Transactions on Information Theory, v. 14, no. 1, p. 55–63, accessed April 22, 2023, at https://doi.org/10.1109/TIT.1968.1054102.
Jarocińska, A., Kopeć, D., Kycko, M., Piórkowski, H., and Błońska, A., 2022, Hyperspectral vs. multispectral data—Comparison of the spectral differentiation capabilities of Natura 2000 non-forest habitats: ISPRS Journal of Photogrammetry and Remote Sensing, v. 184, p. 148–164, accessed April 22, 2023, at https://doi.org/10.1016/j.isprsjprs.2021.12.010.
Kennedy, R.E., Yang, Z., and Cohen, W.B., 2010, Detecting trends in forest disturbance and recovery using yearly Landsat time series—1. LandTrendr—Temporal segmentation algorithms: Remote Sensing of Environment, v. 114, no. 12, p. 2897–2910, accessed April 22, 2023, at https://doi.org/10.1016/j.rse.2010.07.008.
Kleist, N.J., and Enns, K.D., 2022, USGS BiblioSearch (ver. 1.0.0, December 2021): U.S. Geological Survey software release, accessed April 22, 2023, at https://doi.org/10.5066/P9EW8BO5.
Li, S., Xu, L., Jing, Y., Yin, H., Li, X., and Guan, X., 2021, High-quality vegetation index product generation—A review of NDVI time series reconstruction techniques: International Journal of Applied Earth Observation and Geoinformation, v. 105, 18 p., accessed April 22, 2023, at https://doi.org/10.1016/j.jag.2021.102640.
Lima, A.T., Mitchell, K., O’Connell, D.W., Verhoeven, J., and Van Cappellen, P., 2016, The legacy of surface mining—Remediation, restoration, reclamation and rehabilitation: Environmental Science & Policy, v. 66, p. 227–233, accessed April 22, 2023, at https://doi.org/10.1016/j.envsci.2016.07.011.
Lowe, A., Harrison, N., and French, A.P., 2017, Hyperspectral image analysis techniques for the detection and classification of the early onset of plant disease and stress: Plant Methods, v. 13, 12 p., accessed April 22, 2023, at https://doi.org/10.1186/s13007-017-0233-z.
Lu, W., Okayama, T., and Komatsuzaki, M., 2022, Rice height monitoring between different estimation models using UAV photogrammetry and multispectral technology: Remote Sensing, v. 14, no. 1, 24 p., accessed April 22, 2023, at https://doi.org/10.3390/rs14010078.
McKenna, P.B., Lechner, A.M., Phinn, S., and Erskine, P.D., 2020, Remote sensing of mine site rehabilitation for ecological outcomes—A global systematic review: Remote Sensing, v. 12, no. 21, 34 p., accessed April 22, 2023, at https://doi.org/10.3390/rs12213535.
Monroe, A.P., Nauman, T.W., Aldridge, C.L., O’Donnell, M.S., Duniway, M.C., Cade, B.S., Manier, D.J., and Anderson, P.J., 2022, Assessing vegetation recovery from energy development using a dynamic reference approach: Ecology and Evolution, v. 12, no. 2, 22 p., accessed April 22, 2023, at https://doi.org/10.1002/ece3.8508.
Nauman, T.W., and Duniway, M.C., 2016, The automated reference toolset—A soil-geomorphic ecological potential matching algorithm: Soil Science Society of America Journal, v. 80, no. 5, p. 1317–1328, accessed April 22, 2023, at https://doi.org/10.2136/sssaj2016.05.0151.
Peng, Y., Kheir, R.B., Adhikari, K., Malinowski, R., Greve, M.B., Knadel, M., and Greve, M.H., 2016, Digital mapping of toxic metals in Qatari soils using remote sensing and ancillary data: Remote Sensing, v. 8, no. 12, 19 p., accessed April 22, 2023, at https://doi.org/10.3390/rs8121003.
Pericak, A.A., Thomas, C.J., Kroodsma, D.A., Wasson, M.F., Ross, M.R.V., Clinton, N.E., Campagna, D.J., Franklin, Y., Bernhardt, E.S., and Amos, J.F., 2018, Mapping the yearly extent of surface coal mining in Central Appalachia using Landsat and Google Earth Engine: PLoS One, v. 13, no. 7, 15 p., accessed April 22, 2023, at https://doi.org/10.1371/journal.pone.0197758.
Proctor, M.F., Nielsen, S.E., Kasworm, W.F., Servheen, C., Radandt, T.G., Machutchon, A.G., and Boyce, M.S., 2015, Grizzly bear connectivity mapping in the Canada–United States trans-border region: The Journal of Wildlife Management, v. 79, no. 4, p. 544–558, accessed April 22, 2023, at https://doi.org/10.1002/jwmg.862.
Qiu, S., Lin, Y., Shang, R., Zhang, J., Ma, L., and Zhu, Z., 2019, Making Landsat time series consistent—Evaluating and improving Landsat analysis ready data: Remote Sensing, v. 11, no. 1, 21 p., accessed April 22, 2023, at https://doi.org/10.3390/rs11010051.
Quegan, S., Le Toan, T., Chave, J., Dall, J., Exbrayat, J.-F., Minh, D.H.T., Lomas, M., D'Alessandro, M.M., Paillou, P., Papathanassiou, K., Rocca, F., Saatchi, S., Scipal, K., Shugart, H., Smallman, T.L., Soja, M.J., Tebaldini, S., Ulander, L., Villard, L., and Williams, M., 2019, The European Space Agency BIOMASS mission—Measuring forest above-ground biomass from space: Remote Sensing of Environment, v. 227, p. 44–60, accessed April 22, 2023, at https://doi.org/10.1016/j.rse.2019.03.032.
Royster, J.V., 1994, Mineral development in Indian country—The evolution of Tribal control over mineral resources: Tulsa Law Review, v. 29, no. 3, p. 541–637, accessed April 22, 2023, at https://digitalcommons.law.utulsa.edu/tlr/vol29/iss3/3.
Schlemmer, M., Gitelson, A., Schepers, J., Ferguson, R., Peng, Y., Shanahan, J., and Rundquist, D., 2013, Remote estimation of nitrogen and chlorophyll contents in maize at leaf and canopy levels: International Journal of Applied Earth Observation and Geoinformation, v. 25, p. 47–54, accessed April 22, 2023, at https://doi.org/10.1016/j.jag.2013.04.003.
Schmidt, G.L., Jenkerson, C.B., Masek, J., Vermote, E., and Gao, F., 2013, Landsat ecosystem disturbance adaptive processing system (LEDAPS) algorithm description: U.S. Geological Survey Open-File Report 2013–1057, 17 p., accessed April 22, 2023, at https://doi.org/10.3133/ofr20131057.
Sulaiman, N., Che’Ya, N.N., Roslim, M.H.M., Juraimi, A.S., Noor, N.M., and Ilahi, W.F.F., 2022, The application of hyperspectral remote sensing imagery (HRSI) for weed detection analysis in rice fields—A review: Applied Sciences, v. 12, no. 5, 19 p., accessed April 22, 2023, at https://doi.org/10.3390/app12052570.
Teixeira Pinto, C., Jing, X., and Leigh, L., 2020, Evaluation analysis of Landsat level-1 and level-2 data products using in situ measurements: Remote Sensing, v. 12, no. 16, 27 p., accessed April 22, 2023, at https://doi.org/10.3390/rs12162597.
Tu, Y., Jia, K., Liang, S., Wei, X., Yao, Y., and Zhang, X., 2020, Fractional vegetation cover estimation in heterogeneous areas by combining a radiative transfer model and a dynamic vegetation model: International Journal of Digital Earth, v. 13, no. 4, p. 487–503, https://doi.org/10.1080/17538947.2018.1531438.
U.S. Environmental Protection Agency, 2000, Abandoned mine site characterization and cleanup handbook: U.S. Environmental Protection Agency Region 8 EPA 910-B-00-001, 129 p., accessed April 22, 2023, at https://www.epa.gov/sites/default/files/2015-09/documents/2000_08_pdfs_amscch.pdf.
U.S. Geological Survey, 2023, Spectral characteristics viewer: U.S. Geological Survey website, accessed December 22, 2022, at https://landsat.usgs.gov/spectral-characteristics-viewer.
Wickham, J., Wood, P.B., Nicholson, M.C., Jenkins, W., Druckenbrod, D., Suter, G.W., Strager, M.P., Mazzarella, C., Galloway, W., and Amos, J., 2013, The overlooked terrestrial impacts of mountaintop mining: BioScience, v. 63, no. 5, p. 335–348, accessed April 22, 2023, at https://doi.org/10.1525/bio.2013.63.5.7.
Xue, J., and Su, B., 2017, Significant remote sensing vegetation indices—A review of developments and applications: Journal of Sensors, v. 2017, 17 p., accessed April 22, 2023, at https://doi.org/10.1155/2017/1353691.
Young, R.E., Gann, G.D., Walder, B., Liu, J., Cui, W., Newton, V., Nelson, C.R., Tashe, N., Jasper, D., Silveira, F.A.O., Carrick, P.J., Hägglund, T., Carlsén, S., and Dixon, K., 2022, International principles and standards for the ecological restoration and recovery of mine sites: Restoration Ecology Special Issue, v. 30, no. S2, 51 p., accessed April 22, 2023, at https://doi.org/10.1111/rec.13771.
Glossary
adit
Horizontal entrance to a mine.
computing capacity
The storage and transaction processing capability of computer systems.
deposition
Geological process that adds soil, sediments, and (or) rocks to a landform or landmass.
downsampling
Process to reduce the number of samples in a dataset.
dredging
Removing material from a water environment.
electromagnetic radiation
Energy transfer in the form of the electric and magnetic fields that make up electromagnetic waves such as radio waves, visible light, and gamma rays.
endemism
A condition in which a species is restricted to a single area.
functional groups
A group of species that share similar characteristics within a community.
functional vegetative types
A group of vegetation types that share similar characteristics within a community.
gamma rays
Type of electromagnetic radiation with wavelengths generally smaller than 10−10 meters.
geometric correction
Data manipulation that improves the spatial accuracy of the spectral observations by removing or reducing the effects of viewing angle and altitude.
hardrock mine
An excavation in the earth to remove “hard” minerals or metals such as gold, copper, or tin.
in situ measurements
Measurements collected in the field.
land cover type
Categories of physical materials that cover the Earth’s surface, for example, trees, shrubs, or wetland.
microwave
Type of electromagnetic radiation with wavelengths generally ranging from 30 centimeters to 1 millimeter.
mountaintop removal
A surface mining practice that alters topography and commonly removes a summit, hill, or ridge to access coal.
mulching
Adding a protective layer of material to the top of soil.
Normalized Difference Vegetation Index (NDVI)
A measurement of vegetation greenness, which is calculated as the ratio between the red and near-infrared values of the light spectrum that are reflected by land surfaces.
ore
Natural rock or sediment that contains valuable metals or minerals.
overburden soils
Soils that are mechanically displaced and unprocessed and that overly material that will be extracted.
platforms
The structures on which remote sensing instruments are mounted, which can be terrestrial, airborne, or space based.
primary productivity
The rate at which energy is converted to organic substances by photosynthetic producers (obtain energy and nutrients from sunlight) and chemosynthetic producers (obtain energy through oxidation).
radio waves
Type of electromagnetic radiation with wavelengths generally greater than 1 millimeter.
radiometer
A device that detects and measures electromagnetic radiation.
radiometric correction
Data manipulation that improves the accuracy of the spectral observations by removing or reducing the effects of atmospheric, sensor, and illumination factors.
reclamation
The geotechnical stabilization of land (for example, soils and topography), allowing for the return of flora and fauna or species that are endemic to the area but not necessarily the same composition or exact condition as predisturbance.
rehabilitation
Activities focused on reinstating ecosystem services that benefit human communities and livelihoods (such as farming or development).
remediation
Activities focused on removing contaminants from the environment (soils, water, air) and managing risks to humans and wildlife.
restoration
Actions resulting in the recovery of an ecosystem to its original state prior to disturbance.
seedbed
Soil prepared for planting seeds.
shaft
A long, often vertical, passage that gives access or ventilation to an underground mine.
soil
The uppermost layer of the Earth’s crust.
stope
Excavation of ground in layers or steps.
strip mining
Type of surface mining that removes a long line of soil or rock above a layer of valuable material.
tailings
Soil or rock left over after separating out the valuable material.
target
Desired goal or outcome.
tillage
To prepare soil for planting by digging, stirring, and (or) overturning soil using mechanical processes.
topography
The configuration of the Earth’s surface.
topographic relief
The difference in elevation between the lowest and highest points of the surface.
X-ray
Type of electromagnetic radiation with wavelengths generally ranging from 10−8 to 10−12 meters.
Appendix 1. Eight Example Studies and Literature Review References
Case Study 1—Multispectral, Moderate Spatial Resolution at Multiple Sites
Title.—Mines to forests? Analyzing long-term recovery trends for surface coal mines in Central Appalachia (Thomas and others, 2023)
Subject.—Vegetation trends and rate of recovery of mines using mountaintop removal in forests of Central Appalachia in the United States. Used footprints of 71,140 mines in West Virginia, Tennessee, Virginia, and Kentucky.
Platforms, sensors, or both.—Landsat.
Preprocessing.—Annual Landsat imagery spanning the years 1985–2015 were filtered to the growing season period from April through October. Landsat data were harmonized to account for differences in band wavelengths between different Landsat satellites.
Performance indicators, vegetation indicators, or both.—Normalized burn ratio, normalized difference moisture index, and Normalized Difference Vegetation Index (NDVI).
Modeling procedures.—Authors used Google Earth Engine (GEE) to compile imagery and measure vegetation trends. To compare vegetation trends, the authors visually selected 5 reference sites for each of the 24 U.S. Environmental Protection Agency Level 4 Ecoregions found within the study area. These reference sites provided a baseline to compare undisturbed vegetation trends to vegetation trends on disturbed mine footprints. After estimating each index, the authors estimated the rate of recovery and long-term mean conditions based on first-order autoregressive models (“rstanarm” package in program R, https://mc-stan.org/rstanarm/). Long-term mean conditions were used to quantify the equilibrium of each site, which could be compared to surrounding reference sites to estimate the percent recovery of each site.
Results.—The study’s results indicate that, although mines in the study area recovered, such sites are still well below the conditions of surrounding reference forests as only 0.10 percent of sites have a 95-percent probability of reaching or exceeding the conditions of reference forests, based on the three vegetation indices. Recovery rates were not affected by the area, slope, or elevation of, or precipitation at the mine. Although this study only used three vegetation indices, the authors compiled other indices (Enhanced Vegetation Index [EVI], Soil-Adjusted Vegetation Index [SAVI], and modified SAVI), all of which are available from their publicly available dataset (Thomas and others, 2022).
Case Study 2—Multispectral, High Spatial Resolution at a Single Site
Title.—Multi-year mapping of disturbance and reclamation patterns over Tronox’s Hillendale mine, South Africa with DBEST and Google Earth Engine (Xulu and others, 2021)
Subject.—Detection of changes at a zirconium and titanium mine in a subtropical to tropical coastal area of South Africa.
Platforms, sensors, or both.—Landsat (Landsat 5, 7, and 8 images [Level-1 precision terrain-corrected]).
Preprocessing.—Landsat images (from 2001 to 2019) were filtered and cloud masked.
Performance indicators, vegetation indicators, or both.—Trend analysis of changes in NDVI.
Modeling procedures.—The authors used GEE to access Landsat imagery and calculate NDVI. The Detecting Breakpoints and Estimating Segments in Trend (DBEST; Jamali and others, 2015) program was used for segmenting and analyzing trend changes in the time series of NDVI (from 2001 to 2019). They used spline interpolation to fit a cubic spline to each missing NDVI value resulting from cloud masking. The DBEST approach is believed to handle discontinuous time-series data better than other more widely applied methods such as Landsat-based detection of Trends in Disturbance and Recovery (LandTrendr; Kennedy and others, 2010). The algorithm within DBEST has two parts. The first detects trends in the time-series data; determines the behavior of the change (abrupt or gradual); and estimates the timing, magnitude, number, and direction of change. The second part of the algorithm then simplifies the change features into an overall main trend.
Results.—The authors found that DBEST could estimate the breaking points of NDVI values through time and that the breaking points aligned well with the known dates of disturbance and rehabilitation at their mine site of interest. The authors caution against using trends in NDVI values alone to determine rehabilitation success without evaluating the vegetation type associated with the values because NDVI does not distinguish between native or invasive species. They used random forests to classify vegetated and nonvegetated areas (78 percent overall accuracy) to evaluate the results where they observed recovery after mining stopped in 2013. This study provides an example of an affordable approach to tracking vegetation change by leveraging publicly available Landsat data and the high computing power of the GEE platform.
Case Study 3—Multispectral, High Spatial Resolution at Multiple Sites
Title.—Mining and restoration monitoring of rare earth element (REE) exploitation by new remote sensing indicators in Southern Jiangxi, China (Xie and others, 2020)
Subject.—Detection of changes at open-pit mines that extract REE in a subtropical (forested) monsoon ecosystem in southern Jiangxi, China and used footprints of more than 1,281 mines.
Platforms, sensors, or both.—Landsat Thematic Mapper (TM), Enhanced Thematic Mapper Plus (ETM+) and Operational Land Imager (OLI) images were used to develop indices. Mine boundaries were defined using high resolution imagery, such as QuickBird, GeoEye, and SPOT, available on GEE.
Preprocessing.—Preprocessing of Landsat imagery (from 1988 to 2019) included selecting cloud-free dates, atmospheric correction, and dark object subtraction (temporal normalization technique).
Performance indicators, vegetation indicators, or both.—The authors used Landsat imagery (from 1988 to 2019) to develop mining and restoration assessment indicators (MRAIs) based on spectral reflectance, albedo, land-surface temperature, and tasseled cap brightness. The authors compared their index to more common indices, including NDVI, EVI, SAVI, and Generalized Difference Vegetation Index (GDVI) at six typical open-pit mines at varying restoration stages.
Modeling procedures.—Landsat images were obtained from the U.S. Geological Survey Global Visualization Viewer (https://glovis.usgs.gov). High-resolution imagery (for example, Quickbird, GeoEye, and SPOT) was processed on GEE to define mining boundaries. Indices were compared by measuring the relative sensitivity of MRAI to each standard vegetation index (NDVI, EVI, SAVI, and GDVI). When MRAI sensitivity is greater than one, MRAI is more sensitive than the standard vegetation index (VI), when it is equal to one, the indices have the same sensitivity; and when it is less than one, MRAI is less sensitive than the standard VI.
Results.—The authors found MRAIs provided greater sensitivity and were more informative than more common indices (NDVI, EVI, SAVI, and GDVI) at six typical REE open-pit mines. This study demonstrates the challenges of using standard VIs to assess revegetation success of numerous mining sites (n=1,281), resulting in a new index (MRAI) that could better inform monitoring for sites with heterogeneous revegetation stages.
Case Study 4—Multispectral, Very High Spatial Resolution at a Single Site
Title.—Mining land subsidence monitoring using Sentinel-1 SAR data (Yuan and others, 2017)
Subject.—Detection of surface change caused by underground mines (coal mining) for midlatitude steppe climate in Yuanbaoshan District, Chifeng City, China.
Platforms, sensors, or both.—Differential Interferometry Synthetic Aperture Radar (DInSAR) of the Sentinel-1 satellite
Preprocessing.—Preprocessing procedures were not explained.
Performance indicators, vegetation indicators, or both.—Surface deformation (from 2015 to 2016).
Modeling procedures.—Interferometry, or InSAR, uses the phase information recorded by synthetic aperture radar (SAR) to measure the distance from the sensor to the Earth’s ground to assess terrain. By comparing two InSAR measurements of the same area taken at different times, a methodology called DInSAR, surface deformation patterns can be accessed. Yuan and others (2017) used DInSAR techniques to generate interferograms for pairs (phase information) of short time intervals and small spatial perpendicular baseline images to achieve smaller spectral offsets to evaluate deformation.
Results.—The effects of subsidence were detected in mining areas and surrounding urban areas using DInSAR. The methods used in this study demonstrate how remote sensing using radar can provide insight into the effect of underground mining, which might not otherwise be discernable using other remote sensing methods.
Case Study 5—Multispectral, High Spatial Resolution with Radar at a Single Site
Title.—Mountaintop mining legacies constrain ecological, hydrological and biogeochemical recovery trajectories (Ross and others, 2021)
Subject.—Reference site characterization of mines using mountaintop removal in forests of West Virginia, United States
Platforms, sensors, or both.—Landsat and aerial Light Detection and Ranging (lidar) sensor.
Preprocessing.—Landsat data required the standard preprocessing and harmonization (temporal normalization) of the data before generating NDVI from 1984 to 2019. The study’s area of interest had previously been processed and had available lidar data.
Performance indicators, vegetation indicators, or both.—The authors used Landsat-derived NDVI and aerial lidar-derived canopy height data to map vegetation cover and structural change from 1984 to 2019. The authors also collected field data to link remotely sensed vegetation change with on-the-ground hydrologic and biogeochemical effects of mining.
Modeling procedures.—The authors compared mine land recovery (measured by NDVI and canopy height) to reference forests and forests that were disturbed but not in their mining dataset or within mining permit boundaries.
Results.—Since 1977, the United States requires mined areas to be restored to equal or greater value than the ones they replace. However, this study found that vegetation at sites that were previously mined were shorter and sparser than vegetation at reference sites because of substantial changes to the environment's topography, hydrology (water moves more slowly through exploded bedrock), and soil chemistry.
Case Study 6—Multispectral, Very High Spatial Resolution with Radar at a Single Site
Title.—Land cover changes in open-cast mining complexes based on high-resolution remote sensing data (Nascimento and others, 2020)
Subject.—Change detection, disturbance mapping, and land cover classification for open-cast (also called open-pit) iron ore mines in tropical forests and savanna ecosystems in the eastern Amazon Basin in Brazil.
Platforms, sensors, or both.—GeoEye, WorldView-3, IKONOS, and lidar.
Preprocessing.—Preprocessing of optical data (from 2011 to 2015) included atmospheric correction, resampling, orthorectification, mosaicing, and creation of vegetation indices. Processing of lidar data included ground filtering before creating elevation and slope products.
Performance indicators, vegetation indicators, or both.—This study used spectral indices to create NDVI and normalized difference water index from high-resolution commercial satellite imagery. An aerial lidar sensor was flown in 2012 to derive elevation and slope characteristics.
Modeling procedures.—The authors used geographic object-based image analysis (GEOBIA) by means of Trimble eCognition software to map forests, cangas (natural metalliferous savanna ecosystems), mine land, revegetated areas, and water bodies at open-cast iron ore mines. GEOBIA is a method commonly applied to classify pixels into homogeneous clusters that aid with identifying objects in images. It is an alternative software to LandTrendr, which works explicitly with Landsat data. They identified 35 ground-control points per class to inform classification thresholds.
Results.—The author’s methods using GEOBIA by means of Trimble eCognition software to map land cover, mine lands, and water bodies achieved an overall accuracy of greater than 90 percent and kappa indices ranging from 0.82 to 0.88. By combining high-resolution satellite imagery (spectral and lidar) with modeling approaches, the authors were able to map land cover classes and temporal transitions on mine sites.
Case Study 7—Hyperspectral at a Single Site
Title.—Analysis and discrimination of hyperspectral characteristics of typical vegetation leaves in a rare earth reclamation mining area (Zhou and others, 2022)
Subject.—Change detection on a solution mine to extract REEs in subtropical forests in Dingnan County, Jiangxi Province, China.
Platforms, sensors, or both.—This study used an analytical spectral devices (ASD) Field Spec4 spectrometer to record the reflectance of vegetation.
Preprocessing.—The original spectral data was log-transformed and underwent derivative and continuum removal.
Performance indicators, vegetation indicators, or both.—The authors aimed to assess environmental stress of vegetation caused by contamination and how well the reclamation succeeded by evaluating spectral signatures of six species, including wetland pine, eucalyptus, Taiwanese photinia, bamboo willow, camellia, and tungoil tree.
Modeling procedures.—The authors used reflectance of vegetation at a mining site for selected species and three discrimination models (Fisher, stepwise discrimination, and multilayer perception) to determine vegetation types based on the electromagnetic spectrum.
Results.—The results demonstrated that stepwise discrimination with data transformation was the best method for identifying reclaimed vegetation at this site. Additionally, the results provide a theoretical basis for physiological parameters for reclaimed REE mining and monitoring efforts and add to the spectral library of reclaimed vegetation.
Case Study 8—Radar Only at a Single Site
Title.—Rapid assessment of mine rehabilitation areas with airborne lidar and deep learning—Bauxite strip mining in Queensland, Australia (Murray and others, 2022)
Subject.—Land cover classification and reference site characterization of bauxite mining (aluminum) operations in tropical ecosystems of Cape York Peninsula, Queensland, Australia.
Platforms, sensors, or both.—Airborne lidar sensor.
Preprocessing.—Lidar data were delivered as a collection of classified lidar (.LAS) datasets in 1 square-kilometer (km2) tiles. The authors used Esri ArcGIS Pro to process the raw lidar data, which included formatting merged tiles (“.LAS” file format for point clouds) into a scene layer package file that was then colorized by point class (for example, ground, low vegetation, medium vegetation, high vegetation, buildings and structures).
Performance indicators, vegetation indicators, or both.—The authors used lidar point clouds and an intensity image (ratio of the strength of reflected light to that of emitted light) to evaluate vegetation structure on mined compared to “natural” sites.
Modeling procedures.—A convolutional neural network algorithm was trained to classify reference sites (natural ecosystems). The study used airborne lidar data to train a support vector machine (SVM) and a neural network model to identify active rehabilitation sites and natural ecosystems. The result of the neural network is a binary category of whether or not there was active rehabilitation. The SVM generated a predictive surface of rehabilitation status (zero for bare ground and one for natural landscape).
Results.—The authors evaluated the SVM and model results using ground-truthed data, an ordinary least squares regression, and an expert panel of scientists. They found high predictive performance of their methods. Estimates of vegetation structure and canopy height were the most important indices to identify rehabilitated land. Lidar can assess vegetation structural attributes, such as canopy height and basal area; moreover, lidar data used in machine learning can produce additional attributes, such as stem direction and trunk thickness, and density can be derived. The study highlights the importance of remote sensing for monitoring mines, using a quasi-automated approach, and importantly suggests that rehabilitation of mine sites requires continuous monitoring and assessment.
References Cited
Daly, C., Halbleib, M., Smith, J.I., Gibson, W.P., Doggett, M.K., Taylor, G.H., Curtis, J., and Pasteris, P.P., 2008, Physiographically sensitive mapping of climatological temperature and precipitation across the conterminous United States: International Journal of Climatology, v. 28, no. 15, p. 2031–2064, accessed August 10, 2022, at https://doi.org/10.1002/joc.1688.
Jamali, S., Jönsson, P., Eklundh, L., Ardö, J.S., 2015, Detecting changes in vegetation trends using time series segmentation: Remote Sensing of Environment, v. 156, no. 0034-4257, p. 182–195, accessed August 10, 2022, at https://doi.org/10.1016/j.rse.2014.09.010.
Murray, X., Apan, A., Deo, R., and Maraseni, T., 2022, Rapid assessment of mine rehabilitation areas with airborne lidar and deep learning—Bauxite strip mining in Queensland, Australia: Geocarto International, v. 37, no. 26, p. 11223–11252, accessed August 10, 2022, at https://doi.org/10.1080/10106049.2022.2048902.
Nascimento, F.S., Gastauer, M., Souza-Filho, P.W.M., Nascimento, W.R., Santos, D.C., and Costa, M.F., 2020, Land cover changes in open-cast mining complexes based on high-resolution remote sensing data: Remote Sensing, v. 12, no. 4, 21 p., accessed August 10, 2022, at https://doi.org/10.3390/rs12040611.
Ross, M.R.V., Nippgen, F., McGlynn, B.L., Thomas, C.J., Brooks, A.C., Shriver, R.K., Moore, E.M., and Bernhardt, E.S., 2021, Mountaintop mining legacies constrain ecological, hydrological and biogeochemical recovery trajectories: Environmental Research Letters, v. 16, no. 7, 13 p., accessed August 10, 2022, at https://doi.org/10.1088/1748-9326/ac09ac.
Thomas, C.J., Shriver, R.K., Nippgen, F., Hepler, M., and Ross, M.R.V., 2023, Mines to forests? Analyzing long-term recovery trends for surface coal mines in Central Appalachia: Restoration Ecology, v. 31, no. 5, 12 p., accessed August 10, 2022, at https://doi.org/10.1111/rec.13827.
Thomas, C., Shriver, R.K., Nippgen, F., and Ross, M.R.V., 2022, SkyTruth—Surface mining in Central Appalachia—Site recovery data (ver. 2, November 2022): figshare dataset, accessed August 10, 2022, at https://doi.org/10.6084/m9.figshare.19623768.v2.
Xie, L., Wu, W., Huang, X., Ou, P., Lin, Z., Zhiling, W., Song, Y., Lang, T., Huangfu, W., Zhang, Y., Zhou, X., Fu, X., Li, J., Jiang, J., Zhang, M., Zhang, Z., Qin, Y., Peng, S., Shao, C., and Bai, Y., 2020, Mining and restoration monitoring of rare earth element (REE) exploitation by new remote sensing indicators in Southern Jiangxi, China: Remote Sensing, v. 12, no. 21, 19 p., accessed August 10, 2022, at https://doi.org/10.3390/rs12213558.
Xulu, S., Phungula, P.T., Mbatha, N., and Moyo, I., 2021, Multi-year mapping of disturbance and reclamation patterns over Tronox’s Hillendale mine, South Africa with DBEST and Google Earth Engine: Land, v. 10, no. 7, 17 p., accessed August 10, 2022, at https://doi.org/10.3390/land10070760.
Yuan, W., Wang, Q., Fan, J., and Li, H., 2017, Mining land subsidence monitoring using Sentinel-1 SAR data: The International Archives of the Photogrammetry, Remote Sensing and Spatial Information Sciences, v. XLII-2/W7, p. 655–658, accessed August 10, 2022, at https://doi.org/10.5194/isprs-archives-XLII-2-W7-655-2017.
Zhou, B., Li, H., and Xu, F., 2022, Analysis and discrimination of hyperspectral characteristics of typical vegetation leaves in a rare earth reclamation mining area: Ecological Engineering, v. 174, 11 p., accessed August 10, 2022, at https://doi.org/10.1016/j.ecoleng.2021.106465.
Bibliography
Ahumada-Mexía, R., Murillo-Jiménez, J.M., Ortega-Rubio, A., Marmolejo-Rodríguez, A.J., and Nava-Sánchez, E.H., 2021, Identification of mining waste using remote sensing technique—A case study in El Triunfo town, BCS, México: Remote Sensing Applications—Society and Environment, v. 22, 9 p., accessed August 10, 2022, at https://doi.org/10.1016/j.rsase.2021.100493.
Ammirati, L., Chirico, R., Di Martire, D., and Mondillo, N., 2022, Application of multispectral remote sensing for mapping flood-affected zones in the Brumadinho Mining District (Minas Gerais, Brasil): Remote Sensing, v. 14, no. 6, 12 p., accessed August 10, 2022, at https://doi.org/10.3390/rs14061501.
Banerjee, B.P., and Raval, S., 2022, Mapping sensitive vegetation communities in mining eco-space using UAV-lidar: International Journal of Coal Science & Technology, v. 9, 16 p., accessed August 10, 2022, at https://doi.org/10.1007/s40789-022-00509-w.
Bao, N., Li, W., Gu, X., and Liu, Y., 2019, Biomass estimation for semiarid vegetation and mine rehabilitation using Worldview-3 and Sentinel-1 SAR imagery: Remote Sensing, v. 11, no. 23, 20 p., accessed August 10, 2022, at https://doi.org/10.3390/rs11232855.
Bodlák, L., Krováková, K., Nedbal, V., and Pechar, L., 2012, Assessment of landscape functionality changes as one aspect of reclamation quality—The case of Velká podkrušnohorská dump, Czech Republic: Ecological Engineering, v. 43, p. 19–25, accessed August 10, 2022, at https://doi.org/10.1016/j.ecoleng.2011.11.018.
Boerchers, M., Fitzpatrick, P., Storie, C., and Hostetler, G., 2016, Reinvention through regreening–Examining environmental change in Sudbury, Ontario: The Extractive Industries and Society, v. 3, no. 3, p. 793–801, accessed August 10, 2022, at https://doi.org/10.1016/j.exis.2016.03.005.
Buzzi, M.A., Rueter, B.L., and Ghermandi, L., 2017, Múltiples índices espectrales para predecir la variabilidad de atributos estructurales y funcionales en zonas áridas: Ecología Austral, v. 27, no. 1, p. 55–62, accessed August 10, 2022, at https://doi.org/10.25260/EA.17.27.1.0.315.
Carabassa, V., Montero, P., Crespo, M., Padro, J.C., Pons, X., Balague, J., Brotons, L., and Alcaniz, J.M., 2020, Unmanned aerial system protocol for quarry restoration and mineral extraction monitoring: Journal of Environmental Management, v. 270, 11 p., accessed August 10, 2022, at https://doi.org/10.1016/j.jenvman.2020.110717.
Carol, E.S., Braga, F., Kruse, E.E., and Tosi, L., 2014, A retrospective assessment of the hydrological conditions of the Samborombón coastland (Argentina): Ecological Engineering, v. 67, p. 223–237, accessed August 10, 2022, at https://doi.org/10.1016/j.ecoleng.2014.03.081.
Chen, W., Li, W., Yang, Z., and Wang, Q., 2021, Analysis of mining-induced variation of the water table and potential benefits for ecological vegetation—A case study of Jinjitan coal mine in Yushenfu mining area, China: Hydrogeology Journal, v. 29, no. 4, p. 1629–1645, accessed August 10, 2022, at https://doi.org/10.1007/s10040-021-02325-z.
Corrêa, R.S., Balduíno, A.P.d.C., Teza, C.T.V., and Baptista, G.M.d.M., 2018, Vegetation cover development resulting from different restoration approaches of exploited mines: Floresta e Ambiente, v. 25, no. 4, 9 p., accessed August 10, 2022, at https://doi.org/10.1590/2179-8087.111617.
De Simoni, B.S., and Leite, M.G.P., 2019, Assessment of rehabilitation projects results of a gold mine area using landscape function analysis: Applied Geography, v. 108, p. 22–29, accessed August 10, 2022, at https://doi.org/10.1016/j.apgeog.2019.05.005.
Erener, A., 2011, Remote sensing of vegetation health for reclaimed areas of Seyitömer open cast coal mine: International Journal of Coal Geology, v. 86, no. 1, p. 20–26, accessed August 10, 2022, at https://doi.org/10.1016/j.coal.2010.12.009.
Erskine, P.D., Bartolo, R., McKenna, P., and Humphrey, C., 2019, Using reference sites to guide ecological engineering and restoration of an internationally significant uranium mine in the Northern Territory, Australia: Ecological Engineering, v. 129, p. 61–70, accessed August 10, 2022, at https://doi.org/10.1016/j.ecoleng.2019.01.008.
Erthalia, M., Supriatna, and Damayanti, A., 2019, Remote sensing and GIS for land conservation—Identification of post-tin mining land conservation in Perimping Sub Watershed: IOP Conference Series—Earth and Environmental Science, v. 311, 7 p., accessed August 10, 2022, at https://doi.org/10.1088/1755-1315/311/1/012019.
Fan, X., Song, Y., Zhu, C., Balzter, H., and Bai, Z., 2021, Estimating ecological responses to climatic variability on reclaimed and unmined lands using enhanced vegetation index: Remote Sensing, v. 13, no. 6, 20 p., accessed August 10, 2022, at https://doi.org/10.3390/rs13061100.
Gastauer, M., Nascimento, W.R., Caldeira, C.F., Ramos, S.J., Souza-Filho, P.W.M., and Féret, J.-B., 2022, Spectral diversity allows remote detection of the rehabilitation status in an Amazonian iron mining complex: International Journal of Applied Earth Observation and Geoinformation, v. 106, 11 p., accessed August 10, 2022, at https://doi.org/10.1016/j.jag.2021.102653.
Gong, B., Shu, C., Han, S., and Cheng, S.G., 2021, Mine vegetation identification via ecological monitoring and deep belief network: Plants (Basel), v. 10, no. 6, 22 p., accessed August 10, 2022, at https://doi.org/10.3390/plants10061099.
Götze, C., Beyer, F., and Gläßer, C., 2016, Pioneer vegetation as an indicator of the geochemical parameters in abandoned mine sites using hyperspectral airborne data: Environmental Earth Sciences, v. 75, 14 p., accessed August 10, 2022, at https://doi.org/10.1007/s12665-016-5367-1.
Grösel, K., and Belocky, R., 2003, Mining site environmental assessment and re-vegetation planning in an alpine environment utilizing advanced remote sensing techniques—EARSeL Workshop on Imaging Spectroscopy, 3d, Herrsching, Germany, May 13–16, 2003, Proceedings: European Association of Remote Sensing Laboratories, p. 313–323, accessed August 10, 2022, at https://citeseerx.ist.psu.edu/document?repid=rep1&type=pdf&doi=972869f15ed5f30e15d0c4b8daff19905d5c92aa.
Guan, Y., Wang, J., Zhou, W., Bai, Z., and Cao, Y., 2022, Identification of land reclamation stages based on succession characteristics of rehabilitated vegetation in the Pingshuo opencast coal mine: Journal of Environmental Management, v. 305, 14 p., accessed August 10, 2022, at https://doi.org/10.1016/j.jenvman.2021.114352.
Han, Y., Ke, Y., Zhu, L., Feng, H., Zhang, Q., Sun, Z., and Zhu, L., 2021, Tracking vegetation degradation and recovery in multiple mining areas in Beijing, China, based on time-series Landsat imagery: GIScience & Remote Sensing, v. 58, no. 8, p. 1477–1496, accessed August 10, 2022, at https://doi.org/10.1080/15481603.2021.1996319.
Hao, H., Lian, Z., Zhao, J., Wang, H., and He, Z., 2022, A remote-sensing ecological index approach for restoration assessment of rare-earth elements mining: Computational Intelligence and Neuroscience, v. 2022, 14 p., accessed August 10, 2022, at https://doi.org/10.1155/2022/5335419.
Hao, L., Zhang, Z., and Yang, X., 2019, Mine tailing extraction indexes and model using remote-sensing images in southeast Hubei Province: Environmental Earth Sciences, v. 78, no. 15, 11 p., accessed August 10, 2022, at https://doi.org/10.1007/s12665-019-8439-1.
Hawkes, V.C., Miller, M.T., Novoa, J., Ibeke, E., and Martin, J.P., 2020, Opportunistic wetland formation, characterization, and quantification on landforms reclaimed to upland ecosites in the Athabasca Oil Sands region: Wetlands Ecology and Management, v. 28, no. 6, p. 953–970, accessed August 10, 2022, at https://doi.org/10.1007/s11273-020-09760-x.
He, J., Chen, D., Zhan, Y., Liu, C., and Liu, R., 2022, Vegetation changes in Alberta Oil Sands, Canada, based on remotely sensed data from 1995 to 2020: Journal of Environmental & Earth Sciences, v. 4, no. 2, p. 17–32, accessed August 10, 2022, at https://doi.org/10.30564/jees.v4i2.4687.
Hengkai, L., Feng, X., and Qin, L., 2020, Remote sensing monitoring of land damage and restoration in rare earth mining areas in 6 counties in southern Jiangxi based on multisource sequential images: Journal of Environmental Management, v. 267, 9 p., accessed August 10, 2022, at https://doi.org/10.1016/j.jenvman.2020.110653.
Hu, J., Ye, B., Bai, Z., and Hui, J., 2022, Comparison of the vegetation index of reclamation mining areas calculated by multi-source remote sensing data: Land, v. 11, no. 3, 16 p., accessed August 10, 2022, at https://doi.org/10.3390/land11030325.
Hu, X., and Li, X., 2019, Information extraction of subsided cultivated land in high-groundwater-level coal mines based on unmanned aerial vehicle visible bands: Environmental Earth Sciences, v. 78, 11 p., accessed August 10, 2022, at https://doi.org/10.1007/s12665-019-8417-7.
Hui, J., Bai, Z., Ye, B., and Wang, Z., 2021, Remote sensing monitoring and evaluation of vegetation restoration in grassland mining areas—A case study of the Shengli mining area in Xilinhot City, China: Land, v. 10, no. 7, 18 p., accessed August 10, 2022, at https://doi.org/10.3390/land10070743.
Jiang, Y., Liu, S., Liu, M., Peng, X., Liao, X., Wang, Z., Gao, H., Disney, M., and Zhang, J., 2022, A systematic framework for continuous monitoring of land use and vegetation dynamics in multiple heterogeneous mine sites: Remote Sensing in Ecology and Conservation, v. 8, no. 6, p. 793–807, accessed August 10, 2022, at https://doi.org/10.1002/rse2.276.
Karan, S.K., Samadder, S.R., and Maiti, S.K., 2016, Assessment of the capability of remote sensing and GIS techniques for monitoring reclamation success in coal mine degraded lands: Journal of Environmental Management, v. 182, p. 272–283, accessed August 10, 2022, at https://doi.org/10.1016/j.jenvman.2016.07.070.
Kun, M., 2019, Assessment and monitoring of rehabilitation studies on coal mine dump site with UAV’s: Applied Ecology and Environmental Research, v. 17, no. 4, 13 p., accessed August 10, 2022, at https://doi.org/10.15666/aeer/1704_73817393.
Kuzevic, S., Bobikova, D., and Kuzevicova, Z., 2022, Land cover and vegetation coverage changes in the mining area—A case study from Slovakia: Sustainability, v. 14, no. 3, 14 p., accessed August 10, 2022, at https://doi.org/10.3390/su14031180.
LeClerc, E., and Wiersma, Y.F., 2017, Assessing post-industrial land cover change at the Pine Point Mine, NWT, Canada using multi-temporal Landsat analysis and landscape metrics: Environmental Monitoring and Assessment, v. 189, 19 p., accessed August 10, 2022, at https://doi.org/10.1007/s10661-017-5893-7.
Li, S., Wang, J., Zhang, M., and Tang, Q., 2021, Characterizing and attributing the vegetation coverage changes in North Shanxi coal base of China from 1987 to 2020: Resources Policy, v. 74, 12 p., accessed August 10, 2022, at https://doi.org/10.1016/j.resourpol.2021.102331.
Li, J., Yan, X., Cao, Z., Yang, Z., Liang, J., Ma, T., and Liu, Q., 2020, Identification of successional trajectory over 30 years and evaluation of reclamation effect in coal waste dumps of surface coal mine: Journal of Cleaner Production, v. 269, 13 p., accessed August 10, 2022, at https://doi.org/10.1016/j.jclepro.2020.122161.
Li, H., Zhou, B., and Xu, F., 2022, Variation analysis of spectral characteristics of reclamation vegetation in a rare earth mining area under environmental stress: IEEE Transactions on Geoscience and Remote Sensing, v. 60, p. 1–12, accessed August 10, 2022, at https://doi.org/10.1109/TGRS.2022.3141579.
Ma, B., Yang, X., Yu, Y., Shu, Y., and Che, D., 2021, Investigation of vegetation changes in different mining areas in Liaoning Province, China, using multisource remote sensing data: Remote Sensing, v. 13, no. 24, 14 p., accessed August 10, 2022, at https://doi.org/10.3390/rs13245168.
Malaviya, S., Munsi, M., Oinam, G., and Joshi, P.K., 2010, Landscape approach for quantifying land use land cover change (1972–2006) and habitat diversity in a mining area in Central India (Bokaro, Jharkhand): Environmental Monitoring and Assessment, v. 170, no. 1-4, p. 215–229, accessed August 10, 2022, at https://doi.org/10.1007/s10661-009-1227-8.
Matejicek, L., and Kopackova, V., 2010, Changes in croplands as a result of large scale mining and the associated impact on food security studied using time-series Landsat images: Remote Sensing, v. 2, no. 6, p. 1463–1480, accessed August 10, 2022, at https://doi.org/10.3390/rs2061463.
Meyer, V., Saatchi, S., Ferraz, A., Xu, L., Duque, A., Garcia, M., and Chave, J., 2019, Forest degradation and biomass loss along the Choco region of Colombia: Carbon Balance and Management, v. 14, 15 p., accessed August 10, 2022, at https://doi.org/10.1186/s13021-019-0117-9.
Nie, X., Hu, Z., Zhu, Q., and Ruan, M., 2021, Research on temporal and spatial resolution and the driving forces of ecological environment quality in coal mining areas considering topographic correction: Remote Sensing, v. 13, no. 14, 22 p., accessed August 10, 2022, at https://doi.org/10.3390/rs13142815.
Padmanaban, R., Bhowmik, A., and Cabral, P., 2017, A remote sensing approach to environmental monitoring in a reclaimed mine area: ISPRS International Journal of Geo-Information, v. 6, no. 12, 14 p., accessed August 10, 2022, at https://doi.org/10.3390/ijgi6120401.
Padró, J.C., Carabassa, V., Balagué, J., Brotons, L., Alcañiz, J.M., and Pons, X., 2019, Monitoring opencast mine restorations using Unmanned Aerial System (UAS) imagery: Science of the Total Environment, v. 657, p. 1602–1614, accessed August 10, 2022, at https://doi.org/10.1016/j.scitotenv.2018.12.156.
Pan, J., Li, H., and Li, Y., 2022, Spatiotemporal change analysis of environmental quality in mining areas based on long-term Landsat images: Geocarto International, v. 37, no. 26, p. 11052–11067, accessed August 10, 2022, at https://doi.org/10.1080/10106049.2022.2046862.
Peng, Y., Kheir, R.B., Adhikari, K., Malinowski, R., Greve, M.B., Knadel, M., and Greve, M.H., 2016, Digital mapping of toxic metals in Qatari soils using remote sensing and ancillary data: Remote Sensing, v. 8, no. 12, 19 p., accessed August 10, 2022, at https://doi.org/10.3390/rs8121003.
Petropoulos, G.P., Partsinevelos, P., and Mitraka, Z., 2013, Change detection of surface mining activity and reclamation based on a machine learning approach of multi-temporal Landsat TM imagery: Geocarto International, v. 28, no. 4, p. 323–342, accessed August 10, 2022, at https://doi.org/10.1080/10106049.2012.706648.
Raval, S., Sarver, E., Shamsoddini, A., Zipper, C., Donovan, P., Evans, D., and Chu, H.T., 2014, Satellite remote sensing-based estimates of biomass production on reclaimed coal mines: Mining Engineering, v. 66, no. 4, p. 76–82, accessed August 10, 2022, at https://www.scopus.com/inward/record.uri?partnerID=HzOxMe3b&scp=84897513927&origin=inward.
Ren, H., Xiao, W., Zhao, Y., and Hu, Z., 2020, Land damage assessment using maize aboveground biomass estimated from unmanned aerial vehicle in high groundwater level regions affected by underground coal mining: Environmental Science and Pollution Research, v. 27, p. 21666–21679, accessed August 10, 2022, at https://doi.org/10.1007/s11356-020-08695-3.
Ren, H., Zhao, Y., Xiao, W., Zhang, J., Chen, C., Ding, B., and Yang, X., 2022, Vegetation growth status as an early warning indicator for the spontaneous combustion disaster of coal waste dump after reclamation—An unmanned aerial vehicle remote sensing approach: Journal of Environmental Management, v. 317, 17 p., accessed August 10, 2022, at https://doi.org/10.1016/j.jenvman.2022.115502.
Shao, H., Sun, X., Lin, Y., Xian, W., Zhou, Y., Yuan, L., and Qi, J., 2021, A method for spatio-temporal process assessment of eco-geological environmental security in mining areas using catastrophe theory and projection pursuit model: Progress in Physical Geography: Earth and Environment, v. 45, no. 5, p. 647–668, accessed August 10, 2022, at https://doi.org/10.1177/0309133320982542.
Vishwakarma, A.K., Mishra, V.N., Rai, R., and Shrivastva, B.K., 2021, Quantitative assessment of the effect of mining subsidence on the health of native floras using remote sensing techniques: Results in Geophysical Sciences, v. 8, 13 p., accessed August 10, 2022, at https://doi.org/10.1016/j.ringps.2021.100031.
Wang, S., Huang, J., Yu, H., and Ji, C., 2020, Recognition of landscape key areas in a coal mine area of a semi-arid steppe in China—A case study of Yimin Open-Pit Coal Mine: Sustainability, v. 12, no. 6, 22 p., accessed August 10, 2022, at https://doi.org/10.3390/su12062239.
Wang, J., Jiao, Z., and Bai, Z., 2014, Changes in carbon sink value based on RS and GIS in the Heidaigou opencast coal mine: Environmental Earth Sciences, v. 71, p. 863–871, accessed August 10, 2022, at https://doi.org/10.1007/s12665-013-2488-7.
Wang, W., Liu, R., Gan, F., Zhou, P., Zhang, X., and Ding, L., 2021, Monitoring and evaluating restoration vegetation status in mine region using remote sensing data—Case study in Inner Mongolia, China: Remote Sensing, v. 13, no. 7, 23 p., accessed August 10, 2022, at https://doi.org/10.3390/rs13071350.
Wang, H., and Ma, M., 2016, Impacts of climate change and anthropogenic activities on the ecological restoration of wetlands in the arid regions of China: Energies, v. 9, no. 3, 25 p., accessed August 10, 2022, at https://doi.org/10.3390/en9030166.
Wohlfart, C., Mack, B., Liu, G., and Kuenzer, C., 2017, Multi-faceted land cover and land use change analyses in the Yellow River Basin based on dense Landsat time series—Exemplary analysis in mining, agriculture, forest, and urban areas: Applied Geography, v. 85, p. 73–88, accessed August 10, 2022, at https://doi.org/10.1016/j.apgeog.2017.06.004.
Wu, Z., Li, H., and Wang, Y., 2021, Mapping annual land disturbance and reclamation in rare-earth mining disturbance region using temporal trajectory segmentation: Environmental Science and Pollution Research, v. 28, p. 69112–69128, accessed August 10, 2022, at https://doi.org/10.1007/s11356-021-15480-3.
Xu, F., Li, H., and Li, Y., 2021, Ecological environment quality evaluation and evolution analysis of a rare earth mining area under different disturbance conditions: Environmental Geochemistry and Health, v. 43, p. 2243–2256, accessed August 10, 2022, at https://doi.org/10.1007/s10653-020-00761-6.
Yang, Y., Erskine, P.D., Lechner, A.M., Mulligan, D., Zhang, S., and Wang, Z., 2018, Detecting the dynamics of vegetation disturbance and recovery in surface mining area via Landsat imagery and LandTrendr algorithm: Journal of Cleaner Production, v. 178, p. 353–362, accessed August 10, 2022, at https://doi.org/10.1016/j.jclepro.2018.01.050.
Yang, Y., Erskine, P.D., Zhang, S., Wang, Y., Bian, Z., and Lei, S., 2018, Effects of underground mining on vegetation and environmental patterns in a semi-arid watershed with implications for resilience management: Environmental Earth Sciences, v. 77, 12 p., accessed August 10, 2022, at https://doi.org/10.1007/s12665-018-7796-5.
Yang, Z., Li, J., Zipper, C.E., Shen, Y., Miao, H., and Donovan, P.F., 2018, Identification of the disturbance and trajectory types in mining areas using multitemporal remote sensing images: Science of the Total Environment, v. 644, p. 916–927, accessed August 10, 2022, at https://doi.org/10.1016/j.scitotenv.2018.06.341.
Yang, Y., Ren, X., Zhang, S., Chen, F., and Hou, H., 2017, Incorporating ecological vulnerability assessment into rehabilitation planning for a post-mining area: Environmental Earth Sciences, v. 76, 16 p., accessed August 10, 2022, at https://doi.org/10.1007/s12665-017-6568-y.
Yang, Y., Tang, J., Zhang, Y., Zhang, S., Zhou, Y., Hou, H., and Liu, R., 2022, Reforestation improves vegetation coverage and biomass, but not spatial structure, on semi-arid mine dumps: Ecological Engineering, v. 175, 9 p., accessed August 10, 2022, at https://doi.org/10.1016/j.ecoleng.2021.106508.
Yang, Y., Zhang, Y., Su, X., Hou, H., and Zhang, S., 2021, The spatial distribution and expansion of subsided wetlands induced by underground coal mining in eastern China: Environmental Earth Sciences, v. 80, 14 p., accessed August 10, 2022, at https://doi.org/10.1007/s12665-021-09422-y.
Yuan, D., Hu, Z., Yang, K., Guo, J., Li, P., Li, G., and Fu, Y., 2021, Assessment of the ecological impacts of coal mining and restoration in alpine areas—A case study of the Muli Coalfield on the Qinghai-Tibet Plateau: IEEE Access, v. 9, 16 p., accessed August 10, 2022, at https://doi.org/10.1109/ACCESS.2021.3133478.
Zenkov, I.V., Morin, A.S., Vokin, V.N., and Kiryushina, E.V., 2020, Justification of disturbed land reclamation after open pit mining of kimberlite pipes in the Republic of Sakha (Yakutia): Eurasian Mining, v. 2020, no. 1, p. 65–69, accessed August 10, 2022, at https://doi.org/10.17580/em.2020.01.13.
Zenkov, I.V., Nefedov, B.N., Zayats, V.V., and Kiryushina, E.V., 2018, Remote monitoring of mining situation and disturbed land ecology at the Teisk and Abagas iron ore deposits: Eurasian Mining, v. 1, p. 41–44, accessed August 10, 2022, at https://doi.org/10.17580/em.2018.01.09.
Zenkov, I.V., Zayats, V.V., Nefedov, B.N., and Nefedov, N.B., 2017, Earth remote sensing in ecological evaluation of disturbed lands in South Yakutia: Eurasian Mining, v. 2, p. 49–52, accessed August 10, 2022, at https://doi.org/10.17580/em.2017.02.12.
Zhang, L., Bai, K.Z., Wang, M.J., and Karthikeyan, R., 2016, Basin-scale spatial soil erosion variability—Pingshuo opencast mine site in Shanxi Province, Loess Plateau of China: Natural Hazards, v. 80, p. 1213–1230, accessed August 10, 2022, at https://doi.org/10.1007/s11069-015-2019-9.
Zhang, X., Liu, Y., Chen, X., Long, L., Su, Y., Yu, X., Zhang, H., Chen, Y., and An, S., 2022, Analysis of spatial and temporal changes of vegetation cover and its driving forces in the Huainan mining area: Environmental Science and Pollution Research, v. 29, p. 60117–60132, accessed August 10, 2022, at https://doi.org/10.1007/s11356-022-19921-5.
Zhang, Y., Shen, W., Li, M., and Lv, Y., 2020, Integrating Landsat time series observations and corona images to characterize forest change patterns in a mining region of Nanjing, Eastern China from 1967 to 2019: Remote Sensing, v. 12, no. 19, 21 p., accessed August 10, 2022, at https://doi.org/10.3390/rs12193191.
Zhang, M., Wang, J., and Li, S., 2019, Tempo-spatial changes and main anthropogenic influence factors of vegetation fractional coverage in a large-scale opencast coal mine area from 1992 to 2015: Journal of Cleaner Production, v. 232, p. 940–952, accessed August 10, 2022, at https://doi.org/10.1016/j.jclepro.2019.05.334.
Zhang, M., Zhou, W., and Li, Y., 2017, The analysis of object-based change detection in mining area—A case study with Pingshuo Coal Mine: The International Archives of the Photogrammetry, Remote Sensing and Spatial Information Sciences, v. XLII-2/W7, p. 1017–1023, accessed August 10, 2022, at https://doi.org/10.5194/isprs-archives-XLII-2-W7-1017-2017.
Zhao, Y., Zheng, W., Xiao, W., Zhang, S., Lv, X., and Zhang, J., 2020, Rapid monitoring of reclaimed farmland effects in coal mining subsidence area using a multi-spectral UAV platform: Environmental Monitoring and Assessment, v. 192, art. 474, 19 p., accessed August 10, 2022, at https://doi.org/10.1007/s10661-020-08453-5.
Appendix 2. Selected References and Remote Sensing Tools and Data for Monitoring Mine Lands
Regulatory Documentation
Adams, M.B., 2017, The forestry reclamation approach—Guide to successful reforestation of mined lands: U.S. Department of Agriculture Forest Service General Technical Report NRS-169, 119 p. [Also available at https://doi.org/10.2737/nrs-gtr-169.]
Bureau of Land Management, 2007, Abandoned mine land program policy handbook: Bureau of Land Management, Document H-3720-1, 93 p. [Also available at https://www.blm.gov/sites/default/files/docs/2022-05/Rel.%203-331.pdf.]
U.S. Environmental Protection Agency, 2000, Abandoned mine site characterization and cleanup handbook: U.S. Environmental Protection Agency, Region 8, Document EPA 910-B-00-001, 129 p. [Also available at https://www.epa.gov/sites/default/files/2015-09/documents/2000_08_pdfs_amscch.pdf.]
U.S. Environmental Protection Agency, 2020, Best practices to prevent releases from impoundments at abandoned mine sites while conducting CERCLA response actions: U.S. Environmental Protection Agency, Office of Land and Emergency Management, Directive No. 9285.2-14, 64 p. [Also available at https://semspub.epa.gov/work/HQ/100002586.pdf.]
Mining Data Inventories
Burghardt, J.E., Norby, E.S., and Pranger, H.S., II, 2014, Abandoned mineral lands in the National Park System—Comprehensive inventory and assessment: Fort Collins, Colo., National Park Service, Geologic Resources Division, Natural Resource Technical Report NPS/NRSS/GRD/NRTR—2014/906, 60 p. [Also available at https://irma.nps.gov/DataStore/Reference/Profile/2215804.]
Horton, J.D., and San Juan, C.A., 2016, Prospect- and mine-related features from U.S. Geological Survey 7.5- and 15-minute topographic quadrangle maps of the United States (ver. 10.0, May 2023): U.S. Geological Survey data release. [Also available at https://doi.org/10.5066/F78W3CHG.]
U.S. Department of the Interior, Office of Surface Mining Reclamation and Enforcement, 2022, Abandoned mine land inventory system: Office of Surface Mining Reclamation and Enforcement database, accessed December 15, 2022, at https://amlis.osmre.gov/.
U.S. Geological Survey, 2022, Mineral commodity summaries 2022: U.S. Geological Survey, 202 p., accessed December 16, 2022, at https://doi.org/10.3133/mcs2022. [All annual reports also available at https://www.usgs.gov/centers/national-minerals-information-center/mineral-commodity-summaries.]
U.S. Geological Survey, 2022, National minerals information center: U.S. Geological Survey website, accessed December 16, 2022, at https://www.usgs.gov/centers/national-minerals-information-center.
U.S. Geological Survey, 2022, Explore search—Mine sites: U.S. Geological Survey website, accessed December 16, 2022, at https://www.usgs.gov/search?keywords=mine%20sites.
U.S. Government Accountability Office, 2020, Abandoned hardrock mines— Information on number of mines, expenditures, and factors that limit efforts to address hazards: Washington, D.C., U.S. Government Accountability Office, GAO-20-238, 56 p. [Also available at https://www.gao.gov/products/gao-20-238.]
Literature Reviews that Evaluate Mine Land Recovery Using Remote Sensing and Remote Sensing Vegetation Indices
Feng, Y., Wang, J., Bai, Z., and Reading, L., 2019, Effects of surface coal mining and land reclamation on soil properties—A review: Earth-Science Reviews, v. 191, p. 12–25. [Also available at https://doi.org/10.1016/j.earscirev.2019.02.015.]
Gastauer, M., Silva, J.R., Caldeira, C.F., Jr., Ramos, S.J., Souza Filho, P.W.M., Furtini Neto, A.E., and Siqueira, J.O., 2018, Mine land rehabilitation—Modern ecological approaches for more sustainable mining: Journal of Cleaner Production, v. 172, p. 1409–1422. [Also available at https://doi.org/10.1016/j.jclepro.2017.10.223.]
Gibbons, P., and Freudenberger, D., 2006, An overview of methods used to assess vegetation condition at the scale of the site: Ecological Management & Restoration, v. 7, no. S1, p. S10–S17. [Also available at https://doi.org/10.1111/j.1442-8903.2006.00286.x.]
McKenna, P.B., Lechner, A.M., Phinn, S., and Erskine, P.D., 2020, Remote sensing of mine site rehabilitation for ecological outcomes—A global systematic review: Remote Sensing, v. 12, no. 21, 34 p. [Also available at https://doi.org/10.3390/rs12213535.]
Song, W., Song, W., Gu, H., and Li, F., 2020, Progress in the remote sensing monitoring of the ecological environment in mining areas: International Journal of Environmental Research and Public Health, v. 17, no. 6, 17 p. [Also available at https://doi.org/10.3390/ijerph17061846.]
Xie, Y., Sha, Z., and Yu, M., 2008, Remote sensing imagery in vegetation mapping—A review: Journal of Plant Ecology, v. 1, no. 1, p. 9–23. [Also available at https://doi.org/10.1093/jpe/rtm005.]
Xue, J., and Su, B., 2017, Significant remote sensing vegetation indices—A review of developments and applications: Journal of Sensors, v. 2017, 17 p. [Also available at https://doi.org/10.1155/2017/1353691.]
Guidelines for Ecological Restoration
Gann, G.D., McDonald, T., Walder, B., Aronson, J., Nelson, C.R., Jonson, J., Hallett, J.G., Eisenberg, C., Guariguata, M.R., Liu, J., Hua, F., Echeverría, C., Gonzales, E., Shaw, N., Decleer, K., and Dixon, K.W., 2019, International principles and standards for the practice of ecological restoration. Second edition: Restoration Ecology, v. 27, no. S1, p. S1–S46. [Also available at https://doi.org/10.1111/rec.13035.]
Gibbons, P., and Freudenberger, D., 2006, An overview of methods used to assess vegetation condition at the scale of the site: Ecological Management & Restoration, v. 7, no. S1, p. S10–S17. [Also available at https://doi.org/10.1111/j.1442-8903.2006.00286.x.]
Ruiz-Jaen, M.C., and Aide, T.M., 2005, Restoration success—How is it being measured?: Restoration Ecology, v. 13, no. 3, p. 569–577. [Also available at https://doi.org/10.1111/j.1526-100X.2005.00072.x.]
Pilot Studies on Mine Land Restoration
Bureau of Land Management and Forest Service, 2007, Abandoned mine lands—A decade of progress reclaiming hardrock mines: Bureau of Land Management and U.S. Department of Agriculture Forest Service, 39 p. [ Also available at https://www.blm.gov/sites/blm.gov/files/uploads/AML_PUB_DecadeProgress.pdf.]
Finger, S.E., Church, S.E., and von Guerard, P., 2007, Potential for successful ecological remediation, restoration, and monitoring, chap. F of Church, S.E., von Guerard, P., and Finger, S.E., eds., 2007, Integrated investigations of environmental effects of historical mining in the Animas River watershed, San Juan County, Colorado: U.S. Geological Survey Professional Paper 1651, 1,096 p., plus CD-ROM. [Also available at https://pubs.usgs.gov/pp/1651/.]
Krzyszowska Waitkus, A., 2022, Sustainable reclamation practices for a large surface coal mine in shortgrass prairie, semiarid environment (Wyoming, USA)—Case study: International Journal of Coal Science & Technology, v. 9, 14 p. [Also available at https://doi.org/10.1007/s40789-022-00502-3.]
Mining Effects on Abiotic and Biotic Conditions:
Feng, Y., Wang, J., Bai, Z., and Reading, L., 2019, Effects of surface coal mining and land reclamation on soil properties—A review: Earth-Science Reviews, v. 191, p. 12–25. [Also available at https://doi.org/10.1016/j.earscirev.2019.02.015.]
Sonter, L.J., Ali, S.H., and Watson, J.E.M., 2018, Mining and biodiversity—Key issues and research needs in conservation science: Proceedings of the Royal Society B—Biological Sciences, v. 285, no. 1892, 9 p. [Also available at https://doi.org/10.1098/rspb.2018.1926.]
Thomas, C.J., Shriver, R.K., Nippgen, F., Hepler, M., and Ross, M.R.V., 2023, Mines to forests? Analyzing long‐term recovery trends for surface coal mines in Central Appalachia: Restoration Ecology, v. 31, no. 5, 12 p. [Also available at https://doi.org/10.1111/rec.13827.]
Ugya, Y.A., Ajibade, T.F., and Ajibade, F.O., 2018, Water pollution resulting from mining activity—An overview, in The 2018 Annual Conference of the School of Engineering & Engineering Technology (SEET) 2018, [Proceedings]: Akure, Nigeria, The Federal University of Technology (FUTA), p. 703–718. [Also available at https://www.academia.edu/37213206/Water_Pollution_Resulting_from_Mining_Activity_An_Overview.]
Sources of Publicly Available Remotely Sensed Data
National Aeronautics and Space Administration (NASA) Earth Observations (NEO) website.—Provides access to more than 50 different global datasets represented with daily, weekly, and monthly snapshots, and images that are available in a variety of formats, including joint photographic experts group (JPEG), portable network graphics (PNG), Google Earth, and geographic tag image file format (GeoTIFF; https://neo.gsfc.nasa.gov/about/).
National Oceanic and Atmospheric Administration (NOAA).—Provides access to Geostationary Operational Environmental Satellites (GEOS)-R Series and NOAA-20 data for tracking weather. GOES-R data are refreshed approximately every 15 minutes (such geostationary satellites have high orbits and low resolution of 250 meters and above).
Sentinel Hub.—Provides access to all Sentinel products, including historical (archive) satellite images. Sentinel Hub also gives users access to Landsat and Moderate Resolution Imaging Spectroradiometer (MODIS) products (https://www.sentinel-hub.com/).
U.S. Geological Survey EarthExplorer.—Provides access to aerial photographs, Landsat, Advanced Very High Resolution Radiometer, commercial satellites (Satellite Pour l'Observation de la Terre [SPOT], IKONOS, and OrbView 3, some Sentinel-2B), declassified data, elevation (Shuttle Radar Topography Mission and Advanced Spaceborne Thermal Emission and Reflection Radiometer), MODIS, hyperspectral (Hyperion), digital line graphs, Landsat, and other data (https://earthexplorer.usgs.gov/).
Publicly Available Browsing Tools with Access to Remote Sensing Data
NASA Worldview.—An open platform for browsing recently updated and historical satellite remotely sensed data. The site allows users to visually track wildfires, night lights, floodwaters, icebergs, and earthquakes (https://worldview.earthdata.nasa.gov/).
Zoom Earth.—Shows the most recent satellite images and aerial views in a fast, zoomable map. The platform pulls in refreshed data every 10 minutes from NOAA’s Geostationary Operational Environmental Satellite Network [GOES] and Japan Meteorological Agency Himawari-8 satellites, and every 15 minutes by means of European Organisation for the Exploitation of Meteorological Satellites (https://zoom.earth/).
Global Imagery Browse Services.—Provides quick access to more than 1,000 satellite imagery products, covering every part of the world. Most imagery is updated daily and is available within a few hours after satellite observation, and some products span almost 30 years. The satellite imagery can be rendered in your web client or geographic information system (GIS) application (https://www.earthdata.nasa.gov/eosdis/science-system-description/eosdis-components/gibs).
EarthNow.—Displays near real-time imagery of Landsat 8 and Landsat 9 data being collected by the U.S. Geological Survey as the satellites cross over the Earth. Along with live stream video, the tool replays image loops from recent overpass recordings (https://earthnow.usgs.gov/observer/).
Google Earth Timelapse.—Provides timelapses of the Earth and how it has changed over the past 37 years (https://earthengine.google.com/timelapse/).
Cloud Computing Tools with Access to Remote Sensing Data
Earth on Amazon Web Services (AWS).—Available datasets (open data) currently include Sentinel-2, Landsat-8, GEOS, NOAA, Sentinel-1, and data acquired by the China-Brazil Earth Resources Satellite and processed by Instituto Nacional de Pesquisa Espaciais. There is a cost for using AWS cloud for processing, but AWS offers Cloud Credits to students, researchers, and developers (https://aws.amazon.com/earth/).
Google Earth.—Provides browsing capabilities for some of the highest-resolution satellite imagery. Most of the data on Google Earth was taken in the last 3–4 years (https://earth.google.com/).
Google Earth Engine.—This tool combines a multipetabyte catalog of satellite imagery and geospatial datasets with planetary-scale analysis capabilities (https://earthengine.google.com/).
Data Available from Google Earth Engine
Surface temperature.—MODIS, Advanced Spaceborne Thermal Emission and Reflection Radiometer, Advanced Very High Resolution Radiometer, and Landsat thermal data.
Climate.—North American Land Data Assimilation System, Gridded Surface Meteorological, climate model outputs like the University of Idaho Multivariate Adaptive Constructed Analogs (MACAv2-METDATA), and the NASA Earth Exchange’s Downscaled Climate Projections.
Atmosphere.—NASA's Total Ozone Mapping Spectrometer and Ozone Monitoring Instrument, and the MODIS Monthly Gridded Atmospheric Product.
Weather.—NOAA's Global Forecast System and the National Centers for Environmental Prediction Climate Forecast System.
Sensor data.—For example, the Tropical Rainfall Measuring Mission, Landsat since 1972, Sentinel (includes all-weather radar images from Sentinel-1A and -1B, high-resolution optical images from Sentinel-2A and -2B, and ocean and land data suitable for environmental and climate monitoring from Sentinel-3), and MODIS (NASA's Terra and Aqua satellites have been acquiring images of the Earth daily since 1999, including daily imagery, 16-day bidirectional reflectance distribution function-adjusted surface reflectance, and derived products such as vegetation indices and snow cover).
Aerial imagery.—U.S. National Agriculture Imagery Program offers aerial image data of the United States at 1-meter resolution, including nearly complete coverage every several years since 2003.
Elevation.—Shuttle Radar Topography Mission data at 30-meter resolution, regional Digital Elevation Models at higher resolutions, and derived products such as the World Wildlife Fund’s HydroSHEDS hydrology database.
Landcover.—A variety of land cover datasets, from near real-time Dynamic World to global products such as European Space Agency World Cover.
Cropland.—U.S. Department of Agriculture, National Agricultural Statistical Service Cropland Data Layers, and layers from the Global Food Security-Support Analysis Data including cropland extent, crop dominance, and watering sources.
Nighttime data.—Nighttime imagery from the Defense Meteorological Satellite Program's Operational Linescan System, which has collected imagery of nighttime lights at approximately 1-kilometer resolution continuously since 1992.
Conversion Factors
International System of Units to U.S. customary units
Abbreviations
AWS
Amazon Web Services
DBEST
Detecting Breakpoints and Estimating Segments in Trend
DInSAR
Differential Interferometry Synthetic Aperture Radar
EVI
Enhanced Vegetation Index
GDVI
Generalized Difference Vegetation Index
GEE
Google Earth Engine
GEOBIA
Geographic Object-Based Image Analysis
GeoTIFF
Geographic Tag Image File Format
GIS
geographic information system
JPEG
Joint Photographic Experts Group
LANDFIRE
Landscape Fire and Resource Management Planning Tools
LandTrendr
Landsat-based Detection of Trends in Disturbance and Recovery
LEDAPS
Landsat Ecosystem Disturbance Adaptive Processing System
lidar
Light Detection and Ranging
MODIS
Moderate Resolution Imaging Spectroradiometer
MRAI
mining and restoration assessment indicators
NASA
National Aeronautics and Space Administration
NDVI
Normalized Difference Vegetation Index
REE
rare earth element
PNG
Portable Network Graphics
SAR
Synthetic Aperture Radar
SAVI
Soil Adjusted Vegetation Index
SPOT
Satellite Pour l'Observation de la Terre
SMCRA
Surface Mining Control and Reclamation Act
SVM
support vector machine
VI
vegetation index
Publishing support provided by the Science Publishing Network, Denver, Moffett Field, and Reston Publishing Service Centers
For more information concerning the research in this report, contact the
Director, USGS Fort Collins Science Center
2150 Centre Ave., Bldg. C
Fort Collins, CO 80526-8118
(970) 226-9100
Or visit the Fort Collins Science Center website at:
Disclaimers
Any use of trade, firm, or product names is for descriptive purposes only and does not imply endorsement by the U.S. Government.
Although this information product, for the most part, is in the public domain, it also may contain copyrighted materials as noted in the text. Permission to reproduce copyrighted items must be secured from the copyright owner.
Suggested Citation
O’Donnell, M.S., Whipple, A.L., Inman, R.D., Tarbox, B.C., Monroe, A.P., Robb, B.S., and Aldridge, C.L., 2024, Remote sensing for monitoring mine lands and recovery efforts: U.S. Geological Survey Circular 1525, 34 p., https://doi.org/10.3133/cir1525.
ISSN: 2330-5703 (online)
Study Area
Publication type | Report |
---|---|
Publication Subtype | USGS Numbered Series |
Title | Remote sensing for monitoring mine lands and recovery efforts |
Series title | Circular |
Series number | 1525 |
DOI | 10.3133/cir1525 |
Year Published | 2024 |
Language | English |
Publisher | U.S. Geological Survey |
Publisher location | Reston VA |
Contributing office(s) | Fort Collins Science Center |
Description | v, 34 p. |
Country | United States |
Other Geospatial | continental United States |
Online Only (Y/N) | Y |
Google Analytic Metrics | Metrics page |